Online Quality-Aware Incentive Mechanism for Mobile Crowd Sensing with Extra Bonus
IEEE Transactions on Mobile Computing(2019)
摘要
Mobile crowd sensing is a new paradigm that enables smart mobile devices to collect and share various types of sensing data in urban environments. However, new challenges arise: one is how to evaluate the quality of data each mobile user potentially is capable of providing; another is how to allocate a satisfactory yet profitable amount of reward to mobile users in order to keep them participating in crowd sensing tasks. In this paper, we first introduce a mathematical model for characterizing quality of sensing data to be contributed by mobile users. Then, we present a utility function and formulate an optimization problem for the platform, who recruits participants to contribute sensing data, to maximize the amount of high quality sensing data under a limited task budget. We next present an effective and quality-aware incentive mechanism to solve this problem for online scenarios where participants may arrive or leave at any random time. Moreover, the proposed incentive mechanism allows the platform to provide selected participants with an extra bonus according to task completion level and their previous performance to motivate them further. We formally show the proposed mechanism has the desirable properties of truthfulness, individual rationality, budgetary feasibility, and computational efficiency. We compare the proposed scheme with existing methods via simulation using a real dataset. Extensive simulation results well justify the effectiveness and robustness of the proposed approach, e.g, compared with another online method “OMG”, the gap to the optimum for our proposed Online-QIM approach is reduce by 33.3 percent when budget $B = 1000$B=1000.
更多查看译文
关键词
Sensors,Task analysis,Data integrity,Mobile computing,Computer science,Mobile handsets,Mathematical model
AI 理解论文
溯源树
样例
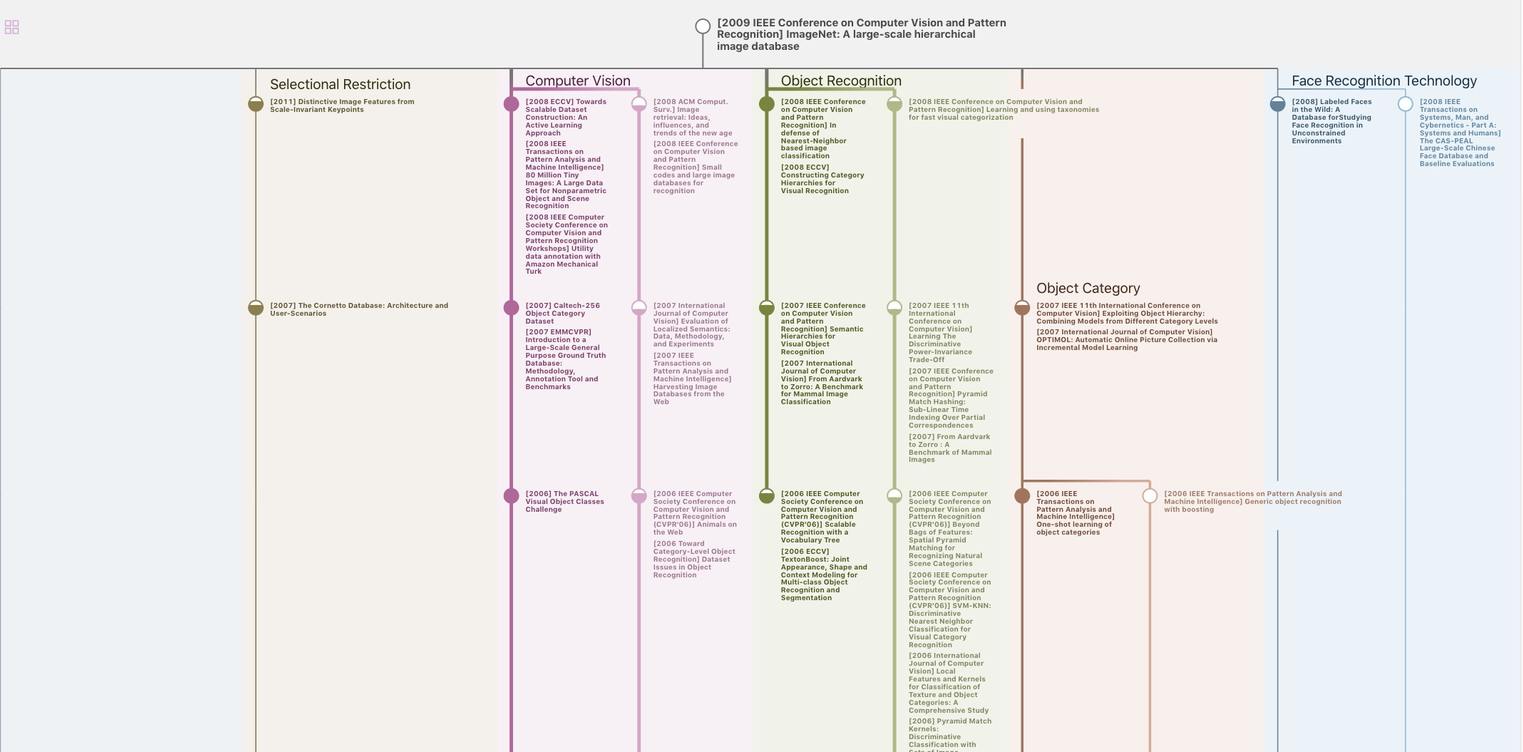
生成溯源树,研究论文发展脉络
Chat Paper
正在生成论文摘要