Addressing the Practical Limitations of Noisy-OR Using Conditional Inter-Causal Anti-Correlation with Ranked Nodes
IEEE Transactions on Knowledge and Data Engineering(2019)
摘要
Numerous methods have been proposed to simplify the problem of eliciting complex conditional probability tables in Bayesian networks. One of the most popular methods -“Noisy-OR”- approximates the required relationship in many real-world situations between a set of variables that are potential causes of an effect variable. However, the Noisy-OR function has the conditional inter-causal independence (CII) property which means that ‘explaining away’ behavior—one of the most powerful benefits of BN inference—is not present when the effect variable is observed as false. Hence, for many real-world problems where the Noisy-OR has been used or proposed, it may be deficient as an approximation of the required relationship. However, there is a very simple alternative solution, namely to define the variables as
ranked nodes
and to use the ranked node weighted average function. This does not have the CII property—instead, we prove it has the conditional anti-correlation property required to ensure that explaining away works in all cases. Moreover, ranked node variables are not restricted to binary states, and hence provide a more comprehensive and general solution to Noisy-OR in all cases.
更多查看译文
关键词
Noise measurement,Gaussian distribution,Covariance matrices,Security,Correlation,Bayes methods,Computer science
AI 理解论文
溯源树
样例
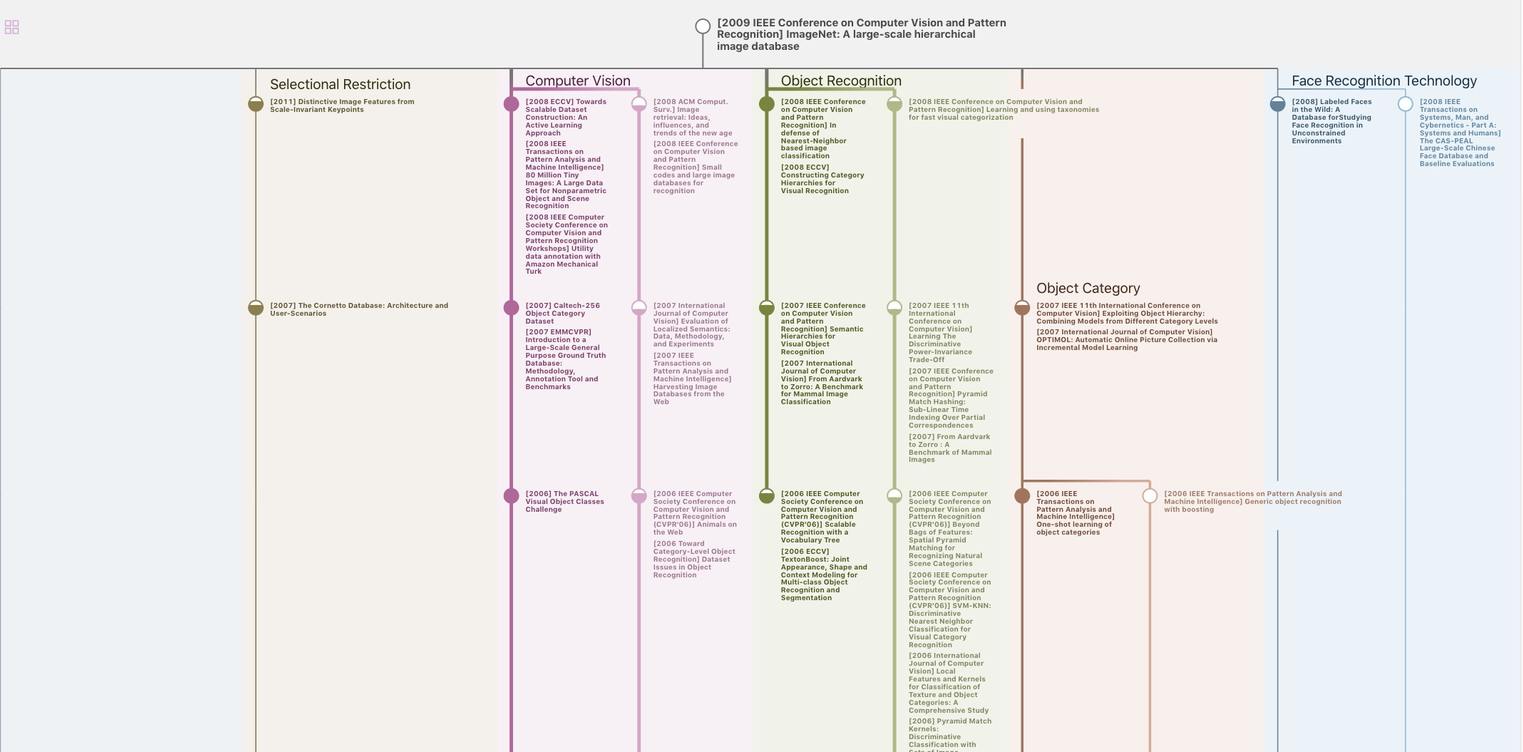
生成溯源树,研究论文发展脉络
Chat Paper
正在生成论文摘要