Discrete Hashing Based Supervised Matrix Factorization for Cross-Modal Retrieval
international conference on artificial intelligence(2018)
摘要
Cross-modal hashing is a method which projects heterogeneous multimedia data into a common low-dimensional latent space. Many methods based on hash codes try to keep the relationship between text and corresponding image, and relax the original discrete learning problem into a continuous learning problem. However, these methods may produce ineffective hash codes since they do not make full use of the relationship between different modalities and simply relax the discrete binary constraint into a continuous problem. Collective matrix factorization (CMF) has achieved impressive results in mining semantic concepts or latent topics from image/text. In this paper, we propose a new supervised learning framework which unifies CMF method that maximizes the correlation between two modalities and discrete cyclic coordinate descent (DCC) method that solves NP-hard problems, which ensures that the hash codes generated in the cross-modal are more accurate and efficient. Experiments on three benchmark data sets show the effectiveness of the proposed method.
更多查看译文
AI 理解论文
溯源树
样例
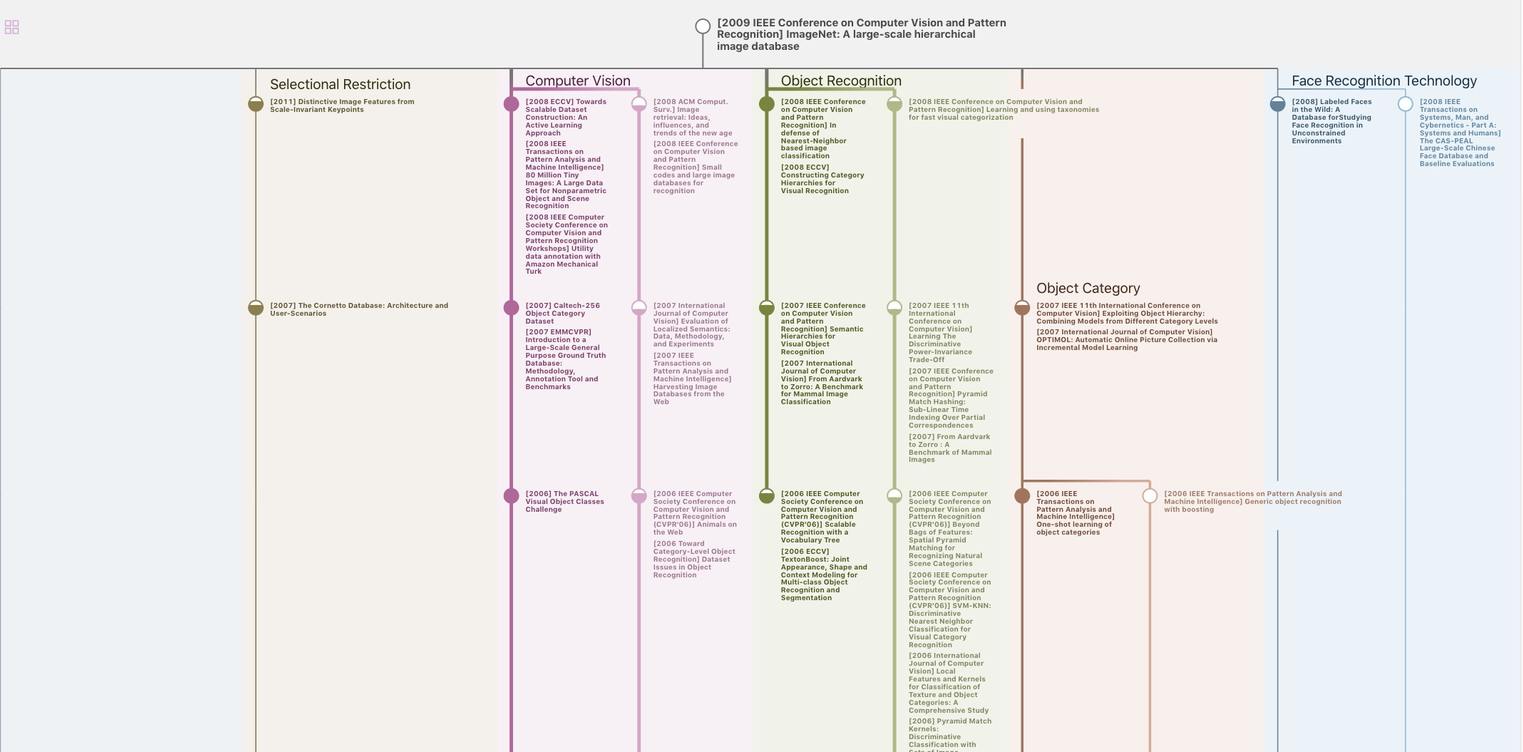
生成溯源树,研究论文发展脉络
Chat Paper
正在生成论文摘要