ELECTOR: Evaluator for long reads correction methods
bioRxiv(2019)
摘要
Motivation: In the last few years, third generation sequencing error rates have been capped above 5%, including many insertions and deletions. Thereby, an increasing number of long reads correction methods have been proposed to reduce the noise in these sequences. Whether hybrid or self-correction methods, there exist multiple approaches to correct long reads data. As the quality of the error correction has huge impacts on downstream processes, developing methods allowing to evaluate error correction tools with precise and reliable statistics is therefore a crucial need. Since error correction is often a resource bottleneck in long reads pipelines. A key feature of assessment methods is therefore to be efficient, in order to allow the fast comparison of different tools. Results: We propose ELECTOR, a reliable and efficient tool to evaluate long reads correction, that enables the evaluation of hybrid and self-correction methods. Our tool provides a complete and relevant set of metrics to assess the read quality improvement after correction and scales to large datasets. ELECTOR is directly compatible with a wide range of state-of-the-art error correction tools, using whether simulated or real long reads. We show that ELECTOR displays a wider range of metrics than the state-of-the-art tool, LRCstats, and additionally importantly decreases the runtime needed for assessment on most datasets.
更多查看译文
AI 理解论文
溯源树
样例
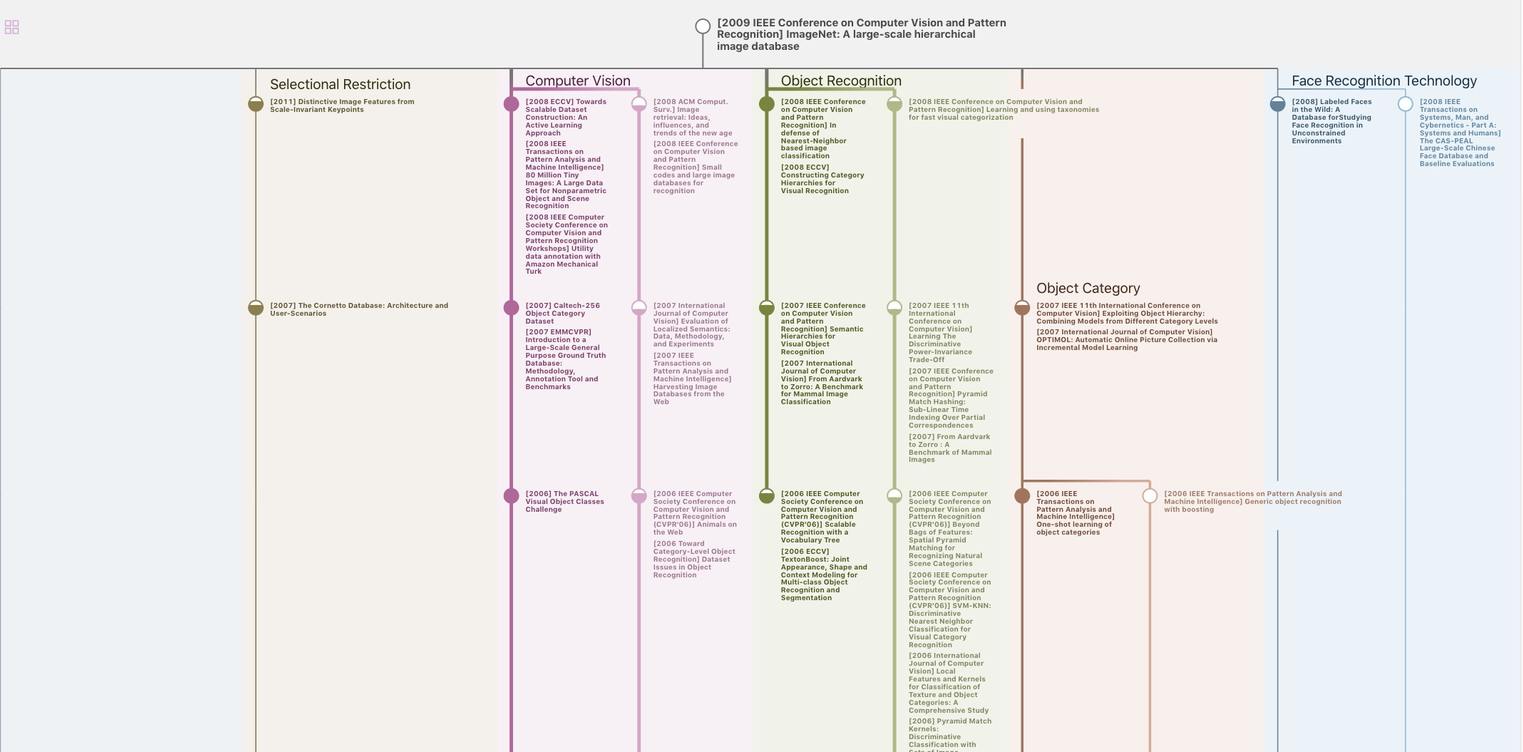
生成溯源树,研究论文发展脉络
Chat Paper
正在生成论文摘要