Addressing Trust Bias for Unbiased Learning-to-Rank
WWW '19: The Web Conference on The World Wide Web Conference WWW 2019(2019)
摘要
Existing unbiased learning-to-rank models use counterfactual inference, notably Inverse Propensity Scoring (IPS), to learn a ranking function from biased click data. They handle the click incompleteness bias, but usually assume that the clicks are noise-free, i.e., a clicked document is always assumed to be relevant. In this paper, we relax this unrealistic assumption and study click noise explicitly in the unbiased learning-to-rank setting. Specifically, we model the noise as the position-dependent trust bias and propose a noise-aware Position-Based Model, named TrustPBM, to better capture user click behavior. We propose an Expectation-Maximization algorithm to estimate both examination and trust bias from click data in TrustPBM. Furthermore, we show that it is difficult to use a pure IPS method to incorporate click noise and thus propose a novel method that combines a Bayes rule application with IPS for unbiased learning-to-rank. We evaluate our proposed methods on three personal search data sets and demonstrate that our proposed model can significantly outperform the existing unbiased learning-to-rank methods.
更多查看译文
关键词
Unbiased learning-to-rank, click noise, inverse propensity scoring, trust bias
AI 理解论文
溯源树
样例
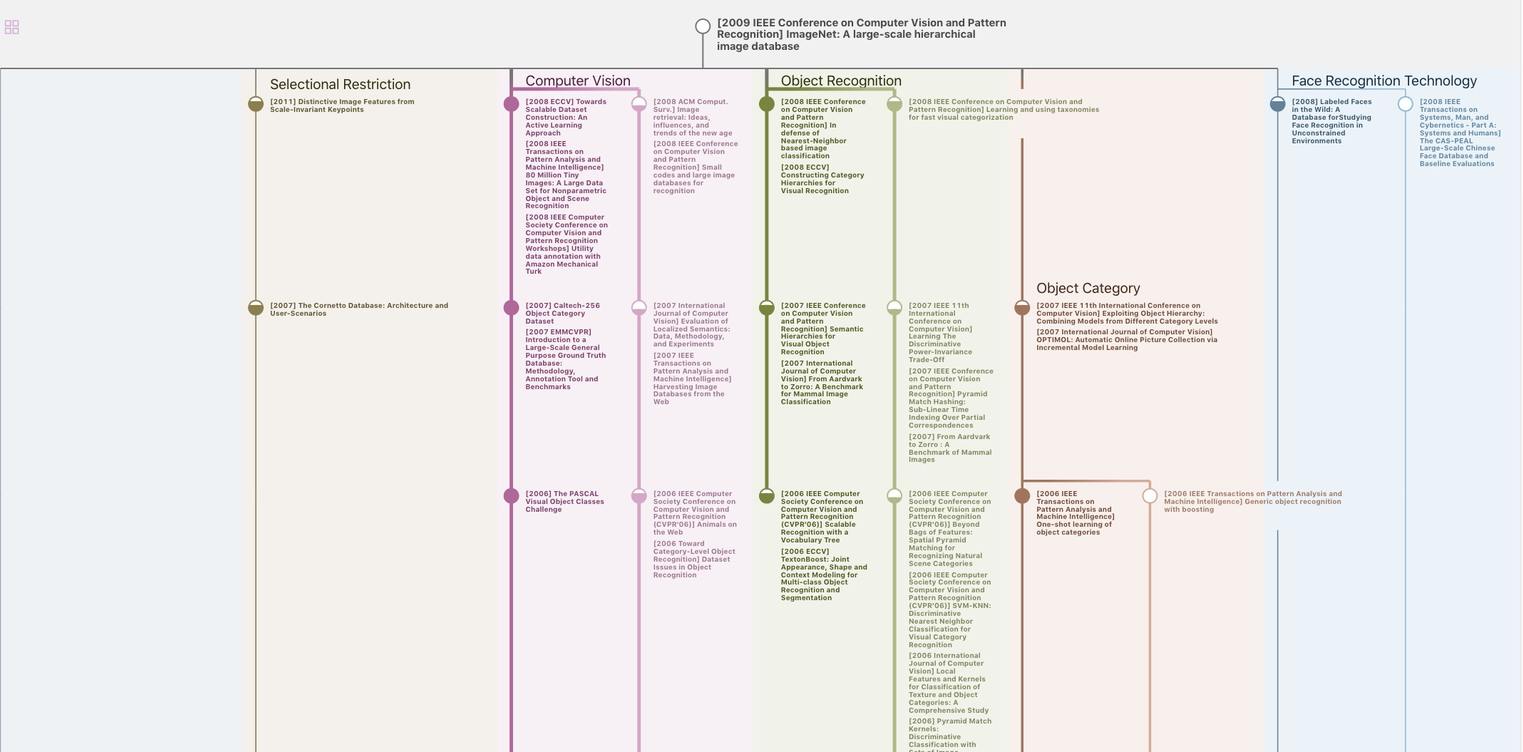
生成溯源树,研究论文发展脉络
Chat Paper
正在生成论文摘要