Secure mobile crowdsensing based on deep learning
China Communications(2018)
摘要
To improve the quality of multimedia services and stimulate secure sensing in Internet of Things applications, such as healthcare and traffic monitoring, mobile crowdsensing (MCS) systems must address security threats such as jamming, spoofing and faked sensing attacks during both sensing and information exchange processes in large-scale dynamic and heterogeneous networks. In this article, we investigate secure mobile crowdsensing and present ways to use deep learning (DL) methods, such as stacked autoencoder, deep neural networks, convolutional neural networks, and deep reinforcement learning, to improve approaches to MCS security, including authentication, privacy protection, faked sensing countermeasures, intrusion detection and anti-jamming transmissions in MCS. We discuss the performance gain of these DL-based approaches compared to traditional security schemes and identify the challenges that must be addressed to implement these approaches in practical MCS systems.
更多查看译文
关键词
Sensors,Servers,Authentication,Privacy,Feature extraction,Machine learning
AI 理解论文
溯源树
样例
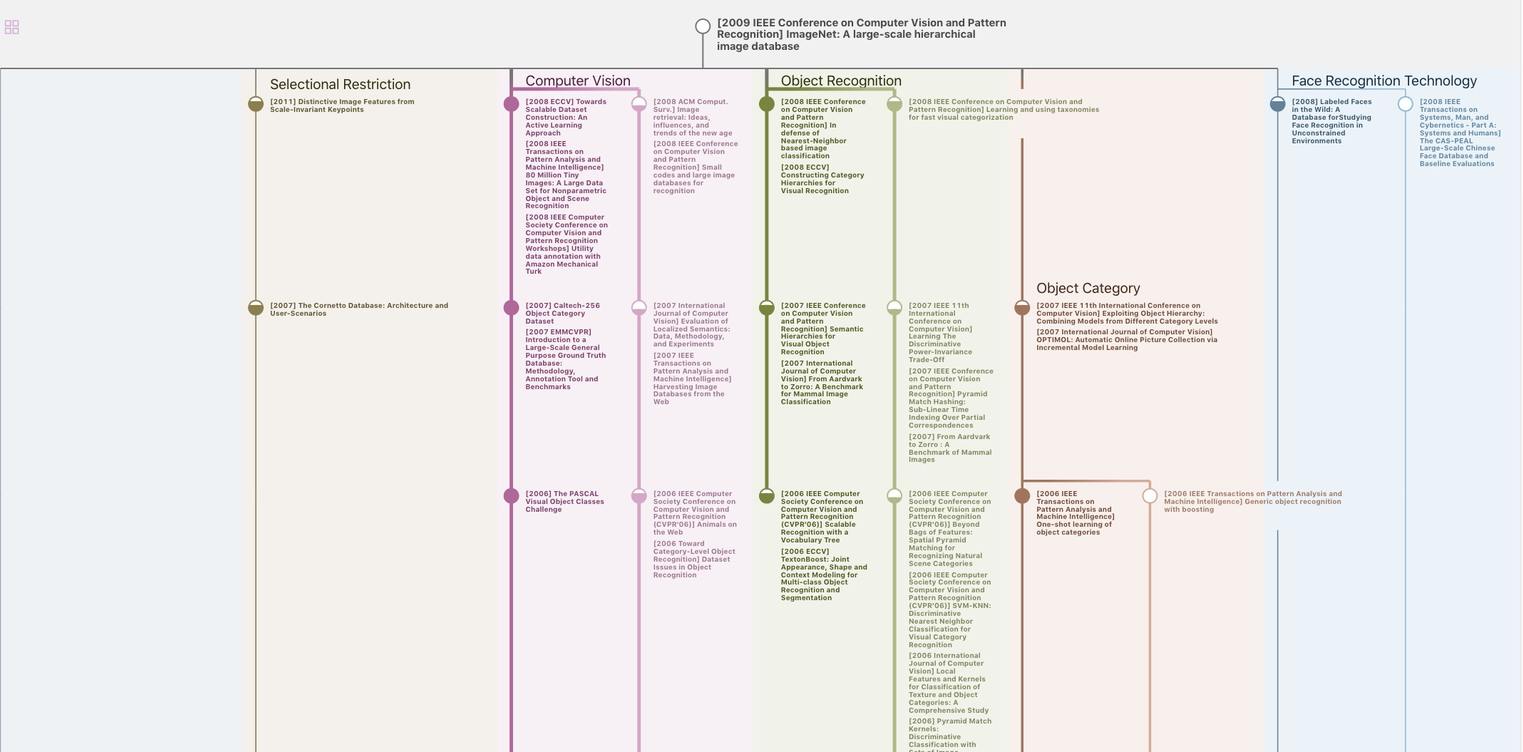
生成溯源树,研究论文发展脉络
Chat Paper
正在生成论文摘要