Cell clustering for spatial transcriptomics data with graph neural networks
Nature Computational Science(2022)
摘要
Spatial transcriptomics data can provide high-throughput gene expression profiling and the spatial structure of tissues simultaneously. Most studies have relied on only the gene expression information but cannot utilize the spatial information efficiently. Taking advantage of spatial transcriptomics and graph neural networks, we introduce cell clustering for spatial transcriptomics data with graph neural networks, an unsupervised cell clustering method based on graph convolutional networks to improve ab initio cell clustering and discovery of cell subtypes based on curated cell category annotation. On the basis of its application to five in vitro and in vivo spatial datasets, we show that cell clustering for spatial transcriptomics outperforms other spatial clustering approaches on spatial transcriptomics datasets and can clearly identify all four cell cycle phases from multiplexed error-robust fluorescence in situ hybridization data of cultured cells. From enhanced sequential fluorescence in situ hybridization data of brain, cell clustering for spatial transcriptomics finds functional cell subtypes with different micro-environments, which are all validated experimentally, inspiring biological hypotheses about the underlying interactions among the cell state, cell type and micro-environment. A graph neural network-based cell clustering model for spatial transcripts obtains cell embeddings from global cell interactions across tissue samples and identifies cell types and subpopulations.
更多查看译文
关键词
Computational models,Data processing,Machine learning,Transcriptomics,Computer Science,general
AI 理解论文
溯源树
样例
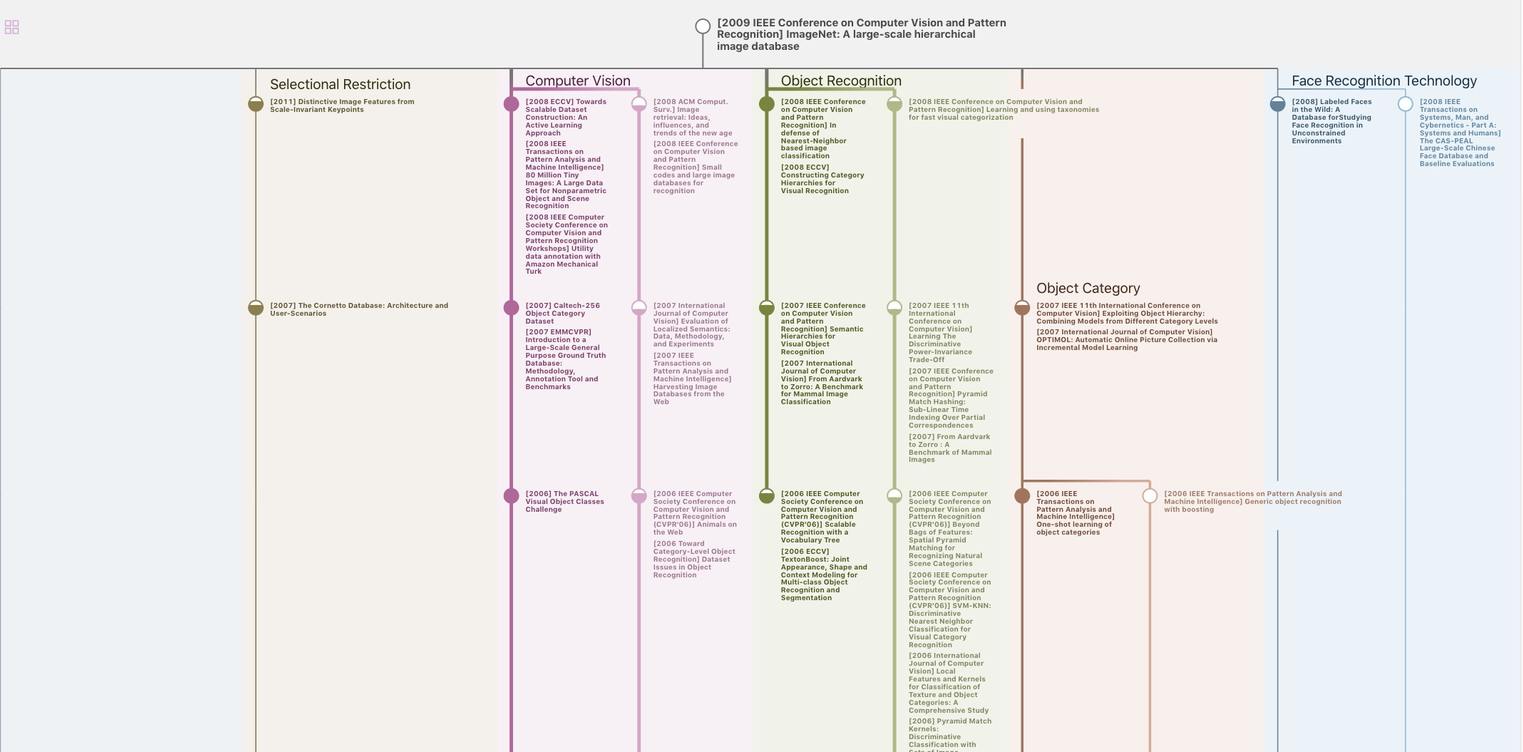
生成溯源树,研究论文发展脉络
Chat Paper
正在生成论文摘要