Latent goal models for dynamic strategic interaction.
PLOS COMPUTATIONAL BIOLOGY(2019)
摘要
Understanding the principles by which agents interact with both complex environments and each other is a key goal of decision neuroscience. However, most previous studies have used experimental paradigms in which choices are discrete (and few), play is static, and optimal solutions are known. Yet in natural environments, interactions between agents typically involve continuous action spaces, ongoing dynamics, and no known optimal solution. Here, we seek to bridge this divide by using a penalty shot task in which pairs of monkeys competed against each other in a competitive, real-time video game. We modeled monkeys' strategies as driven by stochastically evolving goals, onscreen positions that served as set points for a control model that produced observed joystick movements. We fit this goal-based dynamical system model using approximate Bayesian inference methods, using neural networks to parameterize players' goals as a dynamic mixture of Gaussian components. Our model is conceptually simple, constructed of interpretable components, and capable of generating synthetic data that capture the complexity of real player dynamics. We further characterized players' strategies using the number of change points on each trial. We found that this complexity varied more across sessions than within sessions, and that more complex strategies benefited offensive players but not defensive players. Together, our experimental paradigm and model offer a powerful combination of tools for the study of realistic social dynamics in the laboratory setting. Author summary Most studies of strategic decision making make use of simple tasks in which agents choose among only a limited number of distinct options. But real-world behavior is complex, requiring ongoing adjustment of strategies. Here, we propose a new model that is capable of reproducing the rich behavior of monkeys playing against each other in a dynamic decision task. Our model quantifies players' goals at each moment and offers a means of performing controlled experiments via simulation. This makes possible more realistic experimental paradigms for the study of strategic decision making and social interaction.
更多查看译文
AI 理解论文
溯源树
样例
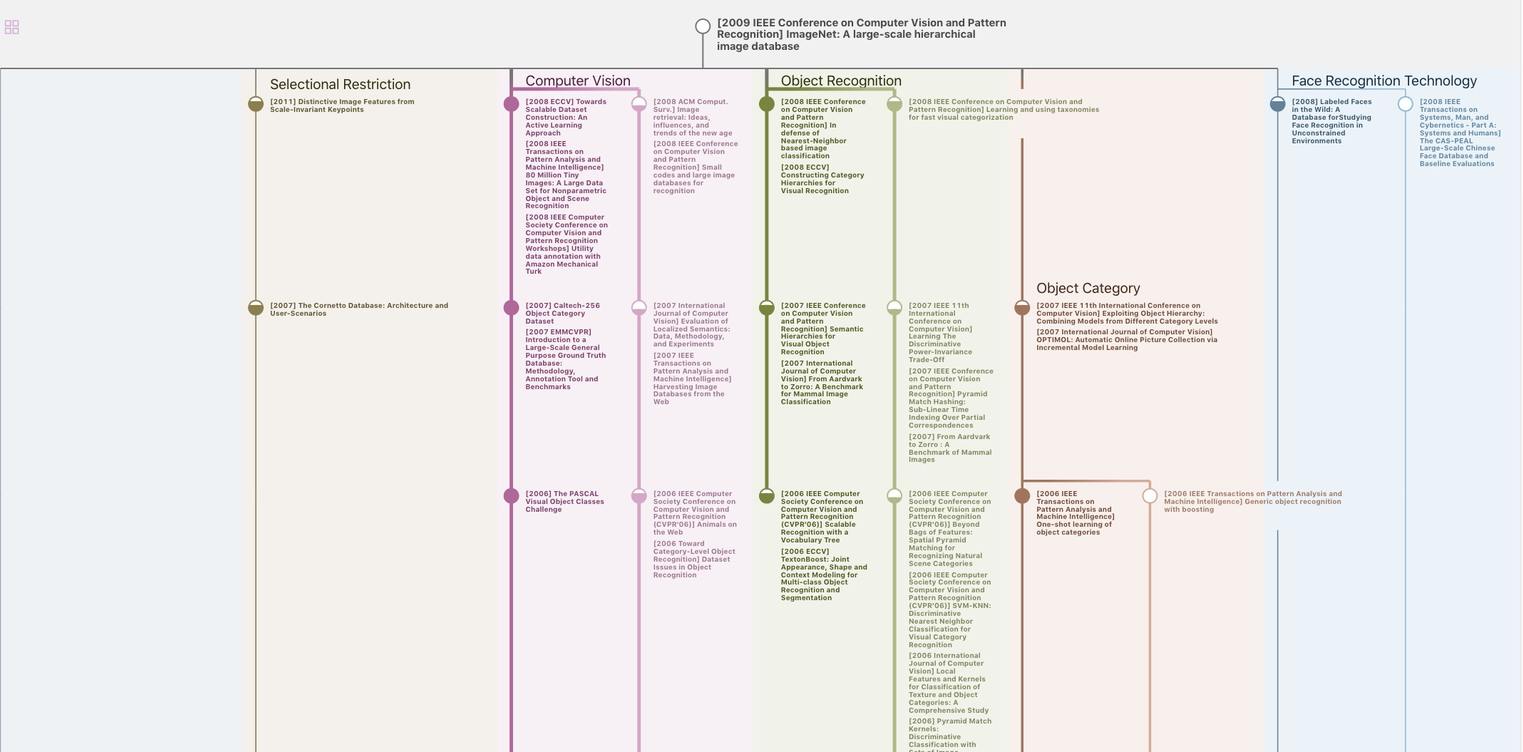
生成溯源树,研究论文发展脉络
Chat Paper
正在生成论文摘要