Compressed Sensing via a Deep Convolutional Auto-encoder
2018 IEEE Visual Communications and Image Processing (VCIP)(2018)
摘要
The nonlinear recovery is not promising in accuracy and speed, which limits the practical usage of compressed sensing (CS). This paper proposes a deep learning-based CS framework which leverages a deep convolutional auto-encoder for image sensing and recovery. The utilized auto-encoder architecture consists of three components: the fully convolutional network acts as an adaptive measurement matrix generator in the encoder; while in the decoder, the deconvolution network and refined reconstruction network are learned for intermediate and final recovery, respectively. Different from most previous work focusing on the block-wise manner to reduce implementation cost but result in blocky artifacts, our adaptive measurement matrix is applicable to any size of scene image and the decoder network reconstructs the whole image efficiently without any blocky artifacts. Moreover, dense connectivity is leveraged to combine multi-level features and alleviate the vanishing-gradient problem in the refined reconstruction network which boosts the performance on image recovery. Compared to the state-of-the-art methods, our algorithm improves more than 0.8 dB in average PSNR.
更多查看译文
关键词
Compressed sensing,Adaptive measurement matrix,Convolutional auto-encoder,Image reconstruction
AI 理解论文
溯源树
样例
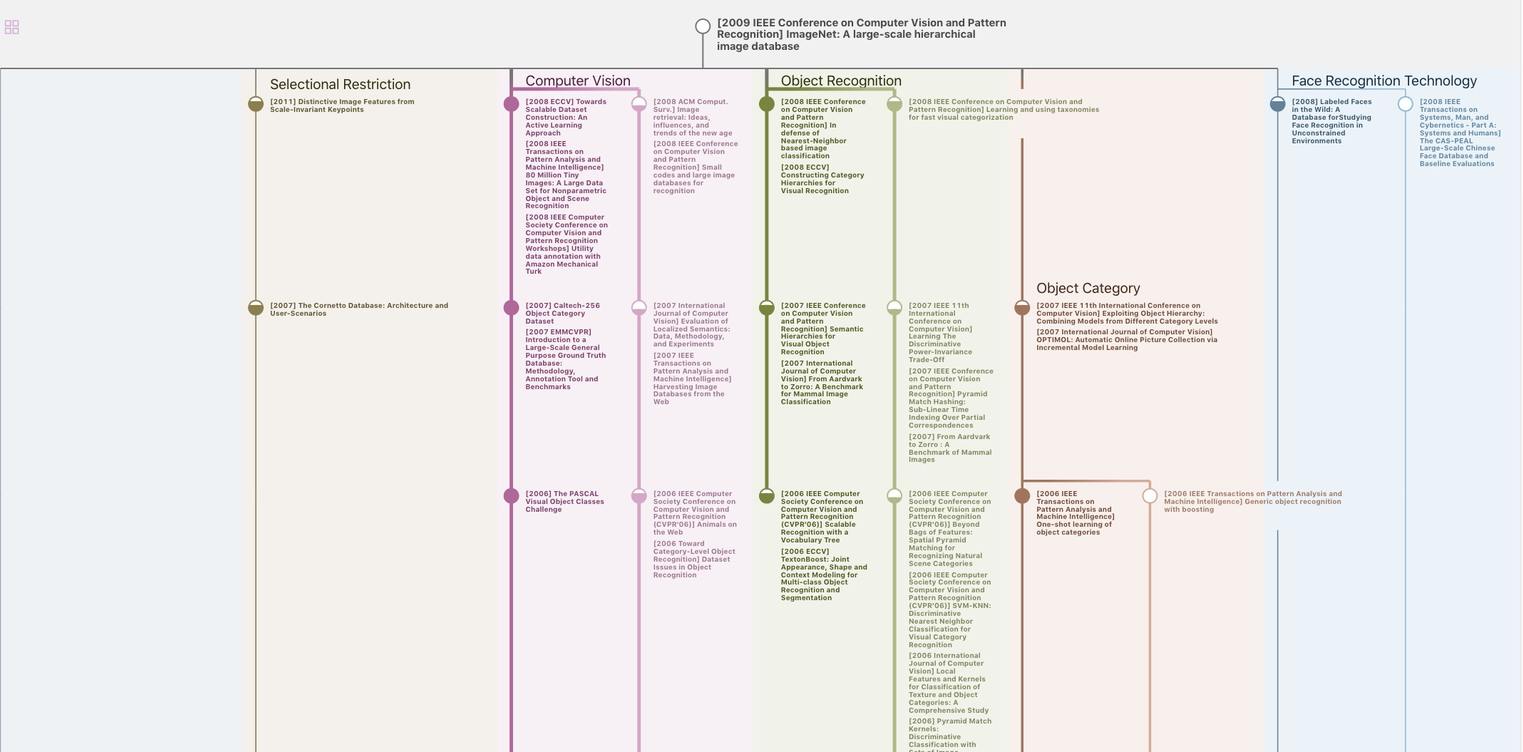
生成溯源树,研究论文发展脉络
Chat Paper
正在生成论文摘要