Modeling Temporal Variation in Physical Activity Using Functional Principal Components Analysis
Statistics in Biosciences(2019)
摘要
Accelerometers are person-worn sensors that provide objective measurements of movement based on minute-level activity counts, thus providing a rich framework for assessing physical activity patterns. New statistical approaches and computational tools are needed to exploit these densely sampled time-series data. We implement a functional principal component mixed model approach to ascertain temporal activity patterns in 578 overweight women (60% cancer survivors) and summarize individual patterns with unique personalized principal component scores. We then test if these patterns are associated with health by performing multiple regression of health outcomes (including biomarkers, namely, insulin, C-reactive protein, and quality of life) on activity patterns represented by these scores. Our model elucidates the most important patterns/modes of variation in physical activities. Results show that health outcomes including biomarkers and quality of life are strongly associated with the total volume, as well as temporal variation in activity. In addition, associations between physical activity and health outcomes are not modified by cancer status. Our findings suggest that employing a multilevel functional principal component analysis approach can elicit important temporal patterns in physical activity. It further allows us to study the relationship between health outcomes and activity patterns, and thus could be a valuable modeling approach in behavioral research.
更多查看译文
关键词
Accelerometer, Functional method, Principal component analysis, Physical activity patterns
AI 理解论文
溯源树
样例
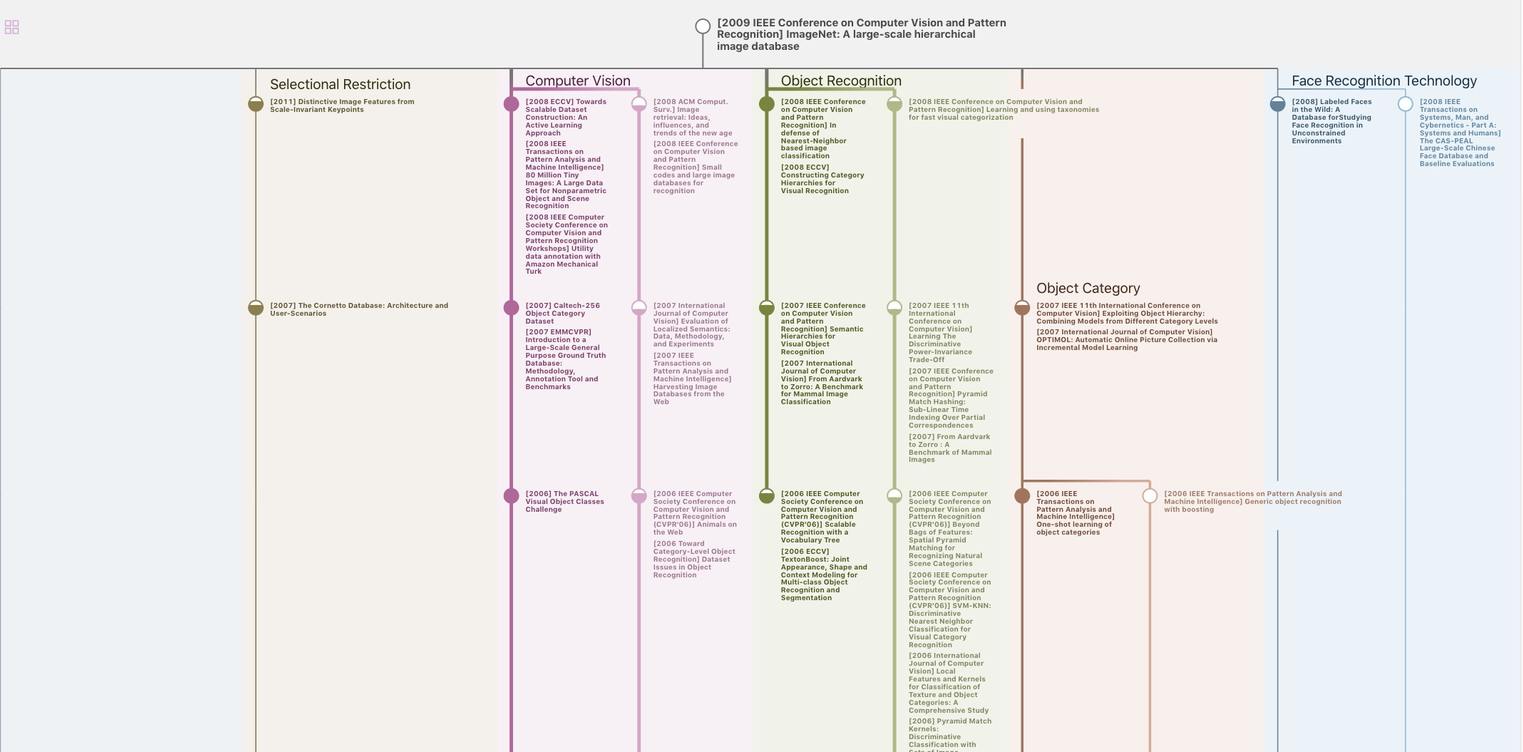
生成溯源树,研究论文发展脉络
Chat Paper
正在生成论文摘要