Lossy Image Compression with Filter Bank Based Convolutional Networks
2019 Data Compression Conference (DCC)(2019)
摘要
Filter bank based convolutional networks (FBCNs) enable efficient separable multiscale and multidirectional decomposition with a convolutional cascade of 1-D radial and directional filter banks. In this paper, we propose a two-stage subband coding framework for FBCN analysis coefficients using a SPIHT-like algorithm and subsequent primitive-based adaptive arithmetic coding (AAC). The SPIHT-like algorithm extends spatial orientation tree to exploit inter-subband dependency between subbands of different scales and directions. Mutual information is estimated for information-theoretical measurement to formulate such dependencies. Various primitives are designed adaptively encode the generated bitstream by fitting its varying lists and passes. Neural networks are leveraged to improve probability estimation for AAC, where nonlinear prediction is made based on contexts regarding scales, directions, locations and significance of analysis coefficients. Experimental results show that the proposed framework improves the lossy coding performance for FBCN analysis coefficients in comparison to the state-of-the-arts subband coding schemes SPIHT.
更多查看译文
关键词
Filter Bank,Convolutional Network,Image Compression
AI 理解论文
溯源树
样例
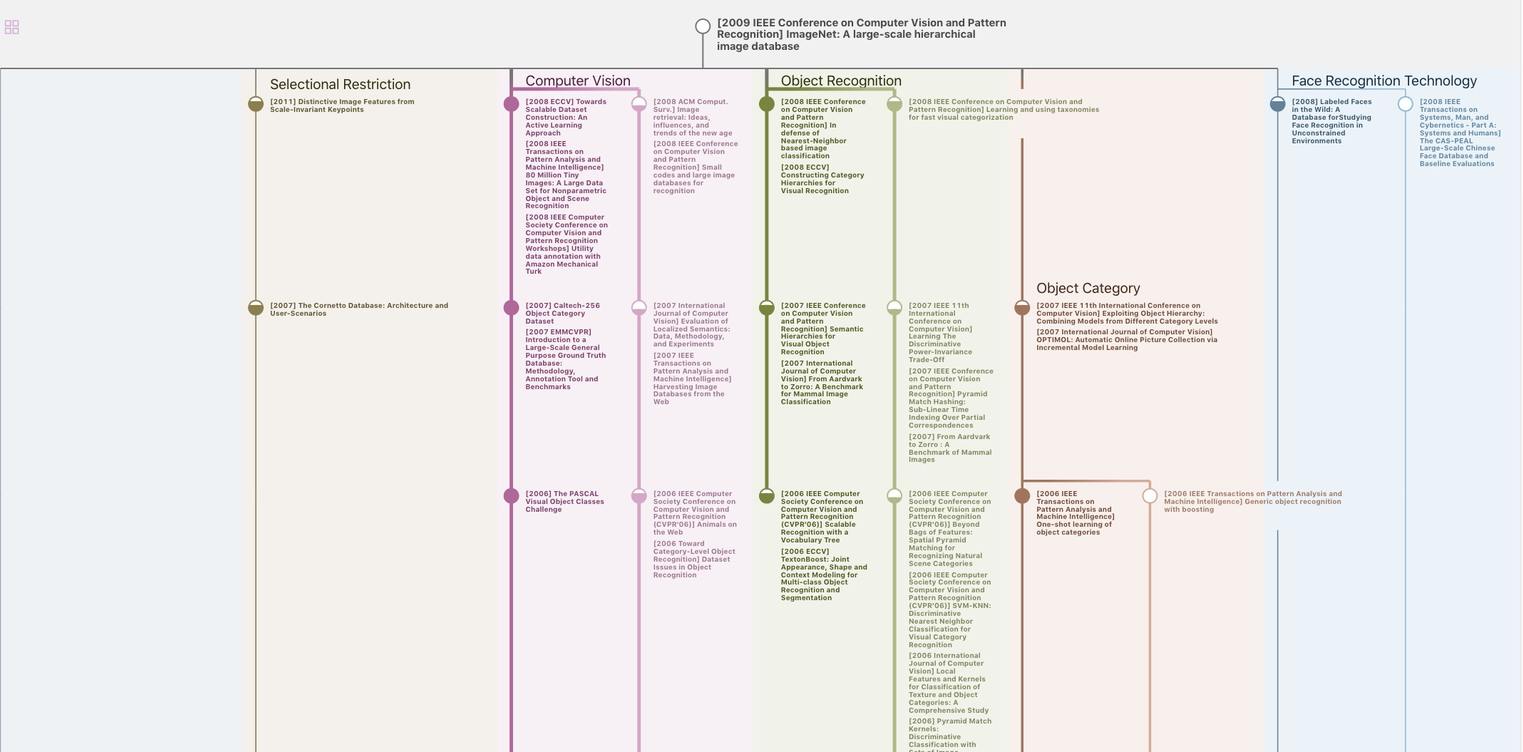
生成溯源树,研究论文发展脉络
Chat Paper
正在生成论文摘要