Function-refresh algorithms for determining the stored energy density of nonlinear elastic orthotropic materials directly from experimental data
International Journal of Non-Linear Mechanics(2018)
摘要
The typical approach to hyperelasticity is to assume an analytical expression of the stored energy function in terms of invariants and some constants, named “material parameters”. From available experimental data, a different data-driven approach is to establish specific function dependencies such that the stored energy can be uniquely determined in a numerical, deterministic way, exactly capturing smooth experimental data curves. In this approach the functions are not restricted to an analytical form and the model does not employ material parameters. Whereas the overall ideas regarding the search for a numerical solution employing proper interpolation functions are common, the actual computational procedure depends on the assumed function dependencies from available experimental data and known material symmetries. For the case of orthotropic materials, it is possible to assume a strain energy decomposition in terms of logarithmic strains which guarantees consistency with the infinitesimal theory at all deformation levels. At the same time, this energy can be determined with a minimum amount of tests. In previous works we introduced algorithms which use a generalization of the Kearsley–Zapas inversion formula for orthotropy. This approach lacks the desired generality to account for different reduced forms and possible tests available to the analyst. In this work we use a simpler approach using function-refresh algorithms, which allows us to obtain the stored energy density of orthotropic materials in more general cases.
更多查看译文
关键词
Soft materials,Data-driven hyperelasticity,Orthotropy
AI 理解论文
溯源树
样例
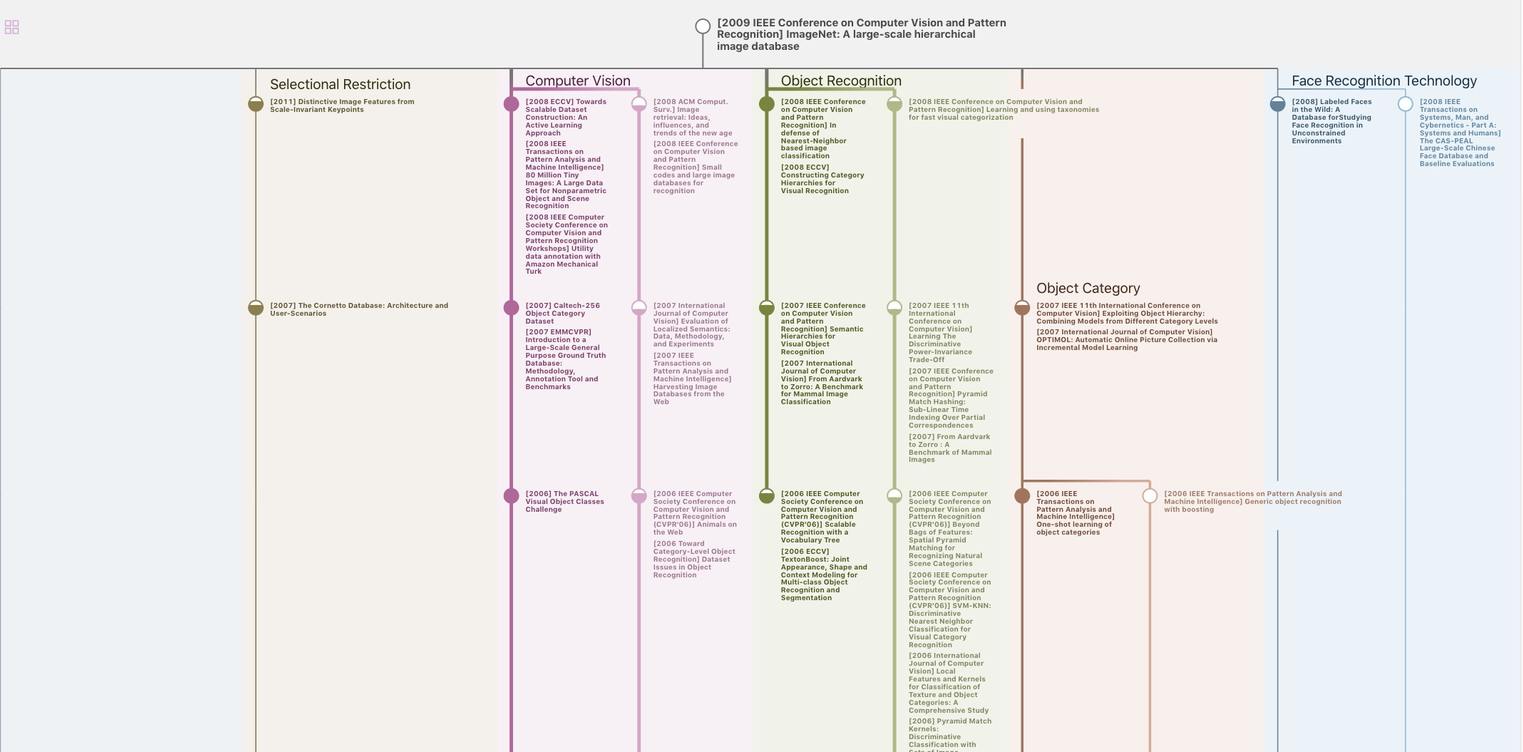
生成溯源树,研究论文发展脉络
Chat Paper
正在生成论文摘要