A data mining approach for population-based methods to solve the JSSP
Soft Computing(2018)
摘要
Up to now, many different methods (e.g., dispatching rules, metaheuristics) have been proposed to solve the job shop scheduling problem (JSSP), but the application of data mining approaches in the literature is limited. In this paper, we propose a data mining-based approach to generate an improved initial population for population-based heuristics/metaheuristics solving the JSSP. First, we apply a combination of ‘attribute-oriented induction’ and ‘association rule mining’ techniques to extract the rules behind the optimal or near-optimal schedules of JSSP. Then, a novel method called ‘Assignment Procedure’ is proposed to heuristically solve the JSSP using the extracted rules. The proposed method is able to generate numerous schedules for a given JSSP instance, and consequently, the generated solutions can be considered as the initial population for population-based solution methods. To evaluate the effectiveness of the proposed method, the two well-known metaheuristics are considered, i.e., genetic algorithm (GA) and particle swarm optimization (PSO). Extensive experiments were carried out on 35 benchmark problem instances, and Friedman test with post hoc Nemenyi test was performed to statistically analyze the results. The results revealed that using the initial populations generated by the proposed approach is very promising compared to the randomly generated population for both GA and PSO. Moreover, the experiments verify the significant amount of FEs that can be saved using the proposed approach and the superiority of the proposed method in comparison with the method of Koonce and Tsai (Comput Ind Eng 38:361–374, 2000 ) and four well-known dispatching rules in the literature.
更多查看译文
关键词
Scheduling, Job shop, Data mining, Population generation, Particle swarm optimization, Genetic algorithm
AI 理解论文
溯源树
样例
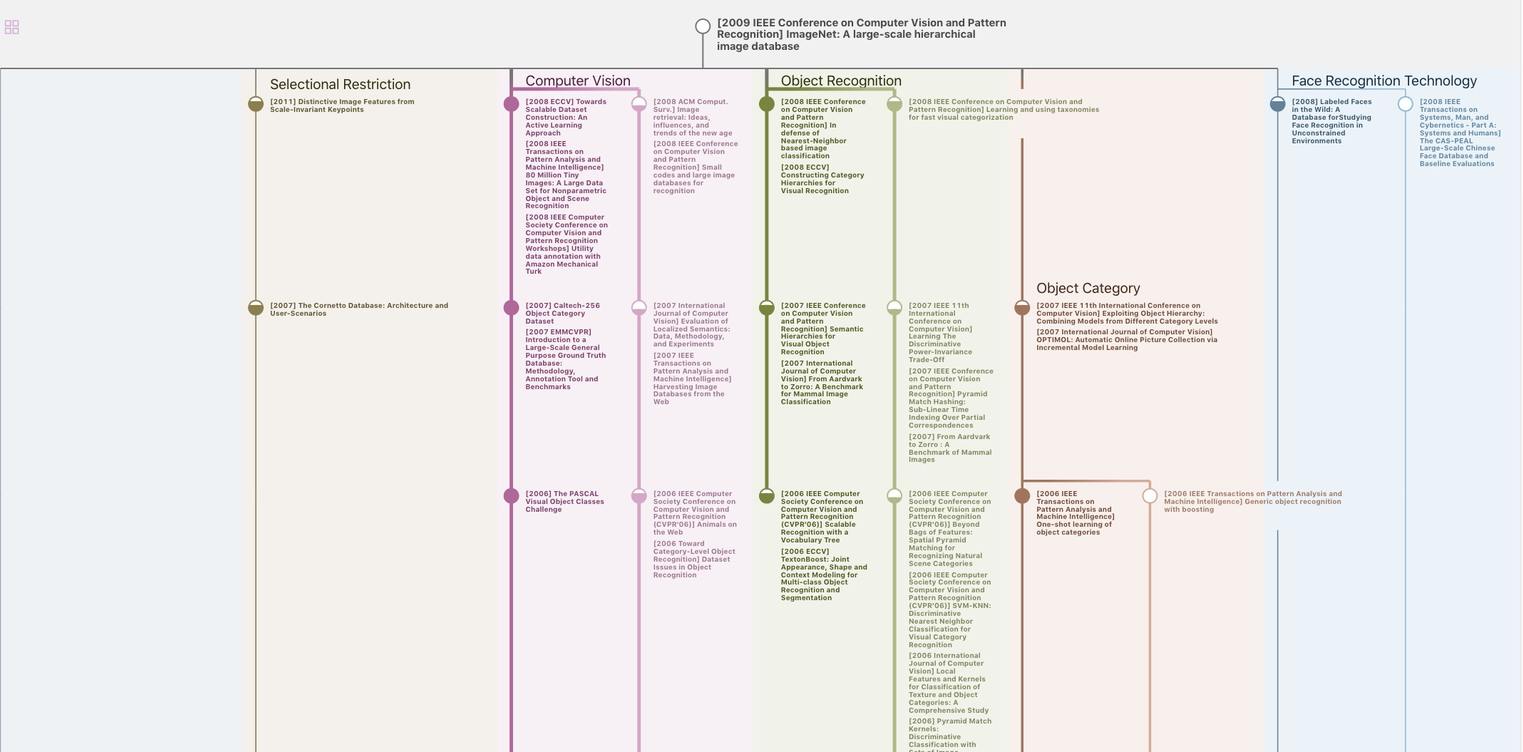
生成溯源树,研究论文发展脉络
Chat Paper
正在生成论文摘要