Identifying the genetic determinants of particular phenotypes in microbial genomes with very small training sets
bioRxiv(2019)
摘要
Background: Machine learning (ML) encompasses a large set of algorithms that aim at discovering complex patterns between elements within large data sets without any prior assumptions or modeling. However, some scientific disciplines still produce small data sets: in particular, empirical studies that try to link complex phenotypes such as virulence or drug resistance to individual sets of protein-coding genes (proteomes) typically have very small sample sizes. To date, it is unknown how ML performs in such cases. Results: To address this question, we evaluated the performance of adaptive boosting, a general ML classifier, on two data sets containing both the phenotype and the complete proteome of a small number of individuals. To assess the impact of proteome size, we contrasted a small genome (a virus: influenza) with a larger one (a bacterium: Pseudomonas). In order to analyze large proteomes, we developed a chunking algorithm. With the influenza data, we were able to rediscover amino acid sites experimentally implicated in three different complex phenotypes (infectivity, transmissibility, and pathogenicity). However, results for the much larger pseudomonas proteome, pertaining to three types of drug resistance (Ciprofloxacin, Ceftazidime, and Gentamicin), proved unstable, depended on a number of assumptions, and were not always biologically sensible. Conclusions: Our results show that ML algorithms such as adaptive boosting can be used to successfully identify the genetic determinants of microbes with small proteomes (viruses). Our chunking algorithm improved runtimes by an order of magnitude without sacrificing accuracy. Yet we found that the size of bacterial proteomes pushed ML to its limits in the face of small number of individuals. The use of these algorithms should probably be limited to preliminary or exploratory analysis, as long as both phenotyping and sequencing are too costly to perform on more individuals.
更多查看译文
关键词
influenza virus,<italic>Pseudomonas aeruginosa</italic>,machine learning,genome-wide association study,drug resistance
AI 理解论文
溯源树
样例
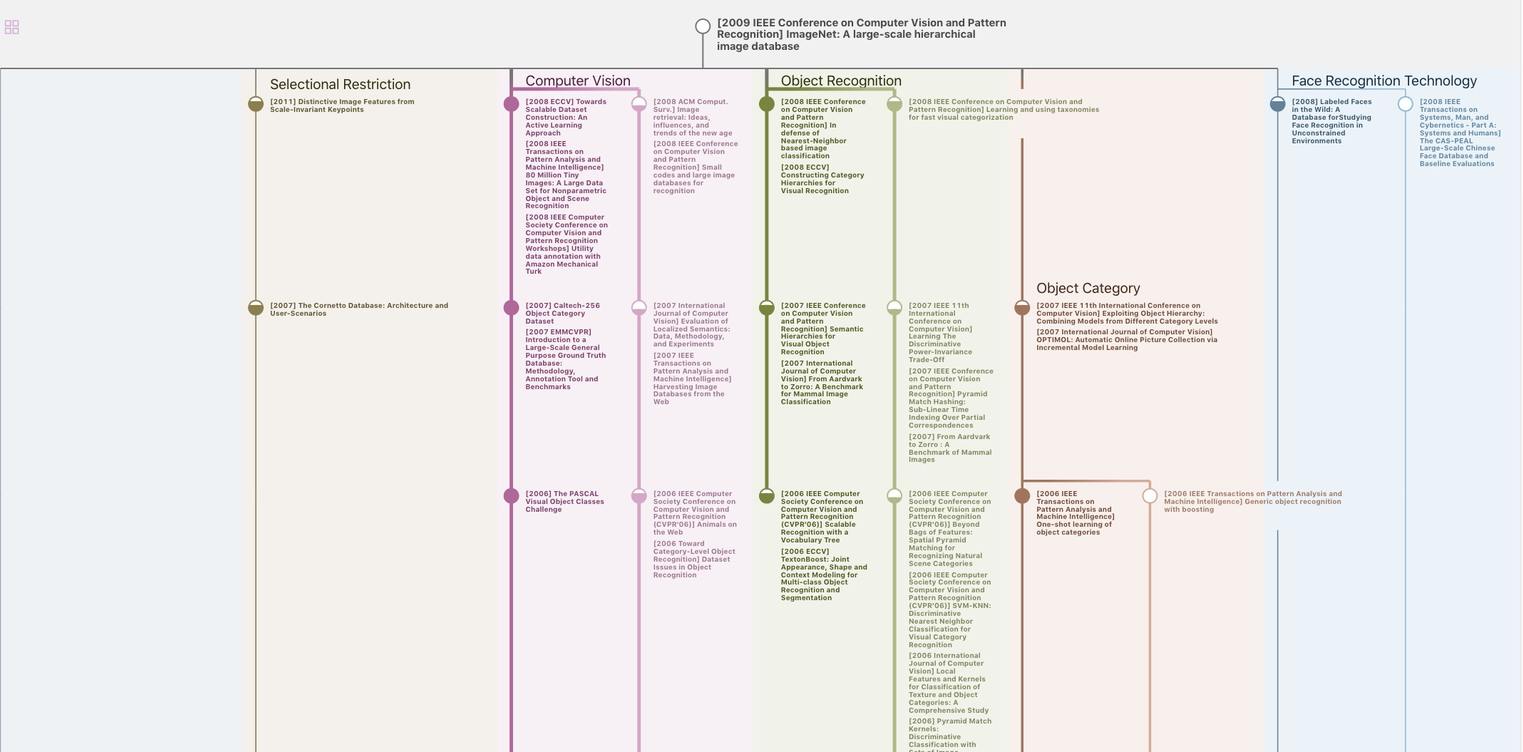
生成溯源树,研究论文发展脉络
Chat Paper
正在生成论文摘要