Convergence of a Relaxed Variable Splitting Method for Learning Sparse Neural Networks via $$\ell _1, \ell _0$$, and Transformed-$$\ell _1$$ Penalties
arXiv: Optimization and Control(2020)
摘要
Sparsification of neural networks is one of the effective complexity reduction methods to improve efficiency and generalizability. We consider the problem of learning a one hidden layer convolutional neural network with ReLU activation function via gradient descent under sparsity promoting penalties. It is known that when the input data is Gaussian distributed, no-overlap networks (without penalties) in regression problems with ground truth can be learned in polynomial time at high probability. We propose a relaxed variable splitting method integrating thresholding and gradient descent to overcome the non-smoothness in the loss function. The sparsity in network weight is realized during the optimization (training) process. We prove that under \(\ell _1, \ell _0,\) and transformed-\(\ell _1\) penalties, no-overlap networks can be learned with high probability, and the iterative weights converge to a global limit which is a transformation of the true weight under a novel thresholding operation. Numerical experiments confirm theoretical findings, and compare the accuracy and sparsity trade-off among the penalties.
更多查看译文
关键词
Regularization,Sparsification,Non-convex optimization.
AI 理解论文
溯源树
样例
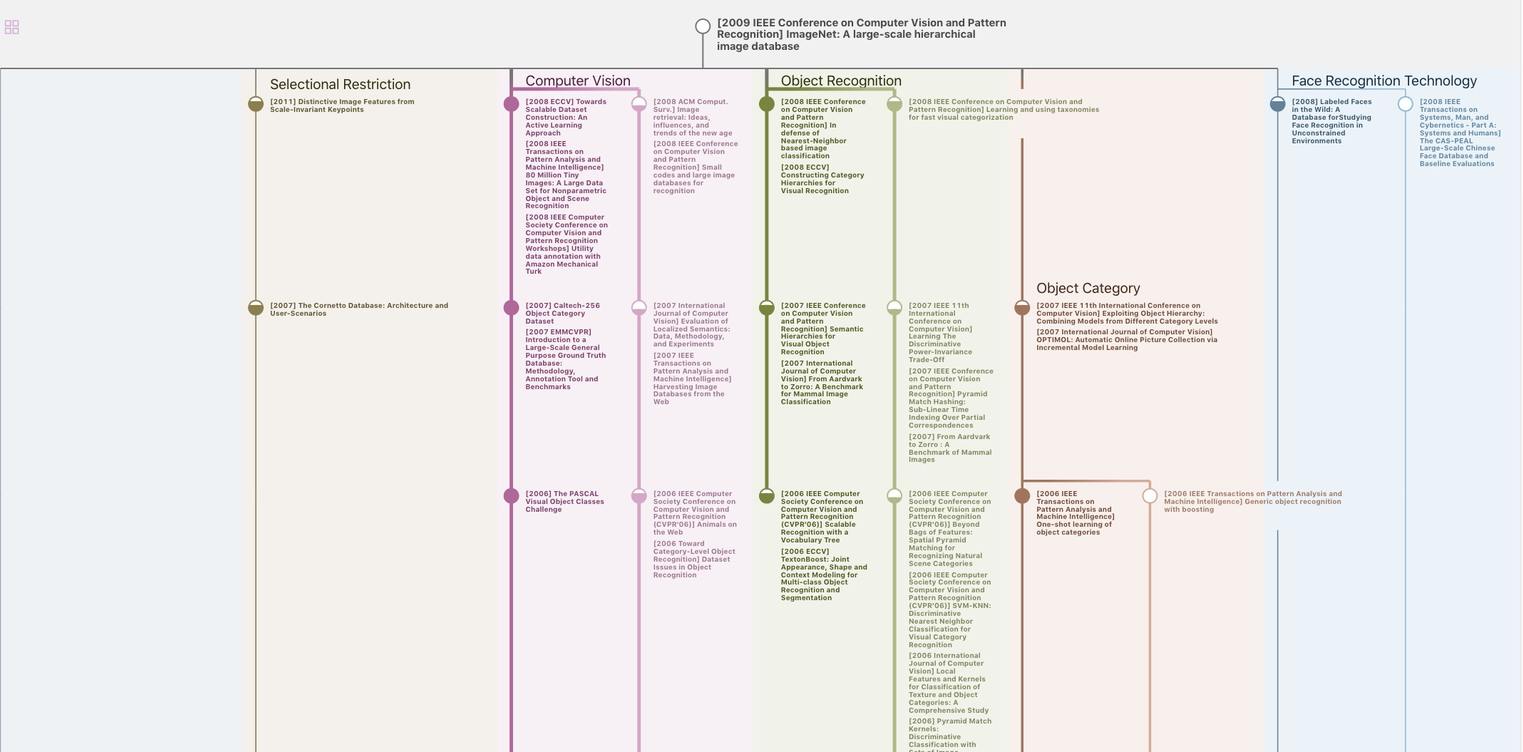
生成溯源树,研究论文发展脉络
Chat Paper
正在生成论文摘要