Improving Transferability Of Adversarial Examples With Input Diversity
2019 IEEE/CVF CONFERENCE ON COMPUTER VISION AND PATTERN RECOGNITION (CVPR 2019)(2019)
摘要
Though CNNs have achieved the state-of-the-art performance on various vision tasks, they are vulnerable to adversarial examples - crafted by adding human-imperceptible perturbations to clean images. However, most of the existing adversarial attacks only achieve relatively low success rates under the challenging black-box setting, where the attackers have no knowledge of the model structure and parameters. To this end, we propose to improve the transferability of adversarial examples by creating diverse input patterns. Instead of only using the original images to generate adversarial examples, our method applies random transformations to the input images at each iteration. Extensive experiments on ImageNet show that the proposed attack method can generate adversarial examples that transfer much better to different networks than existing baselines. By evaluating our method against top defense solutions and official baselines from NIPS 2017 adversarial competition, the enhanced attack reaches an average success rate of 73.0%, which outperforms the top-1 attack submission in the NIPS competition by a large margin of 6.6%. We hope that our proposed attack strategy can serve as a strong benchmark baseline for evaluating the robustness of networks to adversaries and the effectiveness of different defense methods in the future.
更多查看译文
关键词
Deep Learning,Recognition: Detection,Categorization,Retrieval, Statistical Learning
AI 理解论文
溯源树
样例
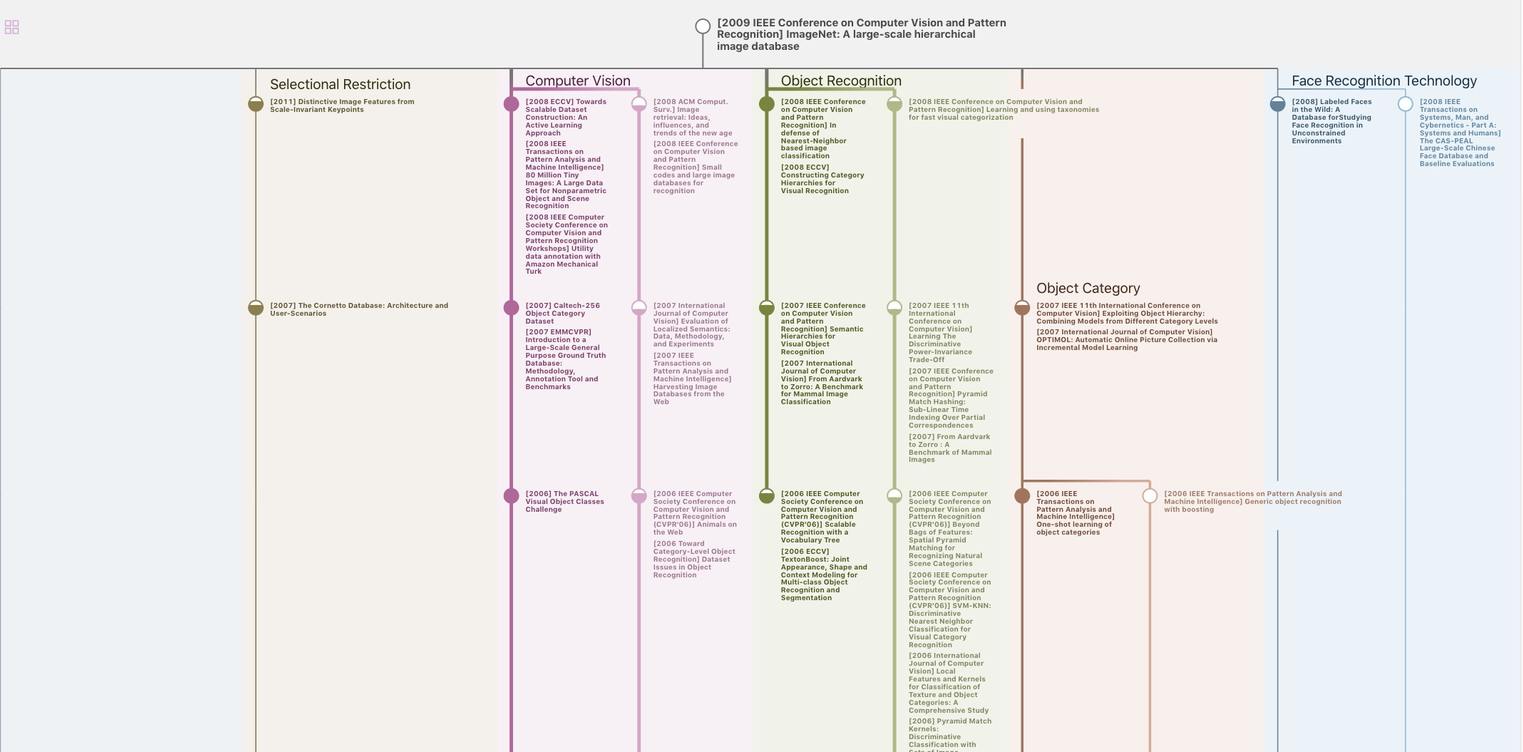
生成溯源树,研究论文发展脉络
Chat Paper
正在生成论文摘要