A distortion-agnostic video quality metric based on multi-scale spatio-temporal structural information.
Signal Processing: Image Communication(2019)
摘要
Considering the high sensitivity of human visual perception towards the structural information, a general purpose no-reference video quality assessment (NR-VQA) metric based on local binary patterns (LBP) is proposed. In the proposed metric, 2D LBP method is extended to third dimension using LBP-TOP (LBP from Three Orthogonal Planes) for extracting the spatio-temporal structural information. Further, spatio-temporal structural information is evaluated for multi-resolution local pixel windows to incorporate the human vision system (HVS) characteristic of multi-scale perception. Since LBP only encodes the sign information of local pixel differences, and ignores the degree of variations, a second set of feature based on the magnitude information of local pixel differences is extracted. The magnitude information based LBP-TOP feature set is accumulated on the sign information based LBP-TOP feature set such that the resultant feature set has more spatio-temporal structural information with same feature vector length. To emulate the HVS mechanism of quality feature mapping to a scalar quantity, a simple two-layer feed-forward artificial neural network (ANN) of single hidden layer with four neurons is proposed. The performance of the proposed metric is validated on LIVE, CSIQ, and IVP video quality datasets and consistent performance is observed. Further, the performance of proposed metric is compared with the existing state-of-the-art NR-VQA and other IQA/VQA metrics and have exhibited better performance than the others. The proposed metric is highly consistent in performance with low computational complexity and thus suitable for real-time applications.
更多查看译文
关键词
Video quality assessment (VQA),No-reference (NR),Local binary patterns (LBP),Three orthogonal planes (TOP),Artificial neural network (ANN),Video quality dataset
AI 理解论文
溯源树
样例
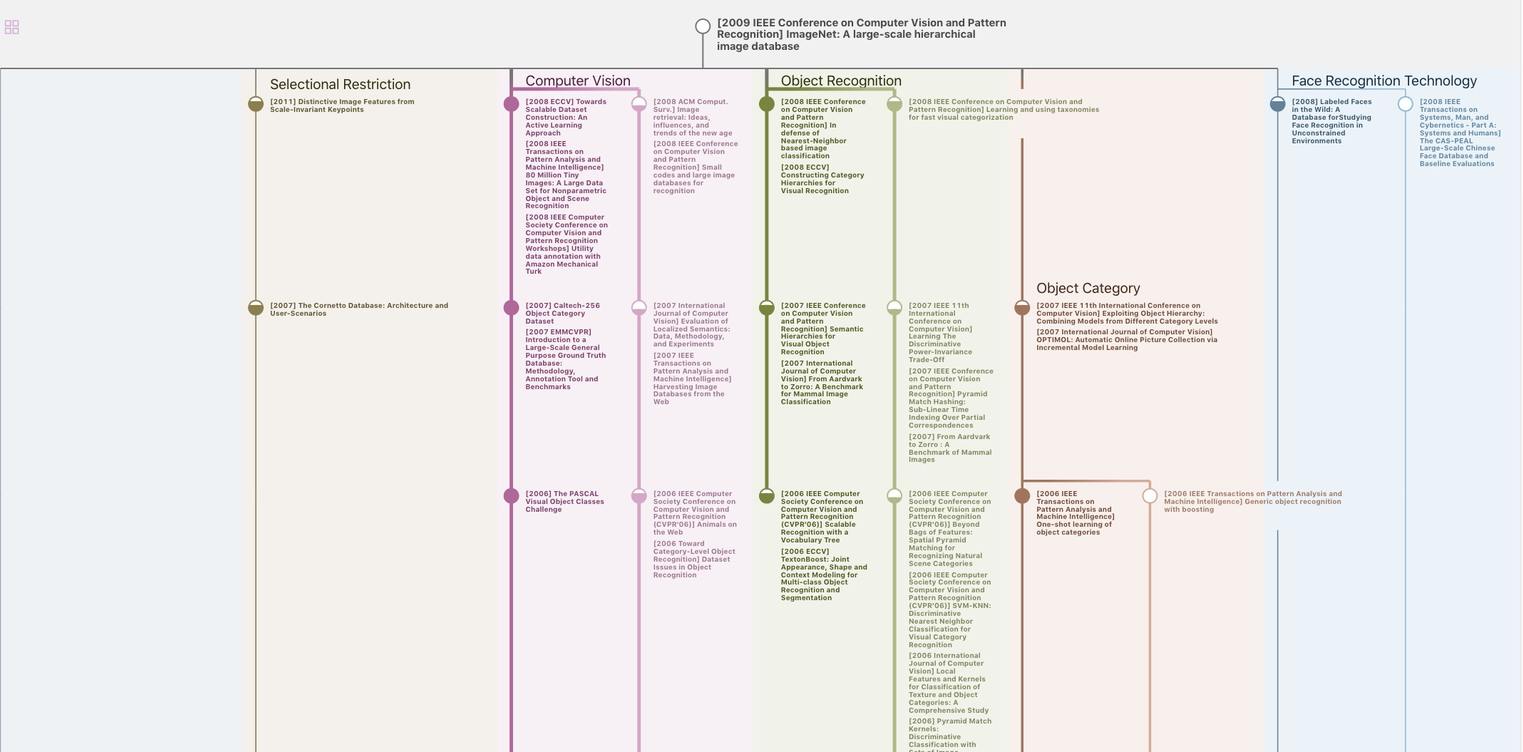
生成溯源树,研究论文发展脉络
Chat Paper
正在生成论文摘要