ECG derived feature combination versus single feature in predicting defibrillation success in out-of-hospital cardiac arrested patients
BIOMEDICAL PHYSICS & ENGINEERING EXPRESS(2019)
摘要
Objective: Algorithms to predict shock outcome based on ventricular fibrillation (VF) waveform features are potentially useful tool to optimize defibrillation strategy (immediate defibrillation versus cardiopulmonary resuscitation). Researchers have investigated numerous predictive features and classification methods using single VF feature and/or their combinations, however reported predictabilities are not consistent. The purpose of this study was to validate whether combining VF features can enhance the prediction accuracy in comparison to single feature. Approach: The analysis was performed in 3 stages: feature extraction, preprocessing and feature selection and classification. Twenty eight predictive features were calculated on 4s episode of the pre-shock VF signal. The preprocessing included instances normalization and oversampling. Seven machine learning algorithms were employed for selecting the best performin single feature and combination of features using wrapper method: Logistic Regression (LR), Naive-Bayes (NB), Decision tree (C4.5), AdaBoost. M1(AB), Support Vector Machine (SVM), Nearest Neighbour (NN) and Random Forest (RF). Evaluation of the algorithms was performed by nested 10 fold cross-validation procedure. Main results: A total of 251 unbalanced first shocks (195 unsuccessful and 56 successful) were oversampled to 195 instances in each class. Performance metric based on average accuracy of feature combination has shown that LR and NB exhibit no improvement, C4.5 and AB an improvement not greater than 1% and SVM, NN and RF an improvement greater than 5% in predicting defibrillation outcome in comparison to the best single feature. Significance: By performing wrapper method to select best performing feature combination the non-linear machine learning strategies (SVM, NN, RF) can improve defibrillation prediction performance.
更多查看译文
关键词
classification,ventricular fibrillation (VF),defibrillation,shock outcome,feature selection,machine learning algorithms
AI 理解论文
溯源树
样例
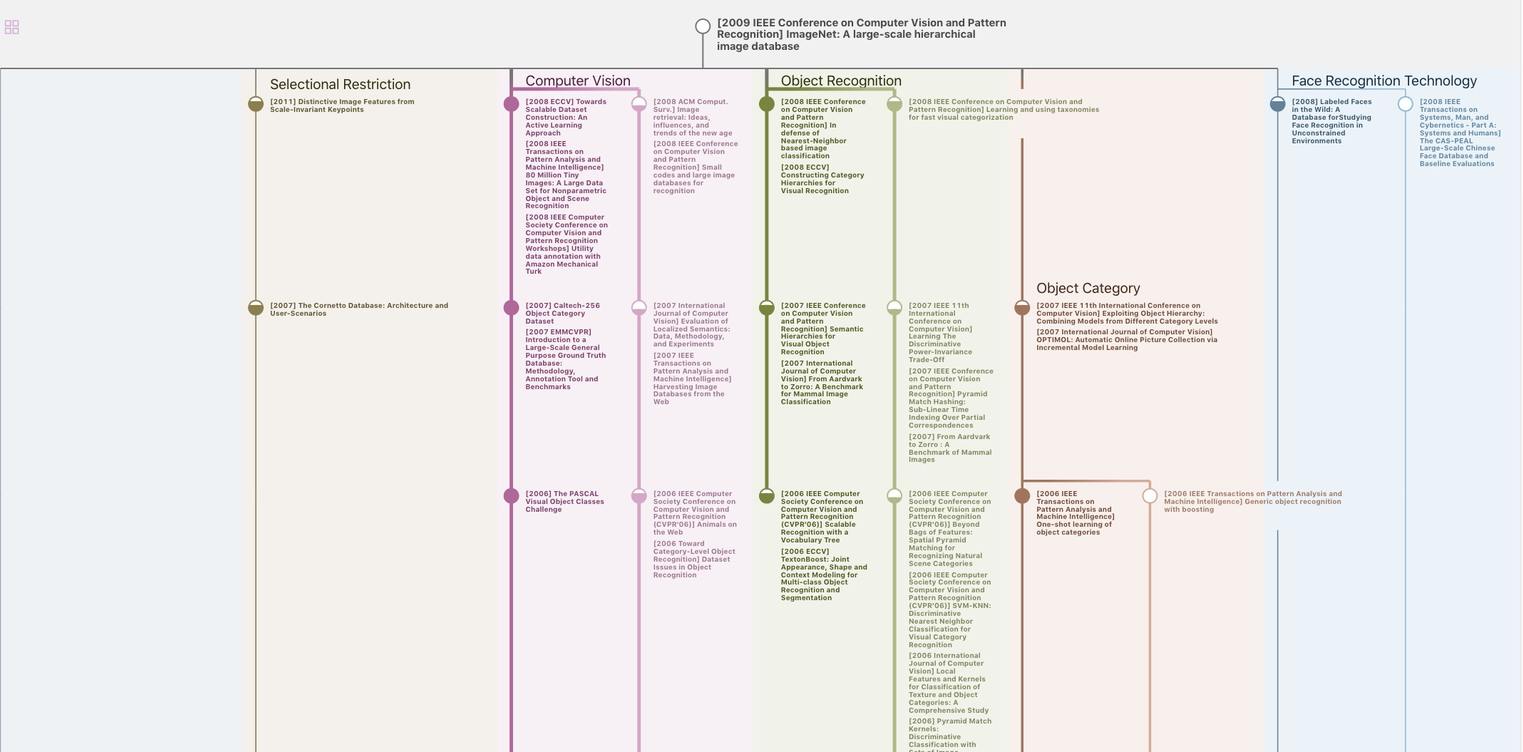
生成溯源树,研究论文发展脉络
Chat Paper
正在生成论文摘要