Unsupervised Topic Extraction from Privacy Policies
Companion Proceedings of The 2019 World Wide Web Conference(2019)
摘要
This paper suggests the use of automatic topic modeling for large-scale corpora of privacy policies using unsupervised learning techniques. The advantages of using unsupervised learning for this task are numerous. The primary advantages include the ability to analyze any new corpus with a fraction of the effort required by supervised learning, the ability to study changes in topics of interest along time, and the ability to identify finer-grained topics of interest in these privacy policies. Based on general principles of document analysis we synthesize a cohesive framework for privacy policy topic modeling and apply it over a corpus of 4,982 privacy policies of mobile applications crawled from the Google Play Store. The results demonstrate that even with this relatively moderate-size corpus quite comprehensive insights can be attained regarding the focus and scope of current privacy policy documents. The topics extracted, their structure and the applicability of the unsupervised approach for that matter are validated through an extensive comparison to similar findings reported in prior work that uses supervised learning (which heavily depends on manual annotation of experts). The comparison suggests a substantial overlap between the topics found and those reported in prior work, and also unveils some new topics of interest.
更多查看译文
关键词
Topic modeling, privacy policies, unsuprevised learning
AI 理解论文
溯源树
样例
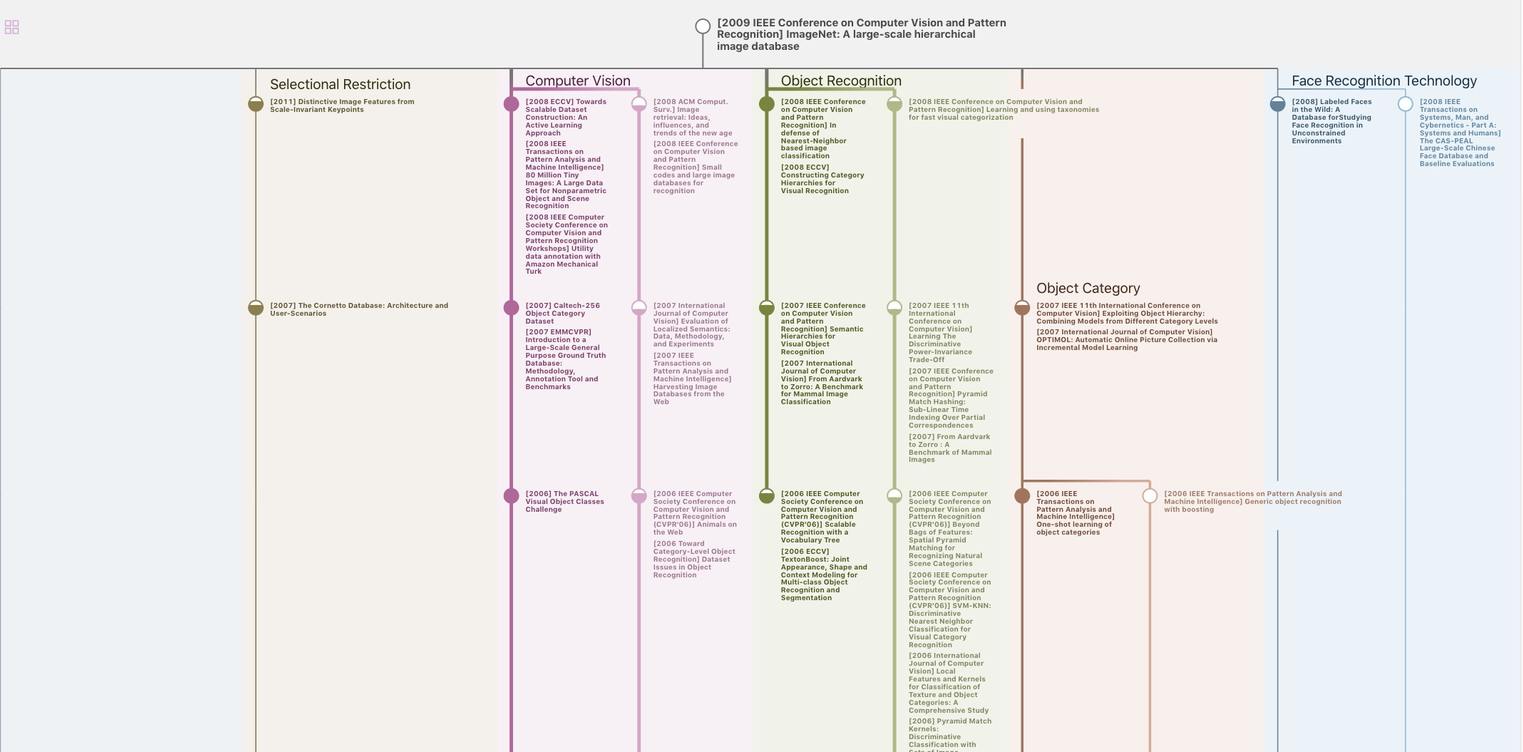
生成溯源树,研究论文发展脉络
Chat Paper
正在生成论文摘要