Patient-Aware EEG-Based Feature and Classifier Selection for e-Health Epileptic Seizure Prediction.
IEEE Global Communications Conference(2018)
摘要
With the advent of wireless EEG headsets and the improved computational capabilities of mobile devices, the ubiquitous monitoring of patients suffering from epilepsy has recently gained notable interest from research and industry under the umbrella of emerging e-health systems. Among others, the problem of seizure prediction plays a central role in reducing the adverse effects of epileptic seizures by trying to foresee the occurrence of an epileptic seizure before its onset. This paper presents a patient-aware seizure prediction approach which combines an optimized spatio-temporal EEG feature extraction algorithm and classifier selection to maximize the prediction accuracy and reduce the false alarm rate. Experimental results demonstrate that the proposed approach leads to promising prediction results when tested on real clinical data from the Freiburg database, with accuracy exceeding 90% for most subjects. The key differentiating aspect of the proposed approach is its flexibility to be efficiently fine-tuned and optimized per subject in order to enhance sensitivity and minimize the false alarm rate.
更多查看译文
关键词
classifier selection,e-health epileptic seizure prediction,wireless EEG headsets,mobile devices,e-health systems,patient-aware seizure prediction approach,false alarm rate,EEG feature extraction algorithm,patient monitoring,epilepsy,Freiburg database
AI 理解论文
溯源树
样例
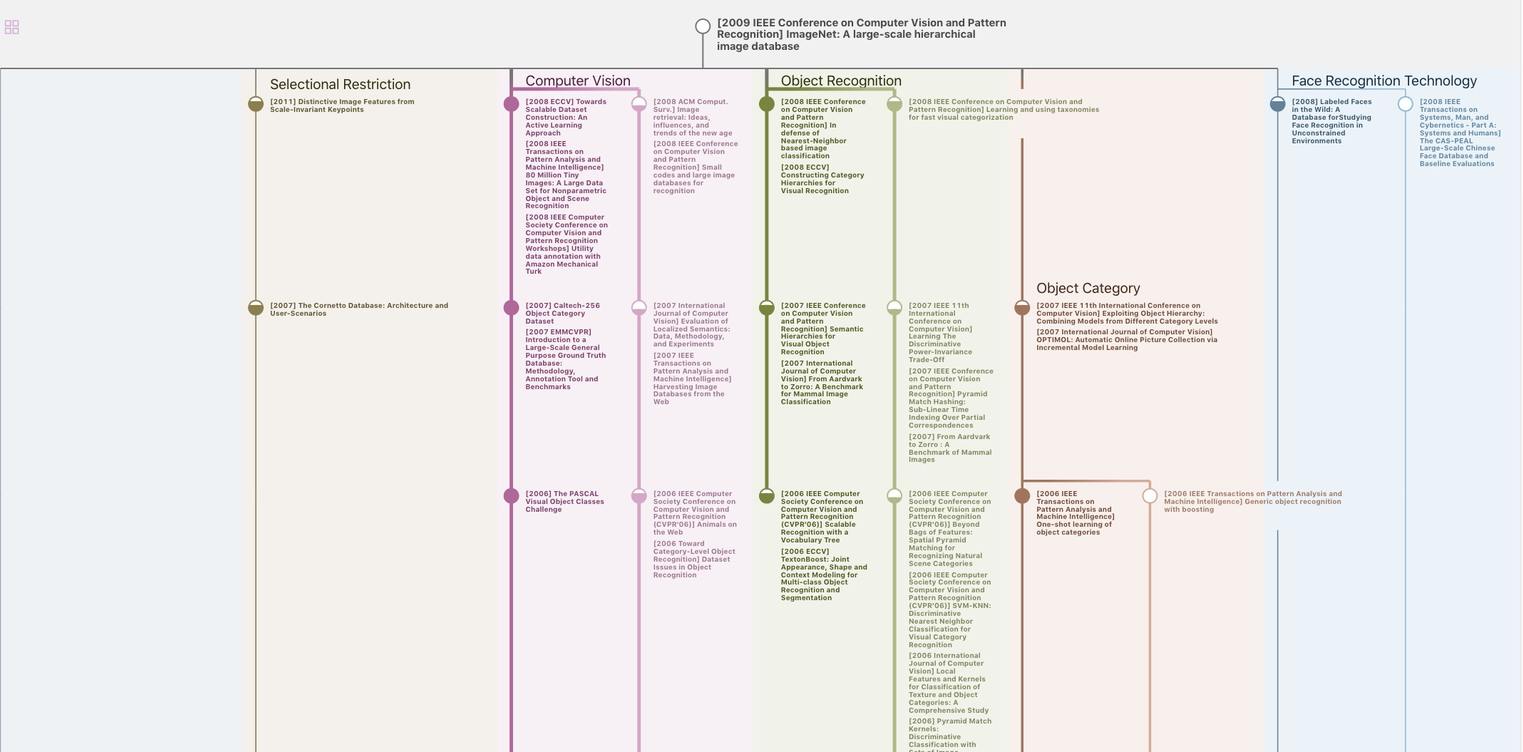
生成溯源树,研究论文发展脉络
Chat Paper
正在生成论文摘要