Graph Neural Networks for Social Recommendation
WWW '19: The Web Conference on The World Wide Web Conference WWW 2019(2019)
摘要
In recent years, Graph Neural Networks (GNNs), which can naturally integrate node information and topological structure, have been demonstrated to be powerful in learning on graph data. These advantages of GNNs provide great potential to advance social recommendation since data in social recommender systems can be represented as user-user social graph and user-item graph; and learning latent factors of users and items is the key. However, building social recommender systems based on GNNs faces challenges. For example, the user-item graph encodes both interactions and their associated opinions; social relations have heterogeneous strengths; users involve in two graphs (e.g., the user-user social graph and the user-item graph). To address the three aforementioned challenges simultaneously, in this paper, we present a novel graph neural network framework (GraphRec) for social recommendations. In particular, we provide a principled approach to jointly capture interactions and opinions in the user-item graph and propose the framework GraphRec, which coherently models two graphs and heterogeneous strengths. Extensive experiments on two real-world datasets demonstrate the effectiveness of the proposed framework GraphRec.
更多查看译文
关键词
Graph Neural Networks, Neural Networks, Recommender Systems, Social Network, Social Recommendation
AI 理解论文
溯源树
样例
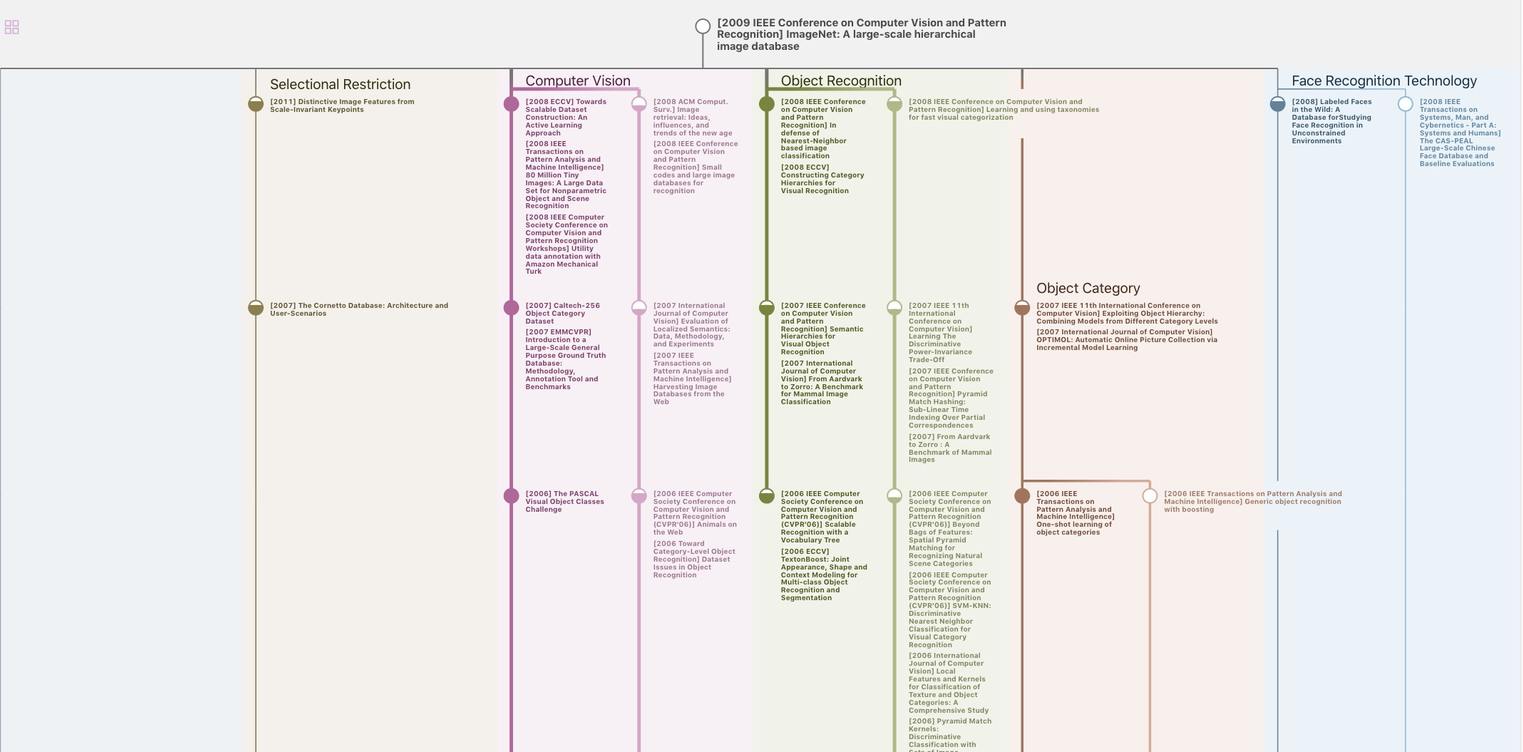
生成溯源树,研究论文发展脉络
Chat Paper
正在生成论文摘要