Calibrated Data Simplification for Energy-efficient Location Sensing in Internet of Things
IEEE Internet of Things Journal(2019)
摘要
The Internet of Things (IoT) has gradually changed the way of people’s lives due to its ability of connecting everything together, and meanwhile the accurate location sensing plays a crucial role in achieving this goal. Up to now, as one of the most representative outdoor localization systems, the global positioning system has been widely used, but its performance may be dramatically declined in indoor environment due to the serious multipath effect and signal attenuation caused by the complicated indoor structure. At the same time, the location fingerprint-based localization approach has become a popular one in indoor environment, and meanwhile the corresponding calibrated signal simplification in location database construction has been primarily considered due to its significant practical meaning in avoiding the blind signal sampling. In this paper, we propose to use an information-theoretic lens to construct the energy-efficient location fingerprint database for the localization in IoT. Interestingly, by analyzing the information loss in signal sampling, we analogize the database construction process into the information propagation process in a lossy channel, and then formulate the relations of sample capacity and localization error from an information-theoretic view. After that, by selecting an appropriate time interval to sample the independent and nonredundant signal, the minimum number of sampled signal under the given expected localization accuracy is determined. Finally, the extensive experimental results show that compared with the state-of-the-art approaches, the proposed one can effectively simplify the calibrated data for the energy-efficient location database construction in different wireless localization networks.
更多查看译文
关键词
Databases,Internet of Things,Sensors,Propagation losses,Indoor environments,Lenses,Calibration
AI 理解论文
溯源树
样例
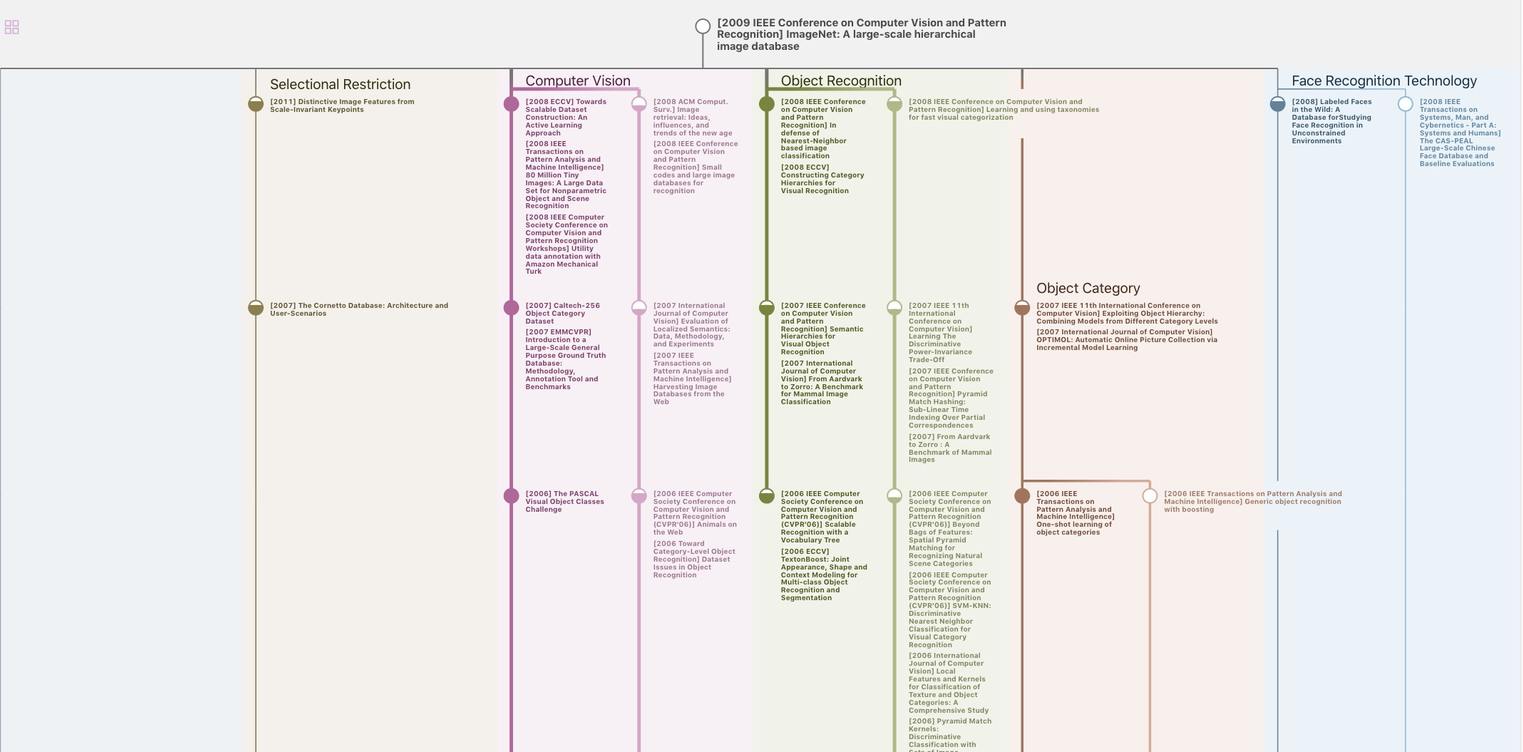
生成溯源树,研究论文发展脉络
Chat Paper
正在生成论文摘要