Data-Driven Haptic Modeling Of Normal Interactions On Viscoelastic Deformable Objects Using A Random Forest
IEEE ROBOTICS AND AUTOMATION LETTERS(2019)
摘要
In this letter, we propose a new data-driven approach for haptic modeling of normal interactions on homogeneous viscoelastic deformable objects. The approach is based on a well-known machine learning technique: random forest. Here, we employ a random forest for regression. We acquire discrete-time interaction data for many automated cyclic compressions of a deformable object. A random forest is trained to estimate a nonparametric relationship between the position and response forces. We train the forest on very simple normal interactions. Our results show that a model trained with just 10% of the training data is capable of modeling other unseen complex normal homogeneous interactions with good accuracy. Thus, it can handle large and complex datasets. In addition, our approach requires five times less training data than the standard approach in the literature to provide similar accuracy.
更多查看译文
关键词
Contact modeling, physical human-robot interaction, virtual reality, haptic interfaces
AI 理解论文
溯源树
样例
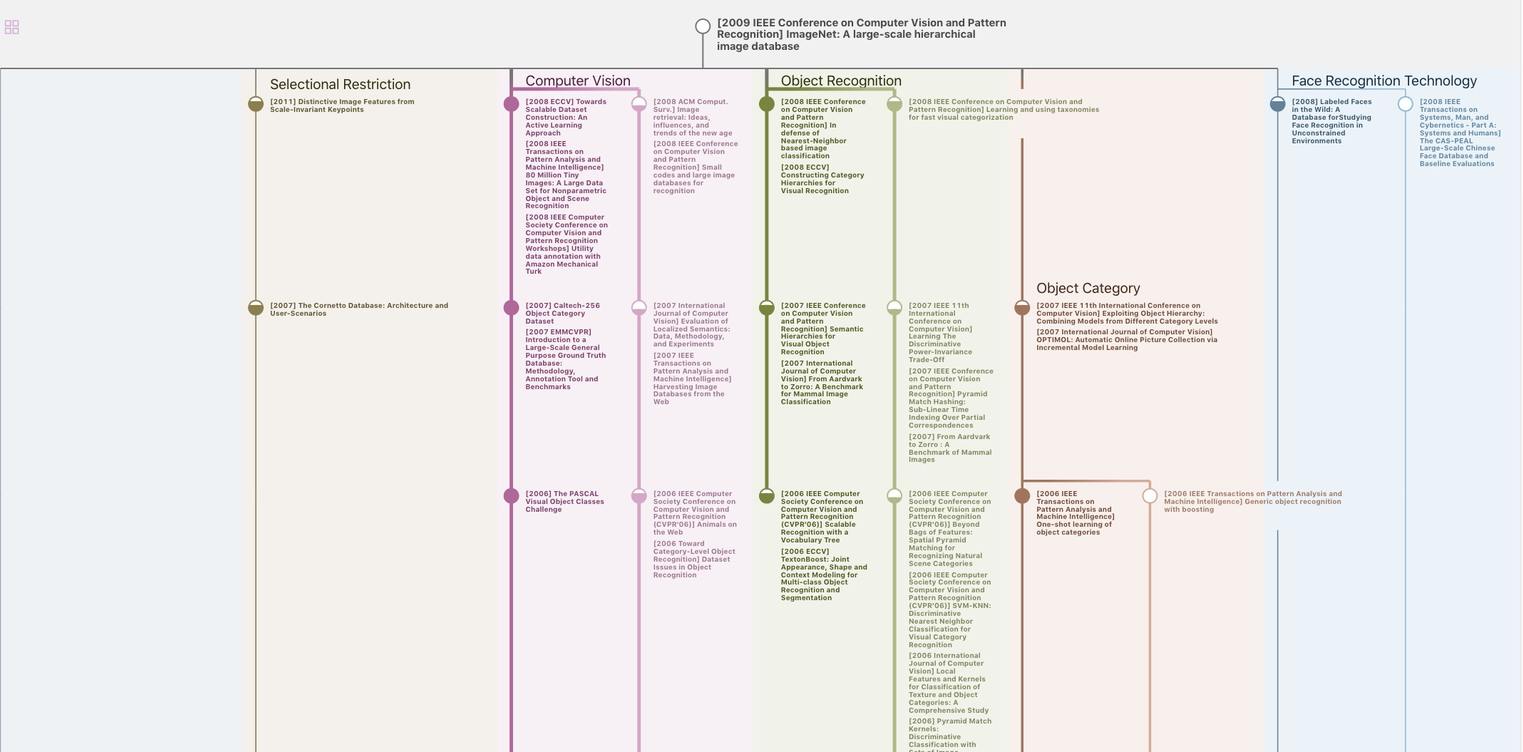
生成溯源树,研究论文发展脉络
Chat Paper
正在生成论文摘要