Modeling Heterogeneous Influences for Point-of-Interest Recommendation in Location-Based Social Networks.
ICWE(2019)
摘要
The huge amount of heterogeneous information in location-based social networks (LBSNs) creates great challenges for POI recommendation. User check-in behavior exhibits two properties, diversity and imbalance. To effectively model both properties, we propose an Aspectaware Geo-Social Matrix Factorization (AGS-MF) approach to exploit various factors in a unified manner for more effective POI recommendation. Specifically, we first construct a novel knowledge graph (KG), named as Aspect-aware Geo-Social Influence Graph (AGS-IG), to unify multiple influential factors by integrating the heterogeneous information about users, POIs and aspects from reviews. We design an efficient meta-path based random walk to discover relevant neighbors of each user and POI based on multiple influential factors. The extracted neighbors are further incorporated into AGS-MF with automatically learned personalized weights for each user and POI. By doing so, both diversity and imbalance can be modeled for better capturing the characteristics of users and POIs. Experimental results on several real-world datasets demonstrate that AGS-MF outperforms state-of-the-art methods.
更多查看译文
关键词
Location-based social network, POI recommendation, Knowledge graph, Matrix factorization
AI 理解论文
溯源树
样例
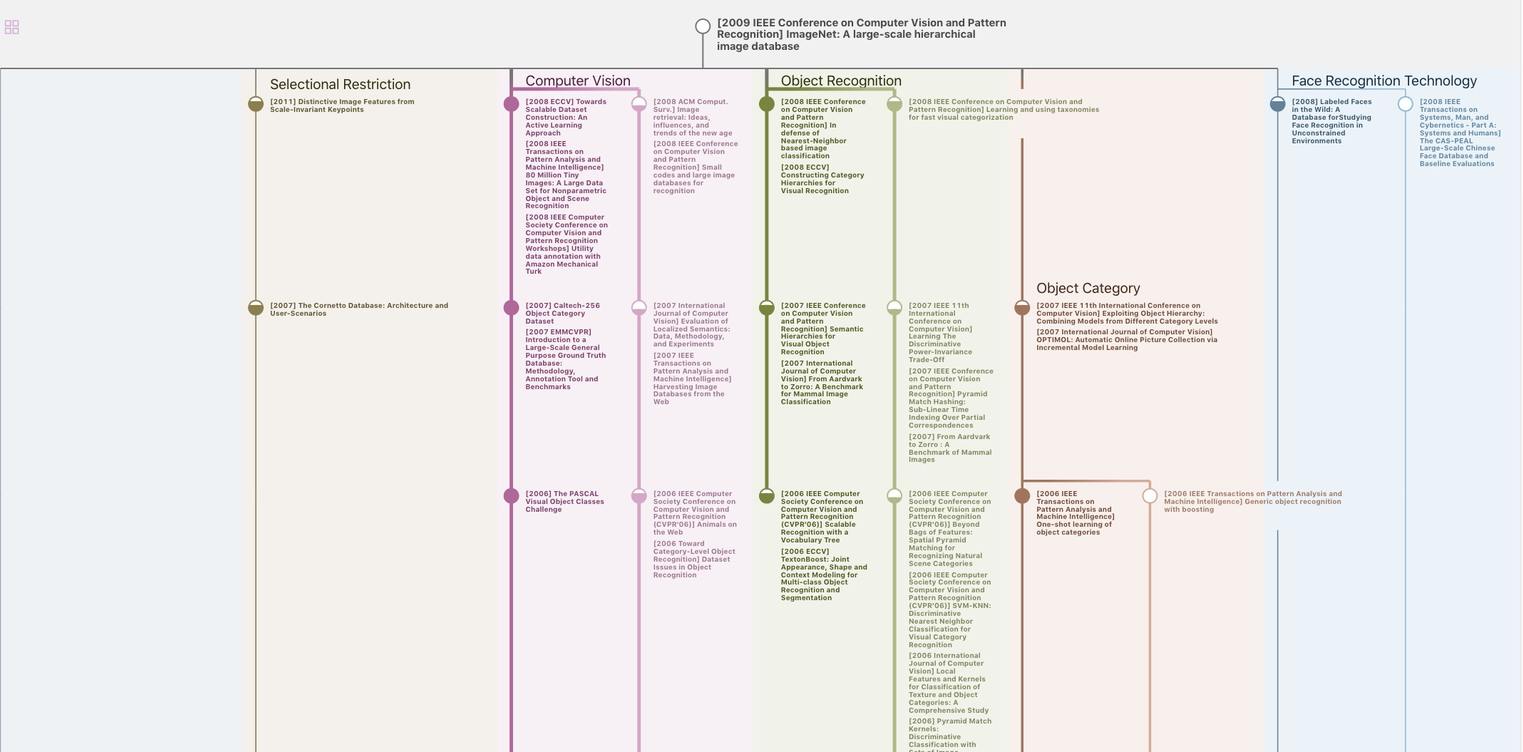
生成溯源树,研究论文发展脉络
Chat Paper
正在生成论文摘要