Data-driven online learning and reachability analysis of stochastic hybrid systems for smart buildings
Cyber-Physical Systems(2019)
摘要
ABSTRACTAnalysis and design of cyber physical systems (CPS) relies typically on detailed dynamical models. Identifying parametric models of complex CPS such as smart buildings is very hard because of the heterogeneity and complexity of components as well as uncertainty and variability. Alternatively, data availability can potentially support the use of machine learning techniques to develop nonparametric models, which can be used for prediction, analysis, and control. In this paper, we present a data-driven methodology to learn a nonparametric stochastic hybrid system from the observed data in an online fashion. The model uses Gaussian processes and periodic Markov chains to represent the coupled continuous and discrete dynamics respectively. Moreover, we propose a reachability analysis algorithm that represents the reachable states for a receding finite horizon using mixtures of Gaussian processes. The reachability analysis algorithm provides an efficient multi-step prediction for SHS, which can be used ...
更多查看译文
关键词
stochastic hybrid systems,reachability analysis,online learning,data-driven
AI 理解论文
溯源树
样例
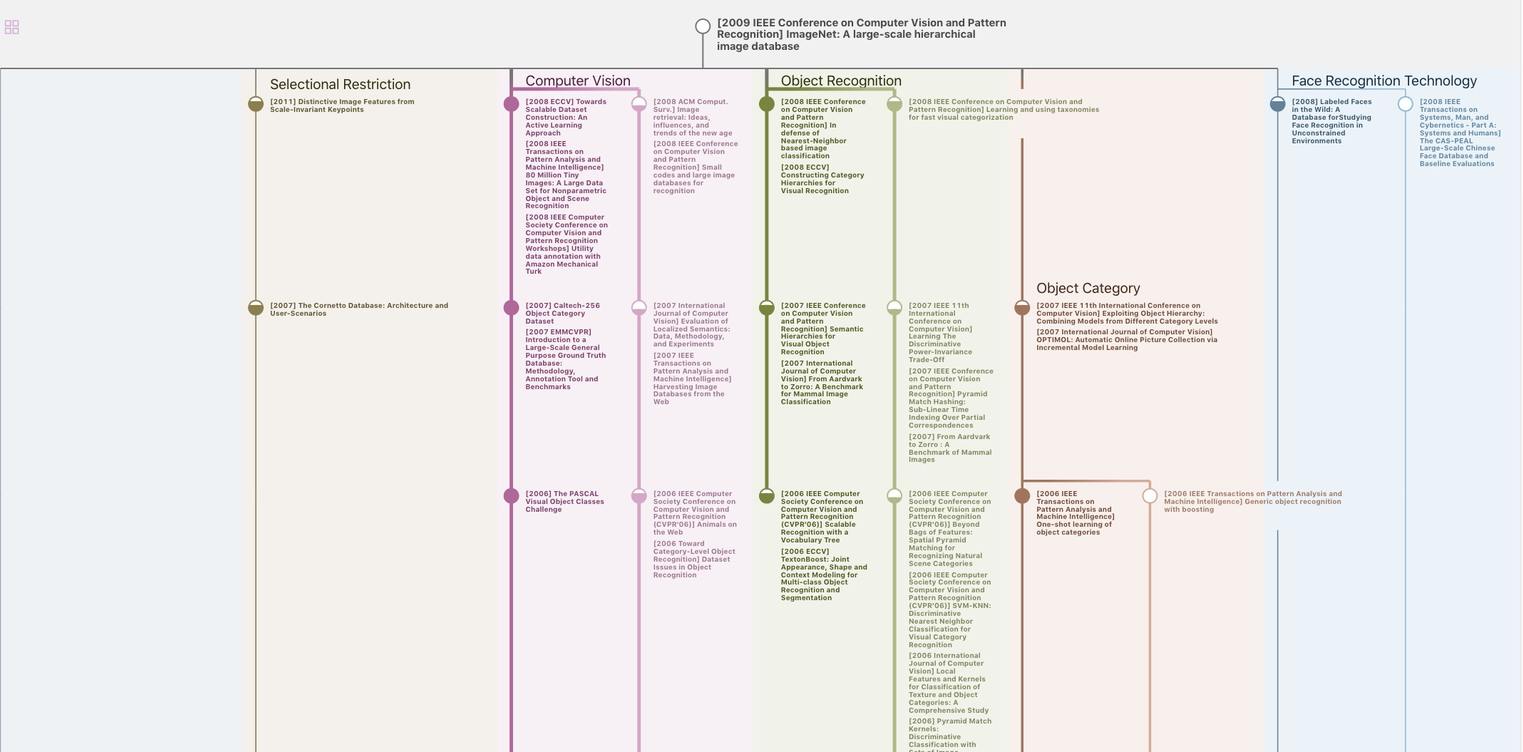
生成溯源树,研究论文发展脉络
Chat Paper
正在生成论文摘要