Laplacian smoothing gradient descent
Research in the Mathematical Sciences(2022)
摘要
We propose a class of very simple modifications of gradient descent and stochastic gradient descent leveraging Laplacian smoothing. We show that when applied to a large variety of machine learning problems, ranging from logistic regression to deep neural nets, the proposed surrogates can dramatically reduce the variance, allow to take a larger step size, and improve the generalization accuracy. The methods only involve multiplying the usual (stochastic) gradient by the inverse of a positive definitive matrix (which can be computed efficiently by FFT) with a low condition number coming from a one-dimensional discrete Laplacian or its high-order generalizations. Given any vector, e.g., gradient vector, Laplacian smoothing preserves the mean and increases the smallest component and decreases the largest component. Moreover, we show that optimization algorithms with these surrogates converge uniformly in the discrete Sobolev H_σ ^p sense and reduce the optimality gap for convex optimization problems. The code is available at: https://github.com/BaoWangMath/LaplacianSmoothing-GradientDescent .
更多查看译文
关键词
Laplacian smoothing, Machine learning, Optimization
AI 理解论文
溯源树
样例
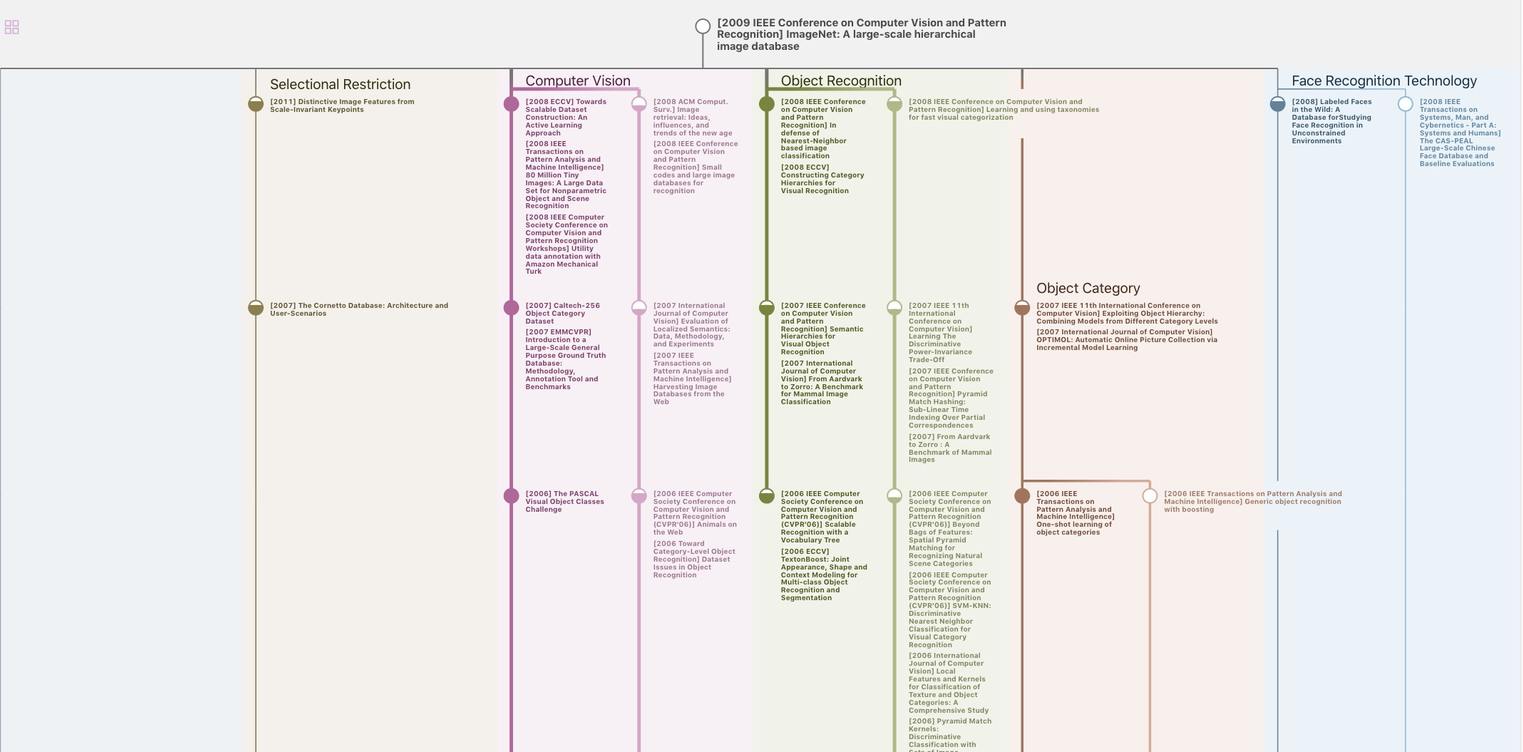
生成溯源树,研究论文发展脉络
Chat Paper
正在生成论文摘要