A Fraud Detection Decision Support System via Human On-Line Behavior Characterization and Machine Learning
2018 First International Conference on Artificial Intelligence for Industries (AI4I)(2018)
摘要
On-line and phone banking frauds are responsible for millions of dollars loss every year. In this work, we propose a Machine Learning-based Decision Support System to automatically associate a risk factor to each transaction performed through an on-line/mobile banking system. The proposed approach has a hierarchical architecture: First, an unsupervised Machine Learning module is used to detect abnormal patterns or wrongly labeled transactions; then, a supervised module provides a risk factor for the transactions that were not marked as anomalies in the previous step. Our solution exploits personal and historical information about the user, statistics that describe online traffic generated on the online/mobile banking system, and features extracted from motives of the transactions. The proposed approach deals with dataset unbalancing effectively. Moreover, it has been validated on a large database of transactions and on-line traffic provided by an industrial partner.
更多查看译文
关键词
Training,Feature extraction,Banking,Decision support systems,Radio frequency,Decision trees,Vegetation
AI 理解论文
溯源树
样例
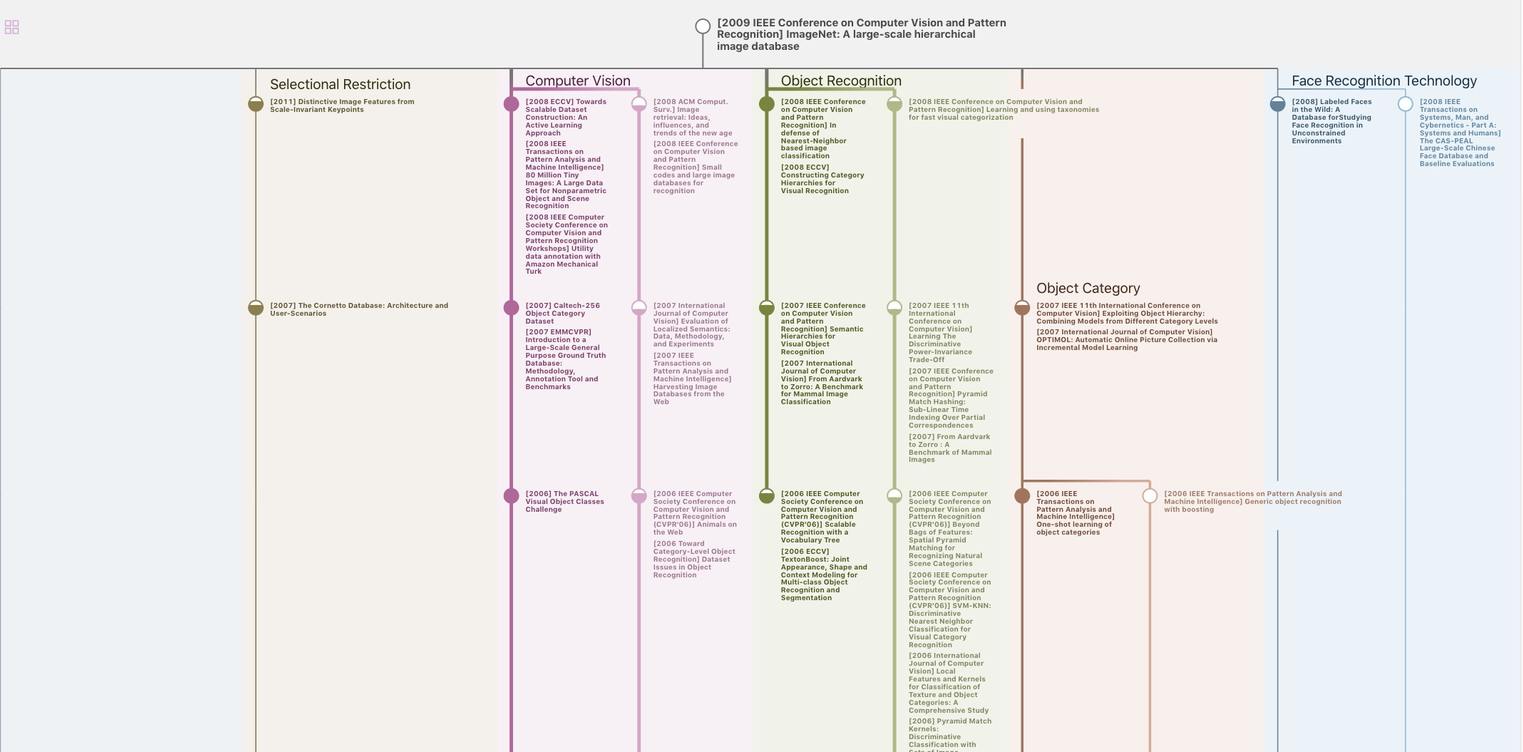
生成溯源树,研究论文发展脉络
Chat Paper
正在生成论文摘要