Hierarchical Gaussian descriptor based on local pooling for action recognition
Mach. Vis. Appl.(2018)
摘要
In this paper, we propose a new approach based on Gaussian descriptors for action recognition. We first develop a feature representation technique that encodes high-order statistics of local features in two levels, where single Gaussians are used to capture the distributions involved. To deal with the possible loss of information about the distribution of features caused by heterogeneous feature vectors when summarizing them, we use K-means clustering and Sparse Coding to construct some sets of feature vectors over which the summarization is performed. We then present two methods based on depth images and pose data for action recognition. In both methods, the proposed feature representation technique is applied to effectively obtain discriminative action descriptors. Experimental evaluation on the seven benchmark datasets, i.e., MSRAction3D, MSRGesture3D, DHA, SKIG, Florence, UTKinect, and HDM05, shows that our methods achieve very promising results on all the datasets.
更多查看译文
关键词
Action recognition, Covariance descriptor, Gaussian descriptor, Riemannian manifold, Lie group, Symmetric Positive Definite matrices
AI 理解论文
溯源树
样例
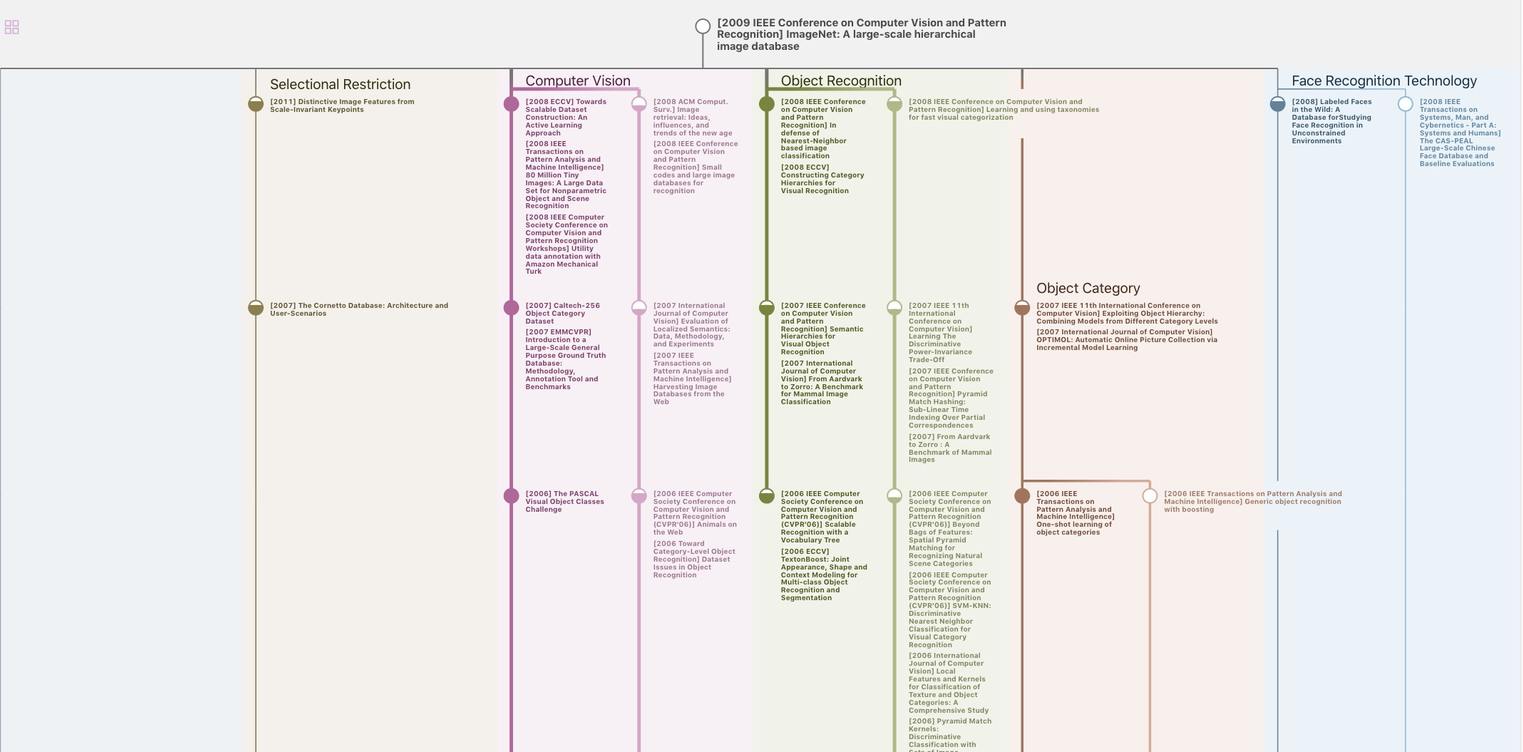
生成溯源树,研究论文发展脉络
Chat Paper
正在生成论文摘要