Unsupervised nonlinear feature selection algorithm via kernel function
Neural Computing and Applications(2018)
摘要
Feature selection is one of the important methods of data preprocessing, but the general feature selection algorithm has the following shortcomings: (1) Noise and outliers cannot be ruled out so that the algorithm does not work well. (2) They only consider the linear relationship between data without considering the nonlinear relationship between data. For this reason, an unsupervised nonlinear feature selection algorithm via kernel function is proposed in this paper. First, each data feature is mapped to a kernel space by a kernel function. In this way, nonlinear feature selection can be performed. Secondly, the low-rank processing of the kernel coefficient matrix is used to eliminate the interference of noise samples. Finally, the feature selection is performed through a sparse regularization factor in the kernel space. Experimental results show that our algorithm has better results than contrast algorithms.
更多查看译文
关键词
Feature selection, Kernel function, Sparse regularization factor
AI 理解论文
溯源树
样例
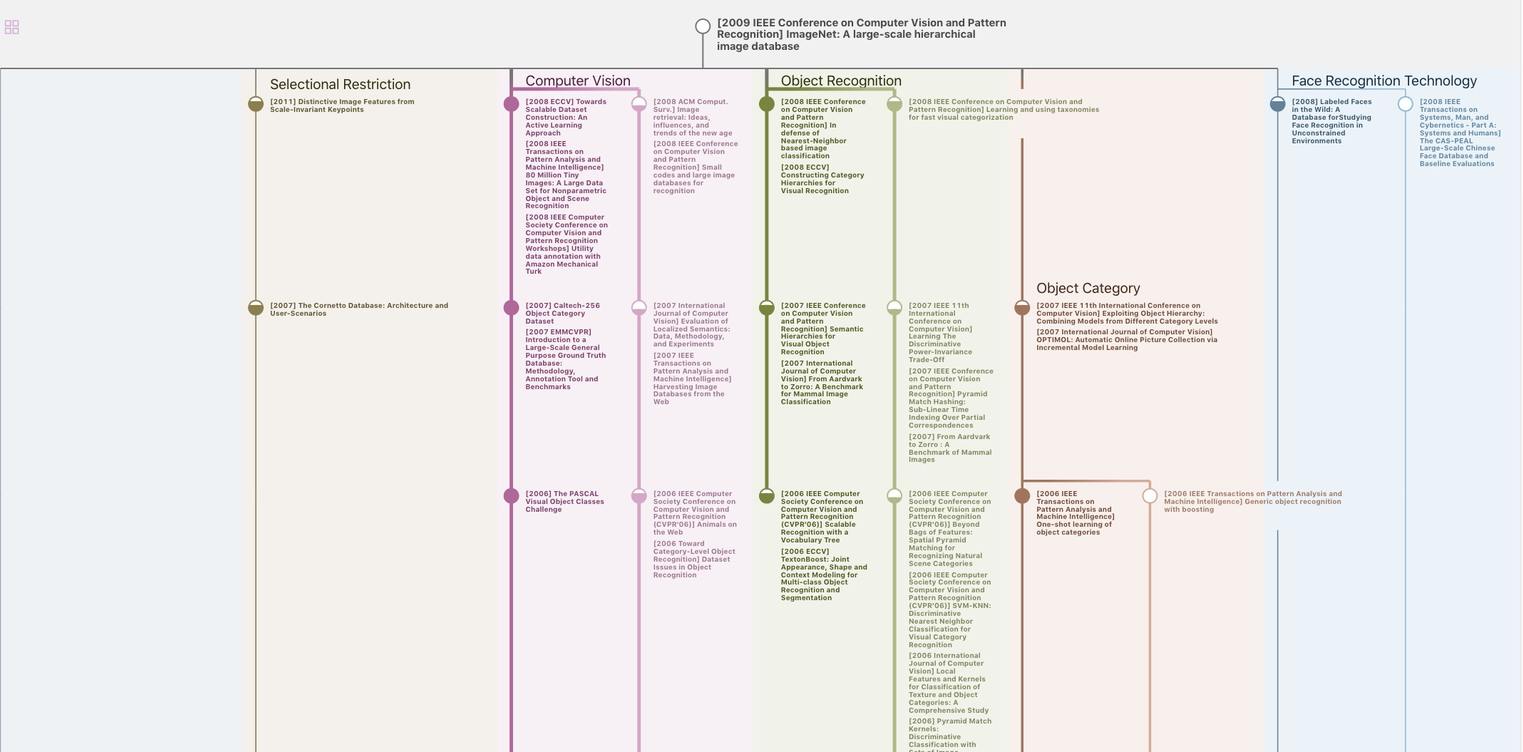
生成溯源树,研究论文发展脉络
Chat Paper
正在生成论文摘要