Augmented Feature-StAte Sensors in Human Activity Recognition
2017 9TH INTERNATIONAL CONFERENCE ON INFORMATION AND KNOWLEDGE TECHNOLOGY (IKT 2017)(2017)
摘要
Nowadays, Human Activity Recognition (HAR) has gain a lot of interest because of demand growth in many applications particularly in smart homes as a fundamental task. This problem is typically addressed as a supervised learning problem with the goal of learning the mapping of extracted related features out of sensors data to the underlying human activities. Most of the proposed methods for HAR do not consider important information such as time domain features explicitly for activity modeling. In this paper, Augmented Feature-StAte (Statistical-Activity context) Sensors (AFSSs) are proposed to incorporate combination of important statistical features and activity context information. To evaluate the proposed AFSSs, they are applied in four benchmark and popular probabilistic graphical activity recognition algorithms including Naive Bayesian classifiers (NBCs), Hidden Markov Models (HMMs), Hidden Semi Markov Models (HSMMs) and Linear-Chain Conditional Random Fields (LCCRFs). The experiments are performed on three well-known and real-world datasets in this field. The results show that the proposed AFSSs improve the classification performance particularly in terms of F1-Score, accuracy and robustness.
更多查看译文
关键词
Activity recognition,Statistical features,Activity context features,Augmented sensors,Probabilistic graphical models,Smart homes
AI 理解论文
溯源树
样例
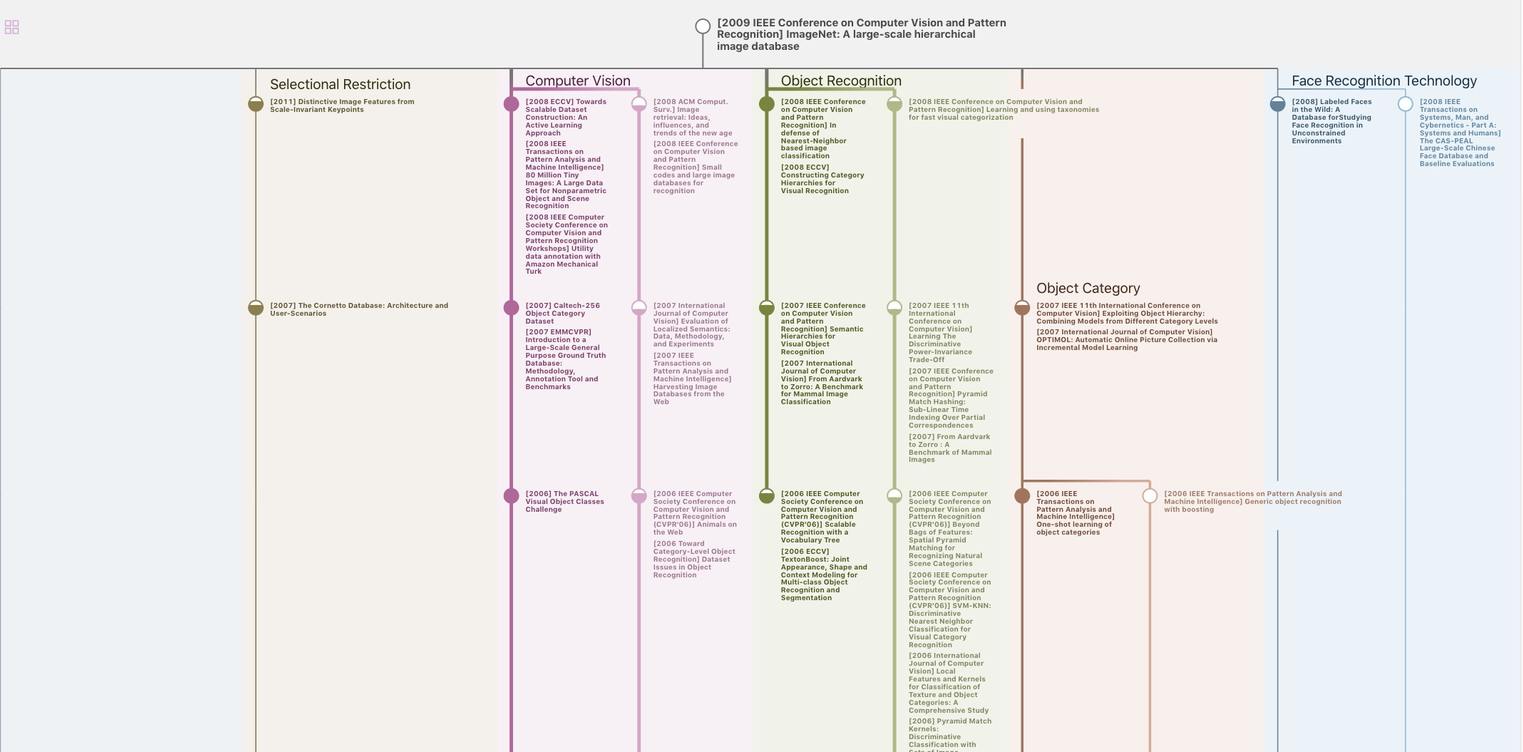
生成溯源树,研究论文发展脉络
Chat Paper
正在生成论文摘要