Sub-trajectory- and Trajectory-Neighbor-based Outlier Detection over Trajectory Streams.
ADVANCES IN KNOWLEDGE DISCOVERY AND DATA MINING, PAKDD 2018, PT I(2018)
摘要
Precisely and efficiently anomaly detection over trajectory streams is critical for many real-time applications. However, due to the uncertainty and complexity of behaviors of objects over trajectory streams, this problem has not been well solved. In this paper, we propose a novel detection algorithm, called STN-Outlier, for real time applications, where a set of fine-grained behavioral features are extracted from the sub-trajectory instead of point and a novel distance function is designed to measure the behavior similarity between two trajectories. Additionally, an optimized framework(TSX) is introduced to reduce the CPU resources cost of STN-Outlier. The performance experiments demonstrate that STN-Outlier successfully captures more fine-grained behaviors than the state-of-the-art methods; besides, the TSX framework outperforms the baseline solutions in terms of the CPU time in all cases.
更多查看译文
关键词
Outlier,Sub-trajectory,Trajectory streams
AI 理解论文
溯源树
样例
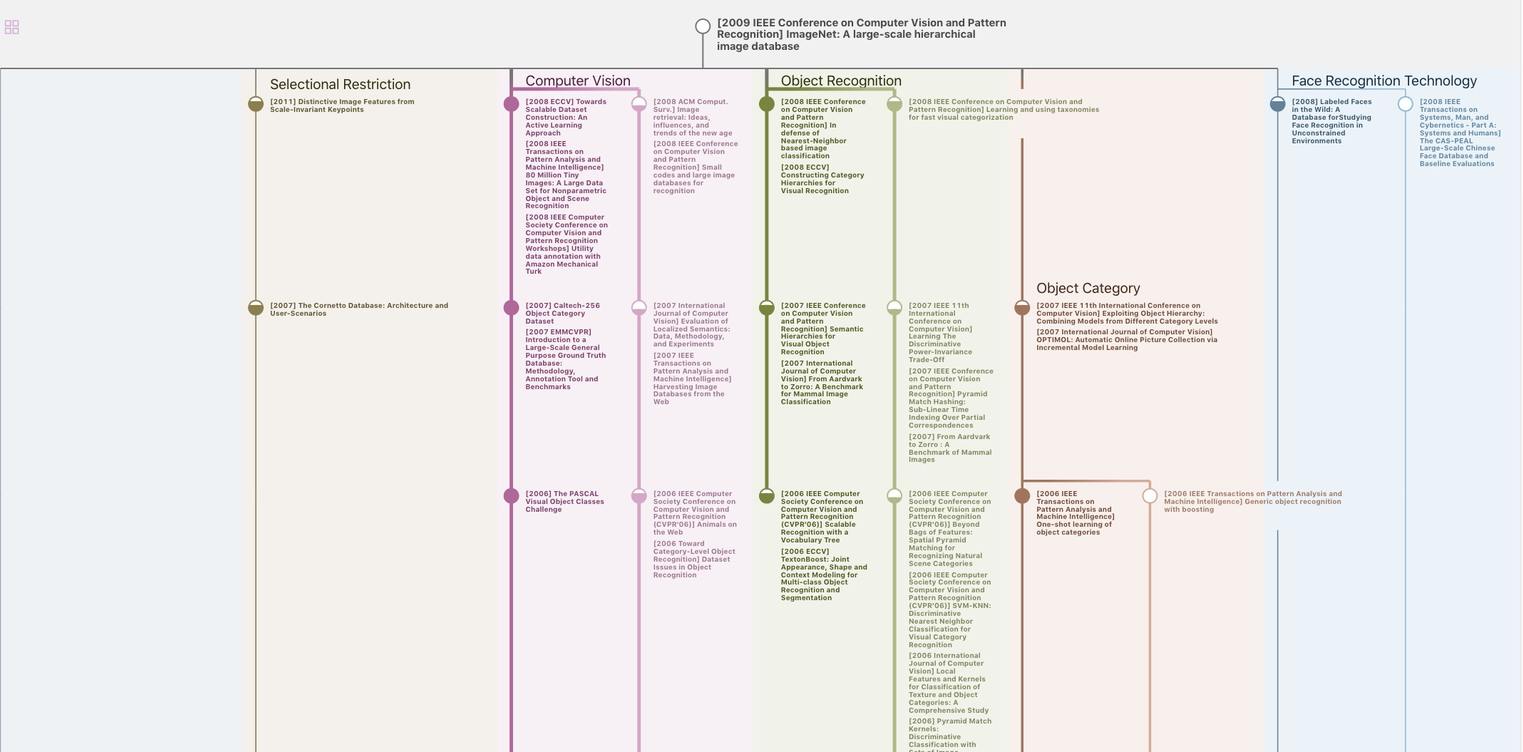
生成溯源树,研究论文发展脉络
Chat Paper
正在生成论文摘要