History matching of surfactant-polymer flooding using polynomial chaos expansion
JOURNAL OF PETROLEUM SCIENCE AND ENGINEERING(2019)
摘要
This paper proposes a robust framework for history matching which employs a sequential execution of sensitivity analysis, proxy modeling and inverse optimization to determine the optimized parameter space of model parameters. A mechanistic surfactant-polymer (SP) flood model is considered for history matching with an ultimate goal of accurately calibrating models that describe physical subprocesses of surfactant flooding, polymer flooding and displacement process. The employed model calibration algorithm starts with Sobol sensitivity analysis which reduces the large uncertain space of model parameters to determine the most important stochastic variables. The resulting low-dimensional parameter space is then represented via appropriate orthonormal basis of polynomial chaos expansion (PCE-proxy). An inverse optimization problem is then posed that minimizes the miss-fit between PCE-proxy response and experimental observations by employing a Genetic Algorithm. Finally, the epistemic uncertainty in PCE-proxy is quantified by combining it with a Gaussian regression process called Kriging. We use this framework to calibrate the SP flood model by history matching a single coreflood experiment for quantities of interest such as pressure drop profile and cumulative oil recovery curve. We then show that the calibrated model is successfully able to predict all our quantities of interest for two other coreflood experiments without any ad-hoc tuning of parameters. The proposed proxy-accelerated inverse optimization framework shows significant promise for model calibration or to improve the quality of history matched results.
更多查看译文
关键词
Surfactant-polymer flooding model,History matching,Calibration,Polynomial chaos expansion,Genetic algorithm,Kriging
AI 理解论文
溯源树
样例
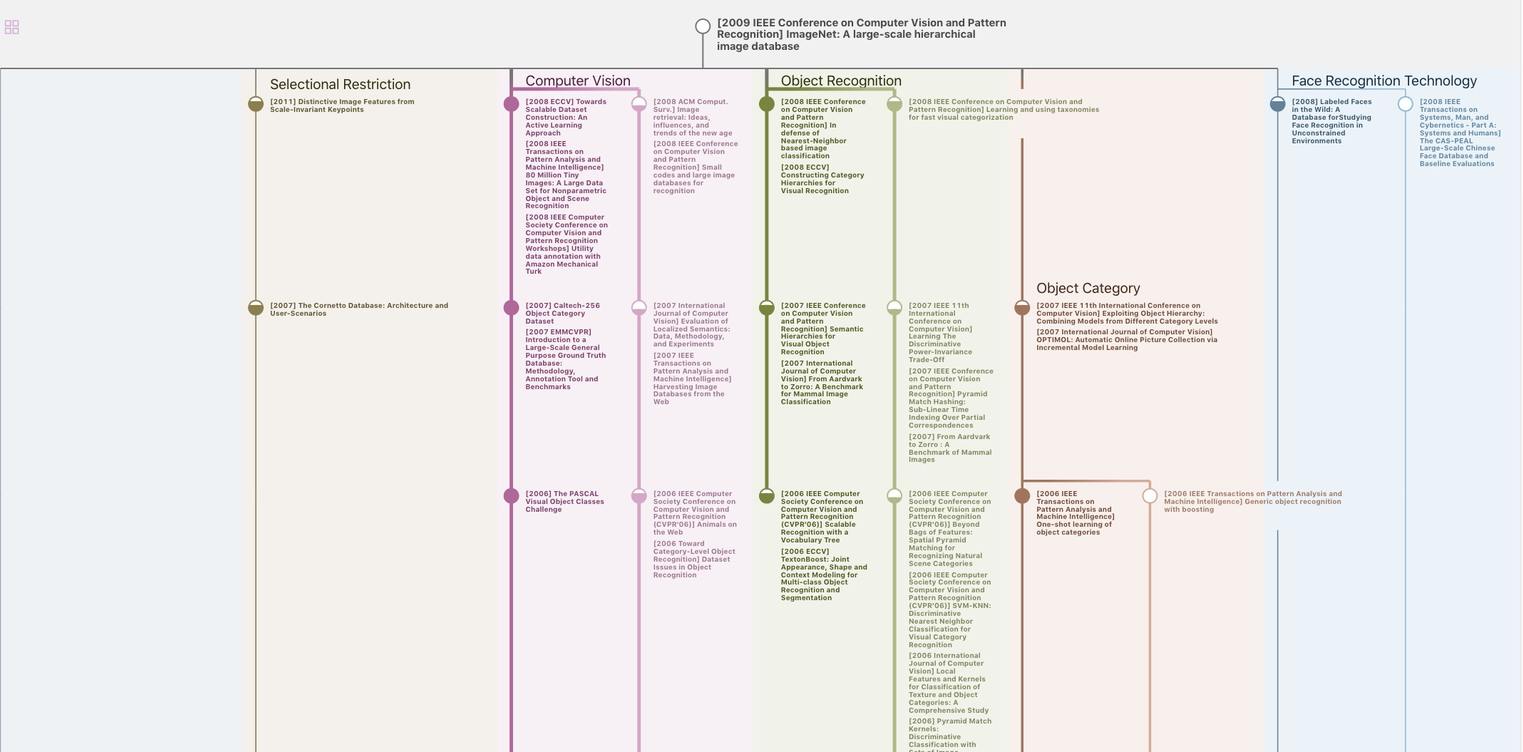
生成溯源树,研究论文发展脉络
Chat Paper
正在生成论文摘要