Decentralized iterative approaches for community clustering in the networks
The Journal of Supercomputing(2019)
摘要
In this era of big data, as the data size is scaling up, the need for computing power is exponentially increasing. However, most of the community detection algorithms in the literature are classified as global algorithms, which require access to the entire information of the network. These algorithms designed to work on a single machine cannot be directly parallelized. Hence, it is impossible for such algorithms working in stand-alone machines to find communities in large-scale networks and also the required processing power far exceeds the processing capabilities of single machines. In this paper, a set of novel Decentralized Iterative Community Clustering Approaches to extract an efficient community structure for large networks are proposed and devalued using the LFR benchmark model. The approaches have the ability to identify the community clusters from the entire network without global knowledge of the network topology and will work with a range of computer architecture platforms (e.g., cluster of PCs, multi-core distributed memory servers, GPUs). Detecting and characterizing such community structures is one of the fundamental topics in network systems’ analysis, and it has many important applications in different branches of science including computer science, physics, mathematics and biology ranging from visualization, exploratory and data mining to building prediction models.
更多查看译文
关键词
Community detection, Connectivity-based graph clustering, Distributed algorithm
AI 理解论文
溯源树
样例
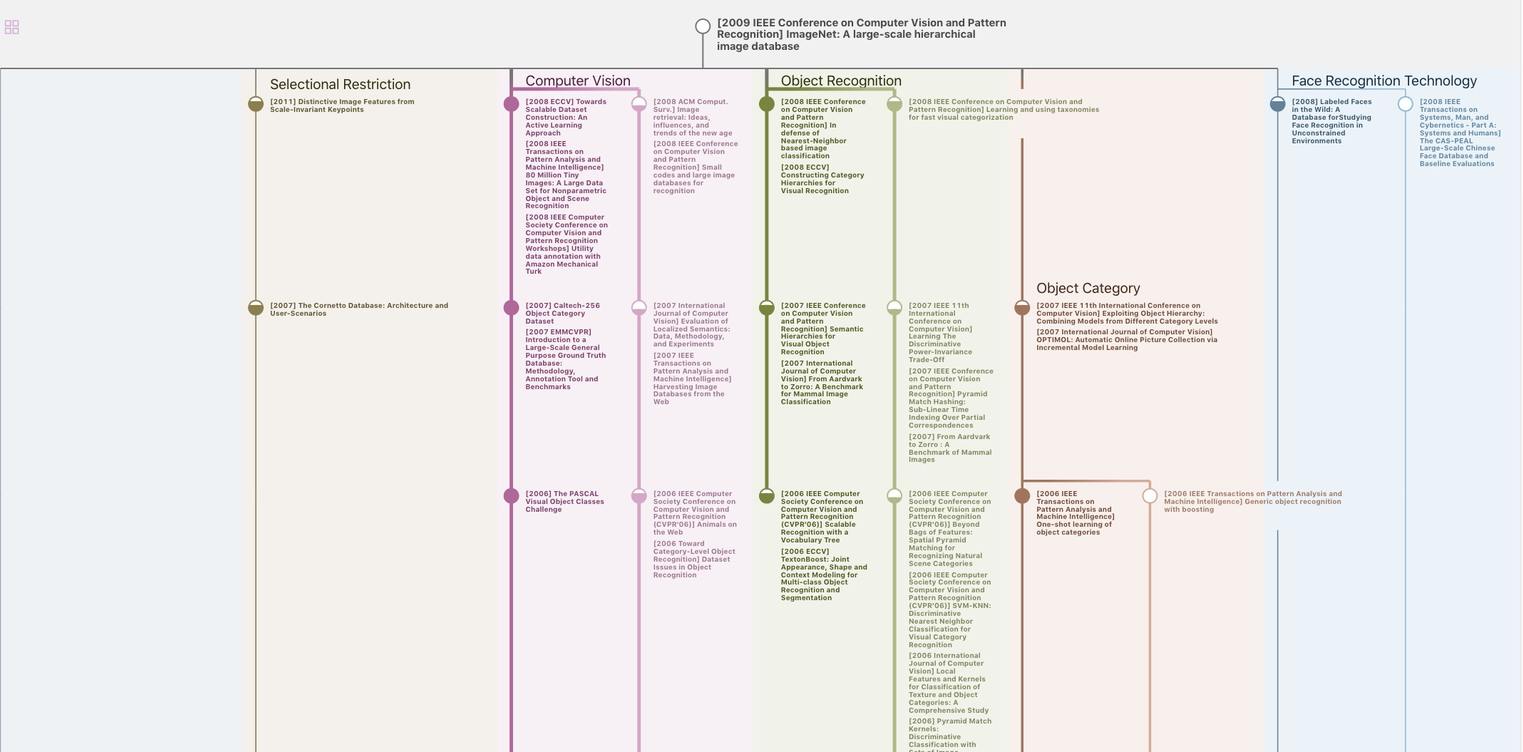
生成溯源树,研究论文发展脉络
Chat Paper
正在生成论文摘要