Multi-factored gene-gene proximity measures exploiting biological knowledge extracted from Gene Ontology : application in gene clustering.
IEEE/ACM transactions on computational biology and bioinformatics(2020)
摘要
To describe the cellular functions of proteins and genes, a potential dynamic vocabulary is Gene Ontology (GO), which comprises of three sub-ontologies namely, Biological-process, Cellular-component, and Molecular-function. It has several applications in the field of bioinformatics like annotating/measuring gene-gene or protein-protein semantic similarity, identifying genes/proteins by their GO annotations for disease gene and target discovery, etc. To determine semantic similarity between genes, several semantic measures have been proposed in literature, which involve information content of
GO-terms
, GO tree structure, or the combination of both. But, most of the existing semantic similarity measures do not consider different topological and information theoretic aspects of
GO-terms
collectively. Inspired by this fact, in this article, we have first proposed three novel semantic similarity/distance measures for genes covering different aspects of GO-tree. These are further implanted in the frameworks of well-known multi-objective and single-objective based clustering algorithms to determine functionally similar genes. For comparative analysis, 10 popular existing GO based semantic similarity/distance measures and tools are also considered. Experimental results on
Mouse genome
,
Yeast
, and
Human genome
datasets evidently demonstrate the supremacy of multi-objective clustering algorithms in association with proposed multi-factored similarity/distance measures. Clustering outcomes are further validated by conducting some biological/statistical significance tests. Supplementary information is available at
https://www.iitp.ac.in/sriparna/journals.html
.
更多查看译文
关键词
Semantics,Integrated circuits,Bioinformatics,Ontologies,Tools,Genomics,Current measurement
AI 理解论文
溯源树
样例
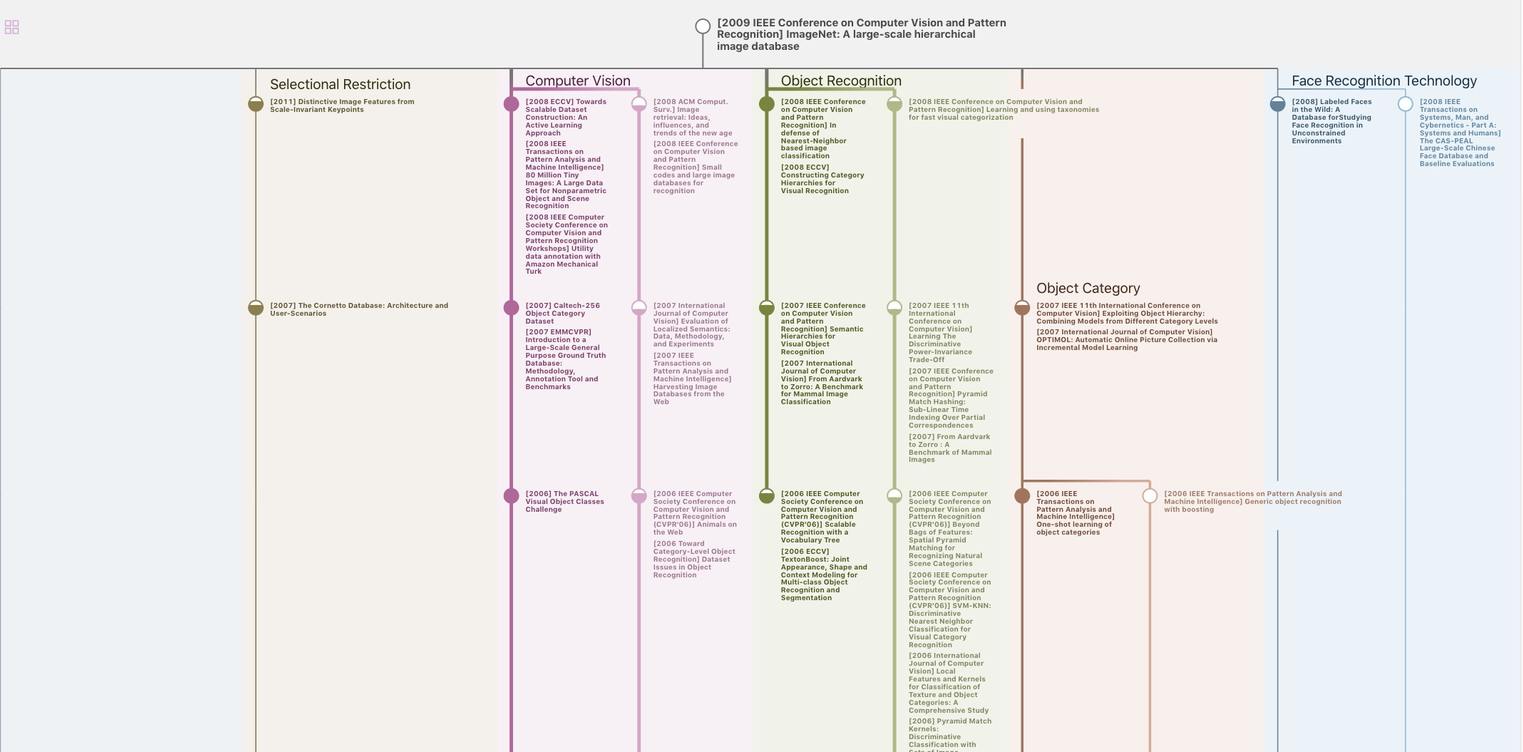
生成溯源树,研究论文发展脉络
Chat Paper
正在生成论文摘要