Customised ensemble methodologies for deep learning: Boosted Residual Networks and related approaches
Neural Computing and Applications(2018)
摘要
This paper introduces a family of new customised methodologies for ensembles, called Boosted Residual Networks, which builds a boosted ensemble of residual networks by growing the member network at each round of boosting. The proposed approach combines recent developments in residual networks—a method for creating very deep networks by including a shortcut layer between different groups of layers—with Deep Incremental Boosting, a methodology to train fast ensembles of networks of increasing depth through the use of boosting. Additionally, we explore a simpler variant of Boosted Residual Networks based on bagging, called Bagged Residual Networks. We then analyse how the recent developments in ensemble distillation can improve our results. We demonstrate that the synergy of residual networks and Deep Incremental Boosting has better potential than simply boosting a residual network of fixed structure or using the equivalent Deep Incremental Boosting without the shortcut layers, by permitting the creation of models with better generalisation in significantly less time.
更多查看译文
AI 理解论文
溯源树
样例
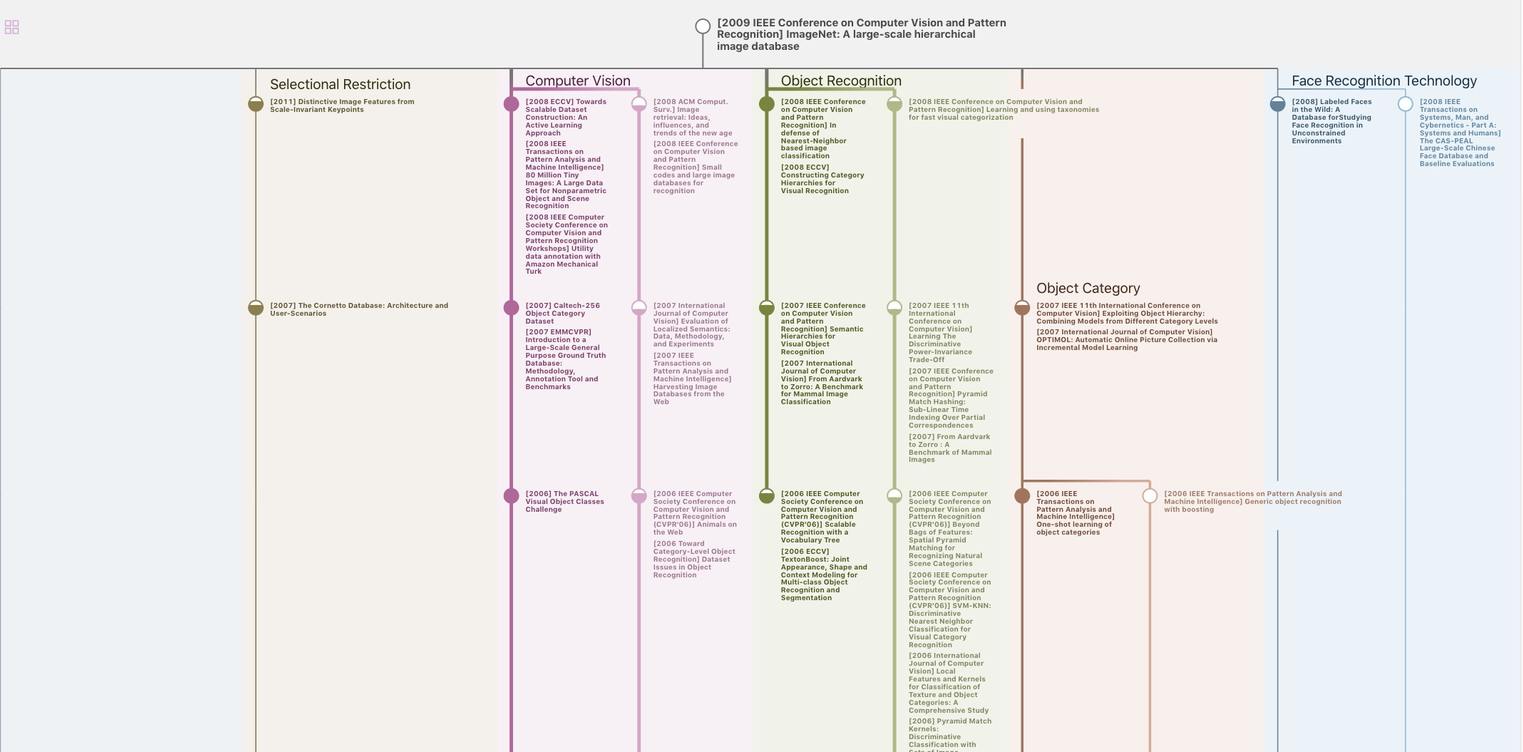
生成溯源树,研究论文发展脉络
Chat Paper
正在生成论文摘要