Clustering of interval-valued time series of unequal length based on improved dynamic time warping.
Expert Systems with Applications(2019)
摘要
Clustering of a group of interval-valued time series of unequal length is often encountered and the key point of this clustering is the distance measure between two interval-valued time series. However, most distance measure methods apply to interval-valued time series of equal length, and another methods applicable to unequal-length ones usually show high computational cost. In order to give a reasonable and efficient distance measure, this paper first proposes a new representation in the form of a sequence of 3-tuples for interval-valued time series. In this representation, fully take into account the time-axis and value-axis information to decrease the loss of information. Meanwhile, this representation is guaranteed to achieve dimensionality reduction. Based on the new representation, dynamic time warping algorithm is then employed and an improved dynamic time warping algorithm is produced. Furthermore, a hierarchical clustering algorithm based on the new proposed distance measure is designed for interval-valued time series of equal or unequal length. Experimental results show the effectiveness of the proposed distance and quantify the performance of the designed clustering method.
更多查看译文
关键词
Interval-valued time series,Unequal length,Hierarchical clustering,Distance measure,Dynamic time warping
AI 理解论文
溯源树
样例
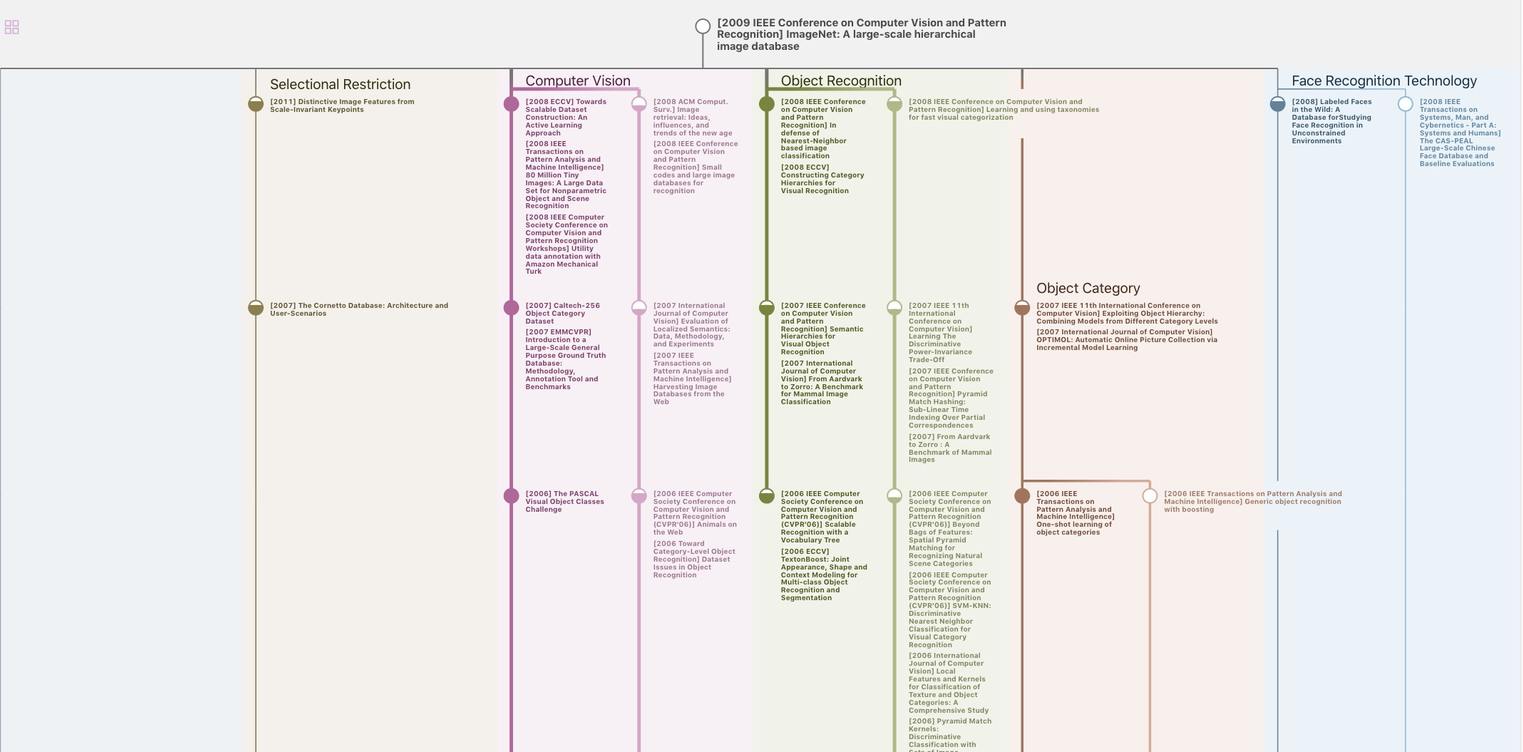
生成溯源树,研究论文发展脉络
Chat Paper
正在生成论文摘要