An integrated classifier for computer-aided diagnosis of colorectal polyps based on random forest and location index strategies.
Proceedings of SPIE(2016)
摘要
Feature classification plays an important role in differentiation or computer-aided diagnosis (CADx) of suspicious lesions. As a widely used ensemble learning algorithm for classification, random forest (RF) has a distinguished performance for CADx. Our recent study has shown that the location index (LI), which is derived from the well-known kNN (k nearest neighbor) and wkNN (weighted k nearest neighbor) classifier [1], has also a distinguished role in the classification for CADx. Therefore, in this paper, based on the property that the LI will achieve a very high accuracy, we design an algorithm to integrate the LI into RF for improved or higher value of AUC (area under the curve of receiver operating characteristics -- ROC). Experiments were performed by the use of a database of 153 lesions (polyps), including 116 neoplastic lesions and 37 hyperplastic lesions, with comparison to the existing classifiers of RF and wkNN, respectively. A noticeable gain by the proposed integrated classifier was quantified by the AUC measure.
更多查看译文
关键词
CADx,RF,location index,mixture classifier,AUC,ROC
AI 理解论文
溯源树
样例
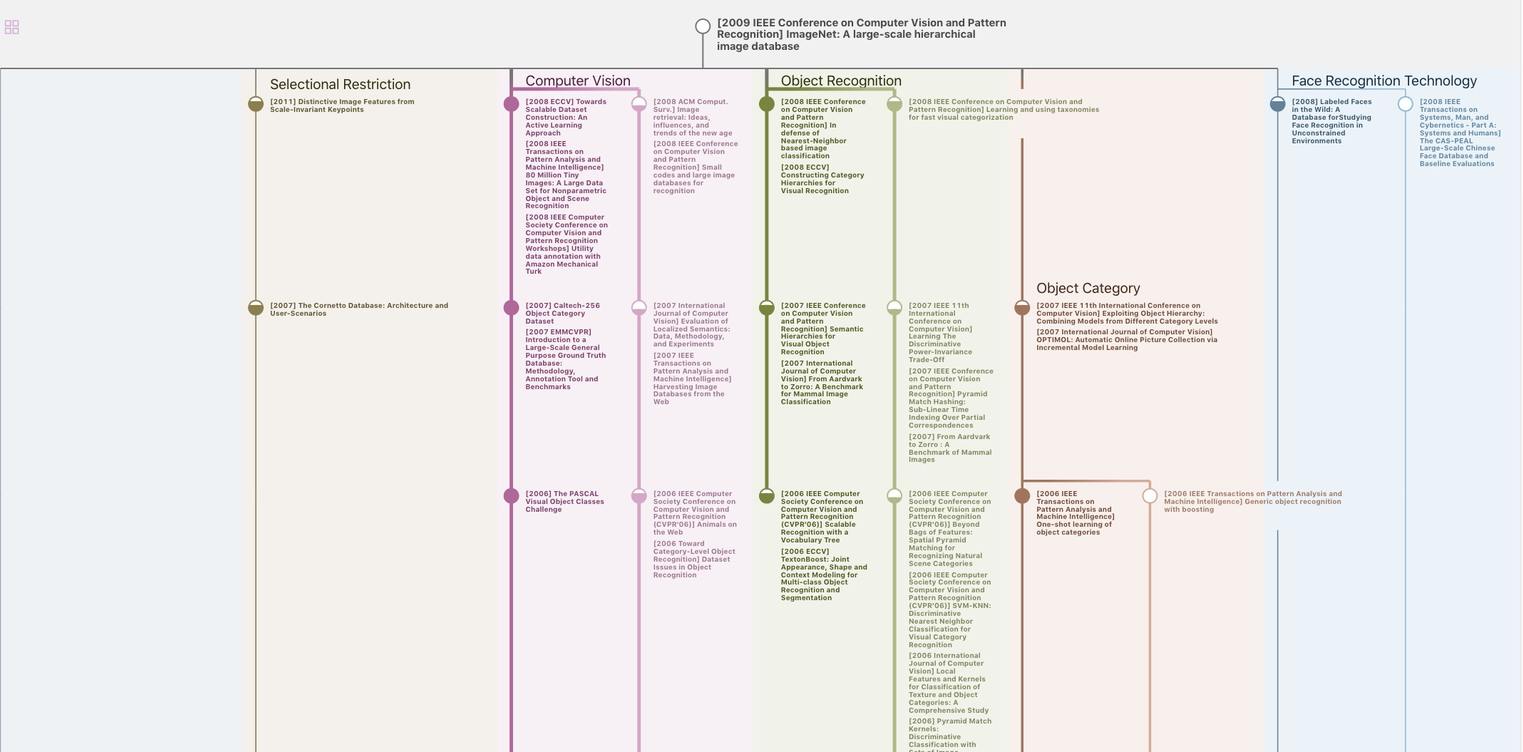
生成溯源树,研究论文发展脉络
Chat Paper
正在生成论文摘要