F(Bgd): Learning Embeddings From Positive Unlabeled Data With Bgd
UNCERTAINTY IN ARTIFICIAL INTELLIGENCE(2018)
摘要
Learning sparse features from only positive and unlabeled (PU) data is a fundamental task for problems of several domains, such as natural language processing (NLP), computer vision (CV), information retrieval (IR). Considering the numerous amount of unlabeled data, most prevalent methods rely on negative sampling (NS) to increase computational efficiency. However, sampling a fraction of unlabeled data as negative for training may ignore other important examples, and thus lead to non-optimal prediction performance. To address this, we present a fast and generic batch gradient descent optimizer (f(BGD)) to learn from all training examples without sampling. By leveraging sparsity in PU data, we accelerate f(BGD) by several magnitudes, making its time complexity the same level as the NS-based stochastic gradient descent method. Meanwhile, we observe that the standard batch gradient method suffers from gradient instability issues due to the sparsity property. Driven by a theoretical analysis for this potential cause, an intuitive solution arises naturally. To verify its efficacy, we perform experiments on multiple tasks with PU data across domains, and show that f(BGD) consistently outperforms NS-based models on all tasks with comparable efficiency.
更多查看译文
AI 理解论文
溯源树
样例
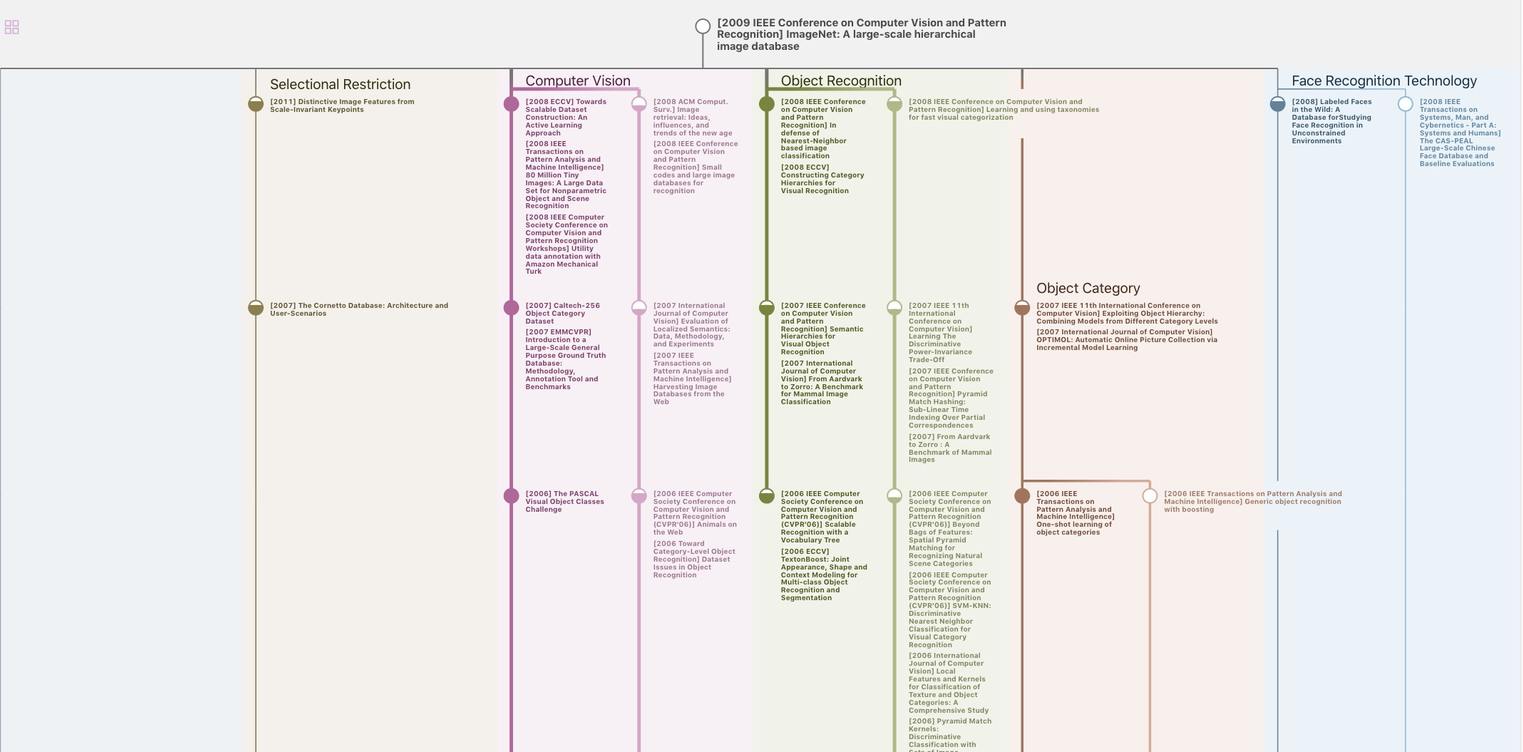
生成溯源树,研究论文发展脉络
Chat Paper
正在生成论文摘要