Sparse Bayesian Learning-Based Adaptive Impedance Control In Physical Human-Robot Interaction
2018 IEEE INTERNATIONAL CONFERENCE ON ROBOTICS AND BIOMIMETICS (ROBIO)(2018)
摘要
For the sake of reducing human partner's effort (operating force and time) in human-robot interaction (HRI), it is of significant importance for robot to modify its impedance parameters dynamically based on human intention. Thus, in this paper, a data-driven adaptive impedance control (AIC) scheme is proposed, including a Sparse Bayesian learning-based (SBL) human intention predictor (HIP) and a variable impedance controller (VIC). And it works as follows: First, HIP is proposed to predict human partner's future intention by using necessary time-series data. Then, the predicted intention is used as an input to modulate impedance parameters by VIC. Thus, the dynamic characteristics of robot is suitable for operator's coming actions. Based on this, robot can adaptively comply to human partner better. The proposed method is verified by simulation on a 2 degrees of freedom (DOF) robot and experiments on a 6-DOF UR5 robot. Results reveal the feasibility and effectiveness of the proposed scheme in interaction process.
更多查看译文
关键词
UR5 robot,physical human-robot interaction,human intention predictor,variable impedance controller,time-series data,sparse Bayesian learning-based adaptive impedance control,data-driven adaptive impedance control,robot dynamic model
AI 理解论文
溯源树
样例
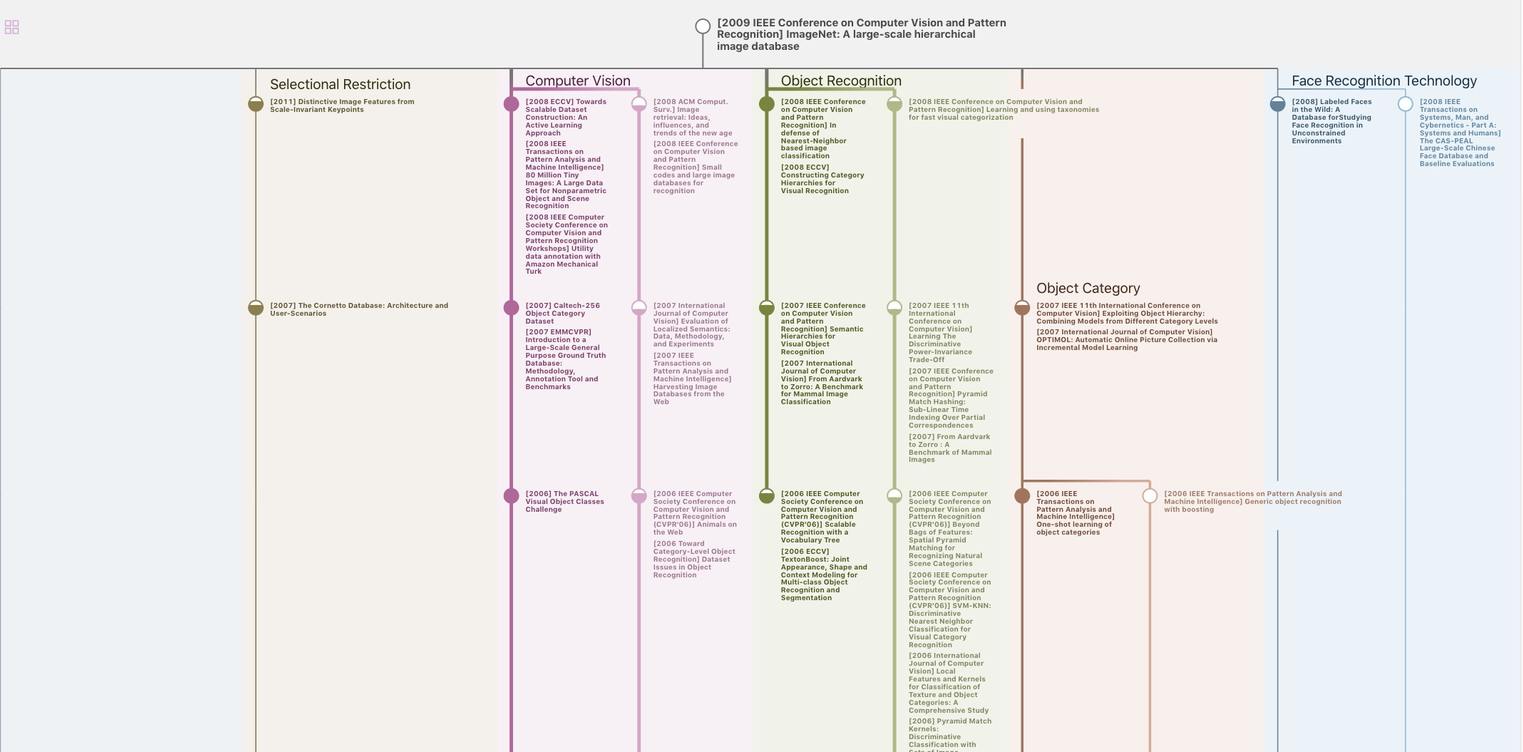
生成溯源树,研究论文发展脉络
Chat Paper
正在生成论文摘要