Unsupervised anomaly detection in underwater acoustic sensor networks.
JOURNAL OF INTELLIGENT & FUZZY SYSTEMS(2019)
摘要
An underwater acoustic sensor network (UASN) offers a promising solution for the exploration of underwater resources remotely. As the UASN acoustic channel is open and the environment is hostile, the risk of malicious activities is very high, particularly in time-critical military applications. In this paper, we propose an unsupervised anomaly detection system by learning the social behavioral correlation among nodes. The location data retrieved from sensors are learned using long short term memory (LSTM) networks to capture the anomalous nature. The network is simulated by modeling anomalies and analyzed the performance. The analysis of results indicates that the anomaly detection system offers an acceptable accuracy with high true positive rate and F-Score by showing consistency in multiple mobility behavior.
更多查看译文
关键词
Underwater sensor networks,time series analysis,anomaly detection,long shot term memory network
AI 理解论文
溯源树
样例
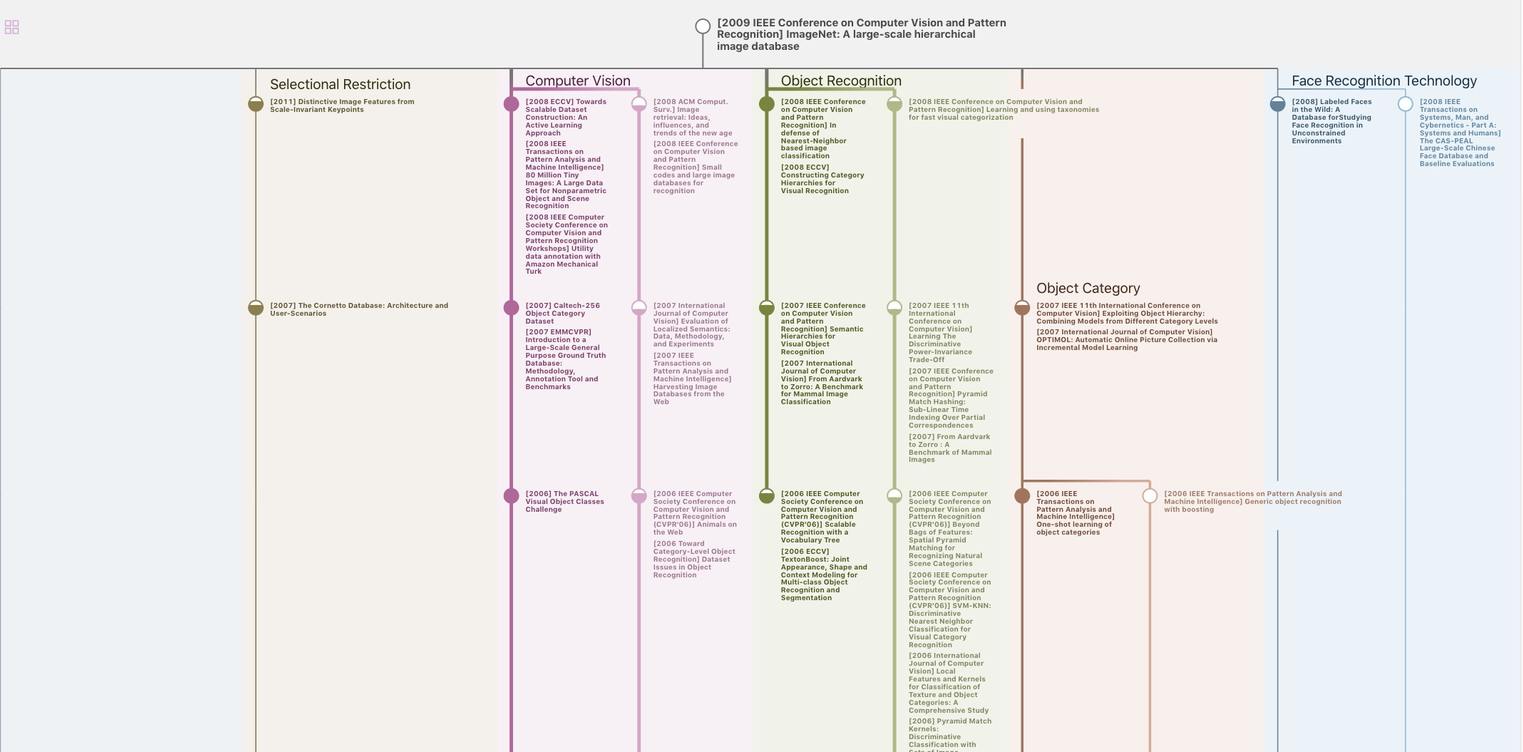
生成溯源树,研究论文发展脉络
Chat Paper
正在生成论文摘要