Joint Feature Classification for Wire Bond Joint Based on KPCA and Random Forest
2018 IEEE 20TH ELECTRONICS PACKAGING TECHNOLOGY CONFERENCE (EPTC)(2018)
摘要
We present a feasible algorithm to automatic identify and classify the quality of bonding joint in wire bonding via machine learning, named as KPCA (Kernel Principal Analysis) and Random Forest. The result can be acceptable in calculation time and accuracy, which is possible to use as feedback to control bonding parameters such as ultrasonic power and pressure, to strength the bonding reliability in production. First, the bonding joint images are mapped to a high dimension space, where KPCA is applied to decrease the image dimension for less calculation consumption and to eliminate high correlation features. The joint defect are then automatically identified and classified by Random Forest algorithm. Several strategies are adopted for improvement of accuracy. Our experiment result shows that the joint classification based on KPCA and Random Forest algorithm are better than conventional SVM and CNN algorithm on efficiency and accuracy.
更多查看译文
AI 理解论文
溯源树
样例
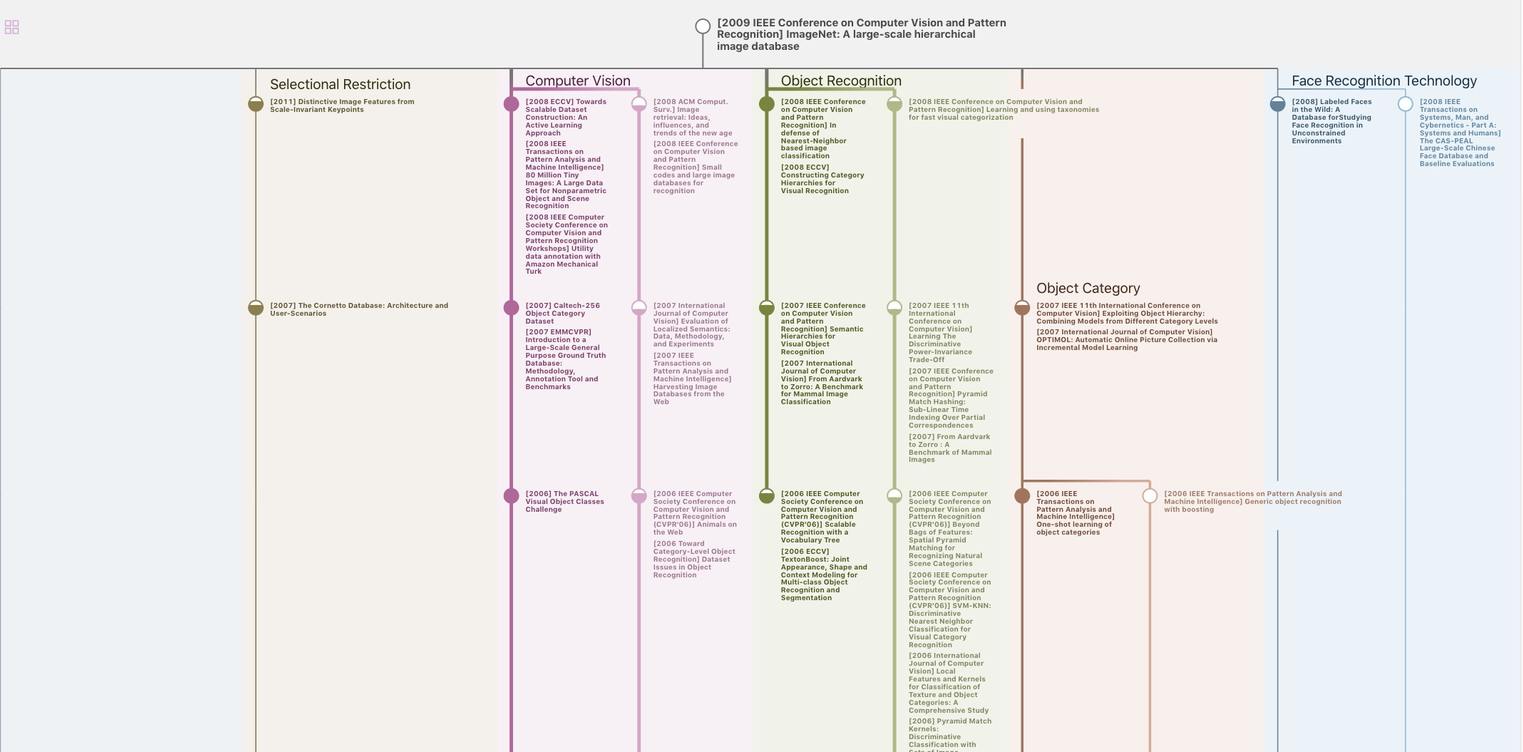
生成溯源树,研究论文发展脉络
Chat Paper
正在生成论文摘要