Bayesian Inference, Stochastic Simulation and Their Applications in Wireless Communication Systems
Journal of Communication and Information Systems(2016)
摘要
Bayesian inference has been successfully applied in fields as varied as anti-spam filtering, DNA sequencing, war codebreaking and election forecasting. Founded on the apparently simple Bayes’ theorem, which relates the previous distribution of a parameter with its distribution after evidence is collected, Bayesian tools allow for incorporating all existing knowledge about the phenomenon under study in order to improve parameter estimation. Because of the stochastic nature of the wireless channel, Bayesian inference is particularly well suited to the problem of symbol detection in many modern digital communication systems. When combined with Markov Chain Monte Carlo (MCMC) techniques, Bayesian receivers are capable of achieving minimum Bit Error Rate (BER) while avoiding the prohibitively high computational complexity associated with standard Maximum Likelihood (ML) or Maximum A Posteriori (MAP) estimators. In addition, such receivers are capable of numerically integrating out channel coefficients and noise variance, thus avoiding the need to use sub-optimal estimates of these parameters. This tutorial presents the rudiments of Bayesian statistics and MCMC in general, and discusses their applications in wireless communications in particular. The paper also details the design of Bayesian MCMC receiver in a system employing BPSK and ubject frequency-selective fading and Gaussian noise. Afterwards, recent advances in Bayesian receivers are surveyed for several important practical wireless transmission schemes, including MIMO, CDMA and OFDM. In addition, the paper addresses the application of Bayesian tools in challenging channel conditions — namely, nonlinear, non-Gaussian, underwater and fast fading channels.
更多查看译文
AI 理解论文
溯源树
样例
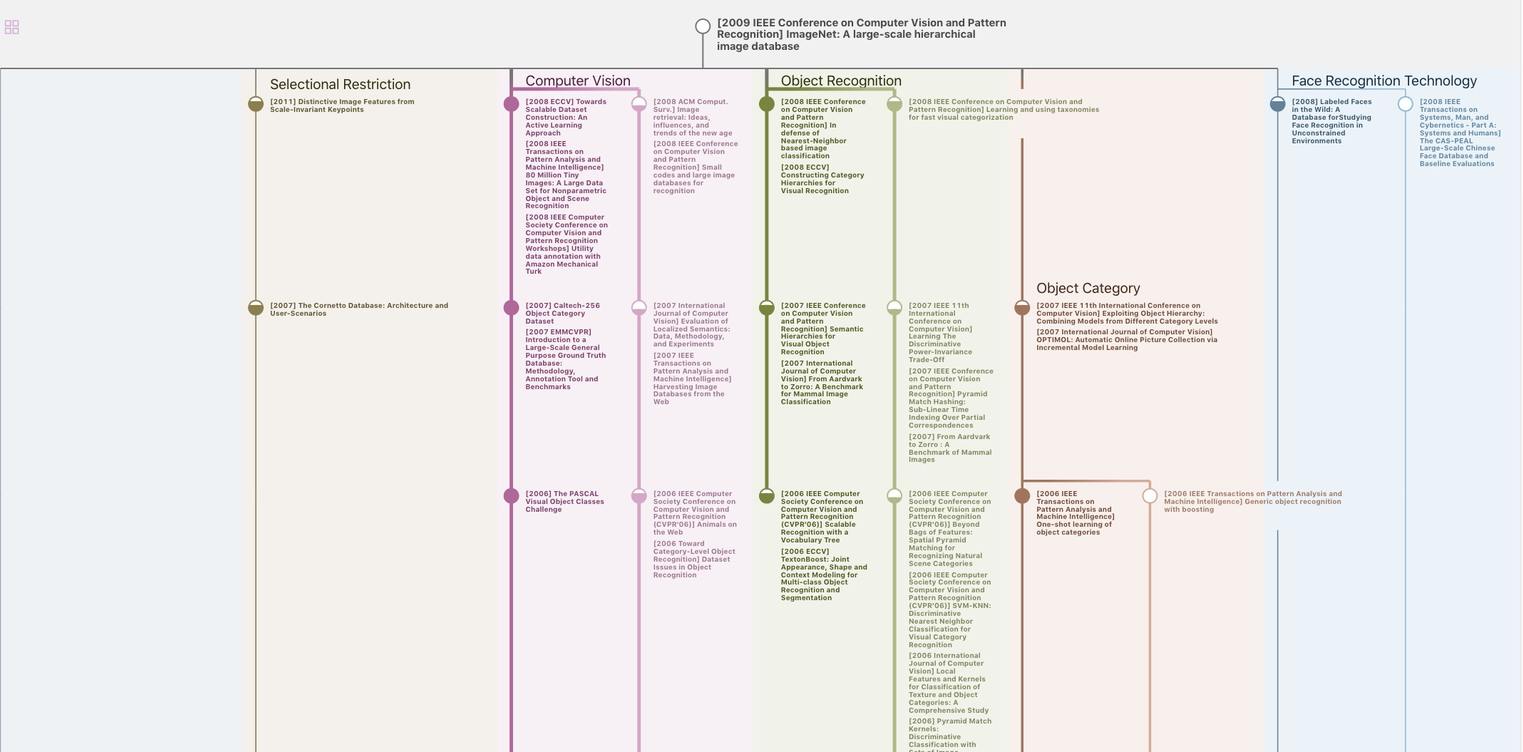
生成溯源树,研究论文发展脉络
Chat Paper
正在生成论文摘要