A feature-based approach to predict hot spots in protein-DNA binding interfaces.
BRIEFINGS IN BIOINFORMATICS(2020)
摘要
DNA-binding hot spot residues of proteins are dominant and fundamental interface residues that contribute most of the binding free energy of protein-DNA interfaces. As experimental methods for identifying hot spots are expensive and time consuming, computational approaches are urgently required in predicting hot spots on a large scale. In this work, we systematically assessed a wide variety of 114 features from a combination of the protein sequence, structure, network and solvent accessible information and their combinations along with various feature selection strategies for hot spot prediction. We then trained and compared four commonly used machine learning models, namely, support vector machine (SVM), random forest, Naive Bayes and k-nearest neighbor, for the identification of hot spots using 10-fold cross-validation and the independent test set. Our results show that (1) features based on the solvent accessible surface area have significant effect on hot spot prediction; (2) different but complementary features generally enhance the prediction performance; and (3) SVM outperforms other machine learning methods on both training and independent test sets. In an effort to improve predictive performance, we developed a feature-based method, namely, PrPDH (Prediction of Protein-DNA binding Hot spots), for the prediction of hot spots in protein-DNA binding interfaces using SVM based on the selected 10 optimal features. Comparative results on benchmark data sets indicate that our predictor is able to achieve generally better performance in predicting hot spots compared to the state-of-the-art predictors. A user-friendly web server for PrPDH is well established and is freely available at http://bioinfo.ahu.edu.cn:8080/PrPDH.
更多查看译文
关键词
machine learning,feature selection,protein-DNA interaction,hot spot,support vector machine
AI 理解论文
溯源树
样例
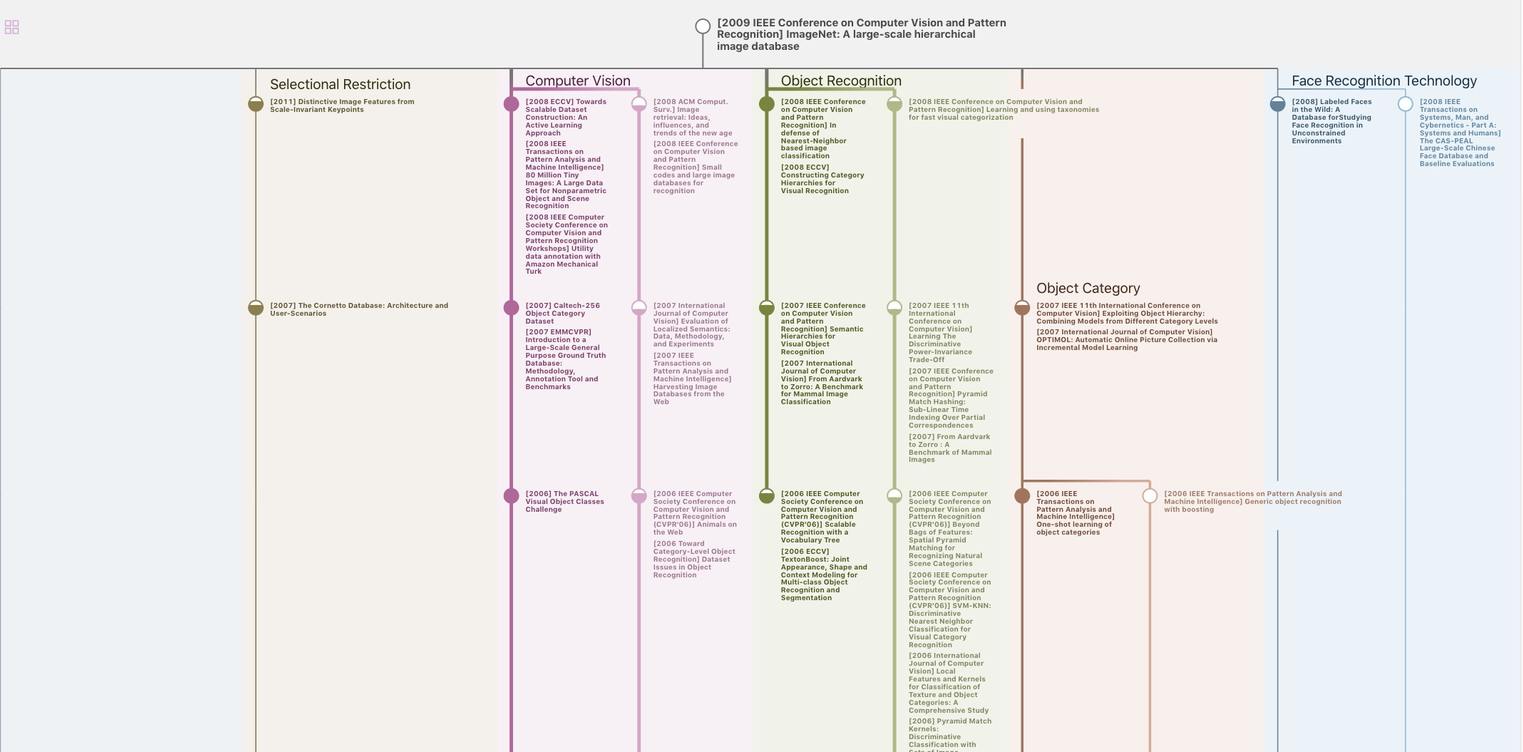
生成溯源树,研究论文发展脉络
Chat Paper
正在生成论文摘要