Image Denoising with Deep Convolutional and Multi-directional LSTM Networks under Poisson Noise Environments
2018 18th International Symposium on Communications and Information Technologies (ISCIT)(2018)
摘要
Image denoising especially in low-light conditions is a very challenging task because the noise characteristics of different noise sources contributed in varying proportions at different signal levels are difficult to capture. Inaccurate modeling of the noise often results in producing undesirable artifacts in the restored images. This paper presents a new image denoising method in Poisson noise called Deep Convolutional and Multidirectional Long-short Term Memory Networks (DCSLNet). Deep Convolutional Neural Network (CNN) is firstly used to extract features and estimate noise components of the images. Multi-directional Long-short Term Memory (LSTM) network is then introduced in the second stage to effectively capture the long-range correlations of the noise in the deeper CNN layers. The proposed DCSLNet is trainable end-to-end to restore the clean image from the input noisy image. Experimental results in both subjective and objective qualities show that the proposed DCSLNet is very competitive in denoising low-light images under heavy-noise conditions compared with the other state-of-the-art Poisson image denoising methods.
更多查看译文
关键词
Image denoising,Poisson noise,Deep Convolutional Neural Network (CNN),Multi-directional Long-short Term Memory (LSTM)
AI 理解论文
溯源树
样例
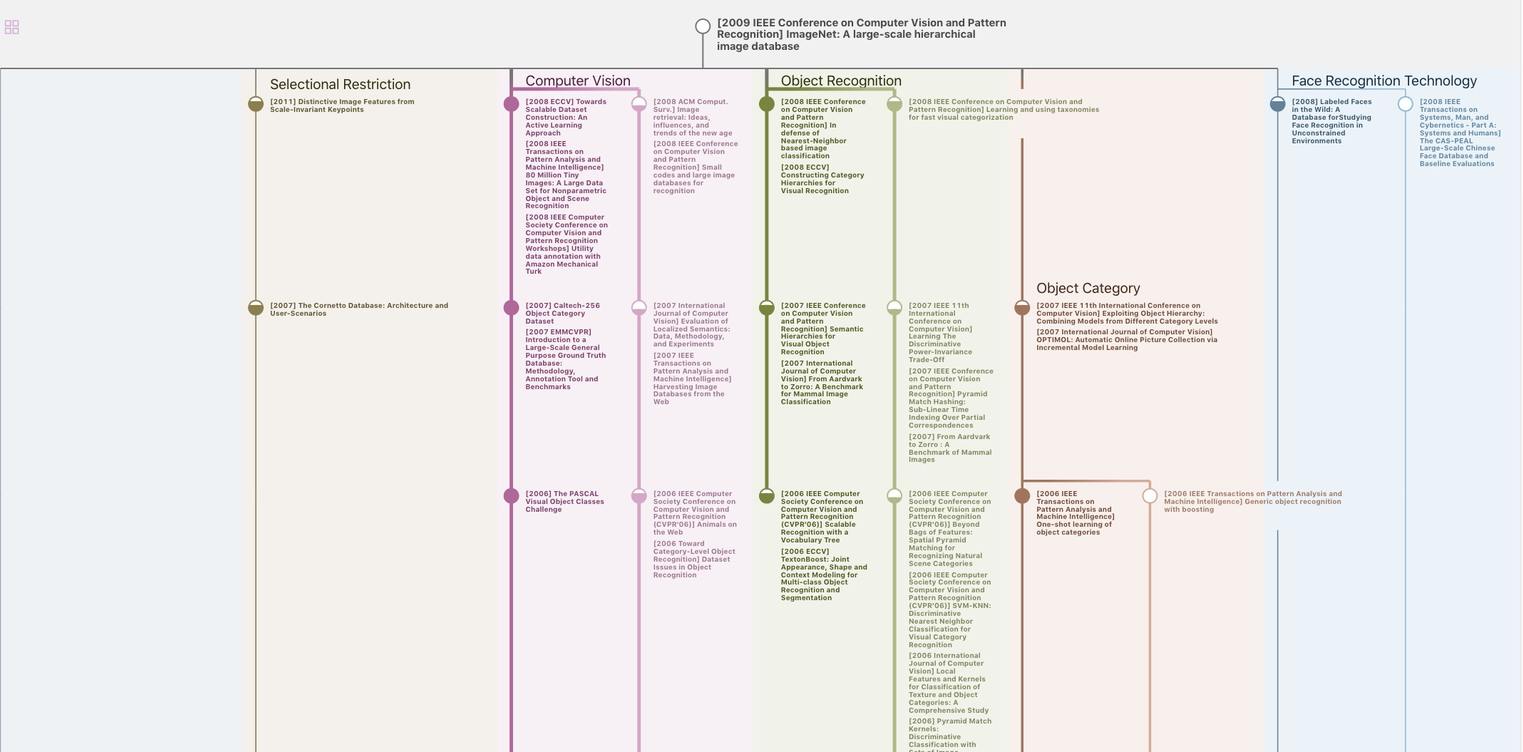
生成溯源树,研究论文发展脉络
Chat Paper
正在生成论文摘要