Probabilistic short term wind power forecasts using deep neural networks with discrete target classes
Advances in Geosciences(2018)
摘要
Abstract. Usually, neural networks trained on historical feed-in time seriesof wind turbines deterministically predict power output over the next hoursto days. Here, the training goal is to minimise a scalar cost function, oftenthe root mean square error (RMSE) between network output and target values.Yet similar to the analog ensemble (AnEn) method, the training algorithm canalso be adapted to analyse the uncertainty of the power output from thespread of possible targets found in the historical data for a certainmeteorological situation. In this study, the uncertainty estimate is achievedby discretising the continuous time series of power targets into several bins(classes). For each forecast horizon, a neural network then predicts theprobability of power output falling into each of the bins, resulting in anempirical probability distribution. Similiar to the AnEn method, the proposedmethod avoids the use of costly numerical weather prediction (NWP) ensembleruns, although a selection of several deterministic NWP forecasts as input ishelpful. Using state-of-the-art deep learning technology, we applied ourmethod to a large region and a single wind farm. MAE scores of the50-percentile were on par with or better than comparable deterministicforecasts. The corresponding Continuous Ranked Probability Score (CRPS) waseven lower. Future work will investigate the overdispersiveness sometimesobserved, and extend the method to solar power forecasts.
更多查看译文
AI 理解论文
溯源树
样例
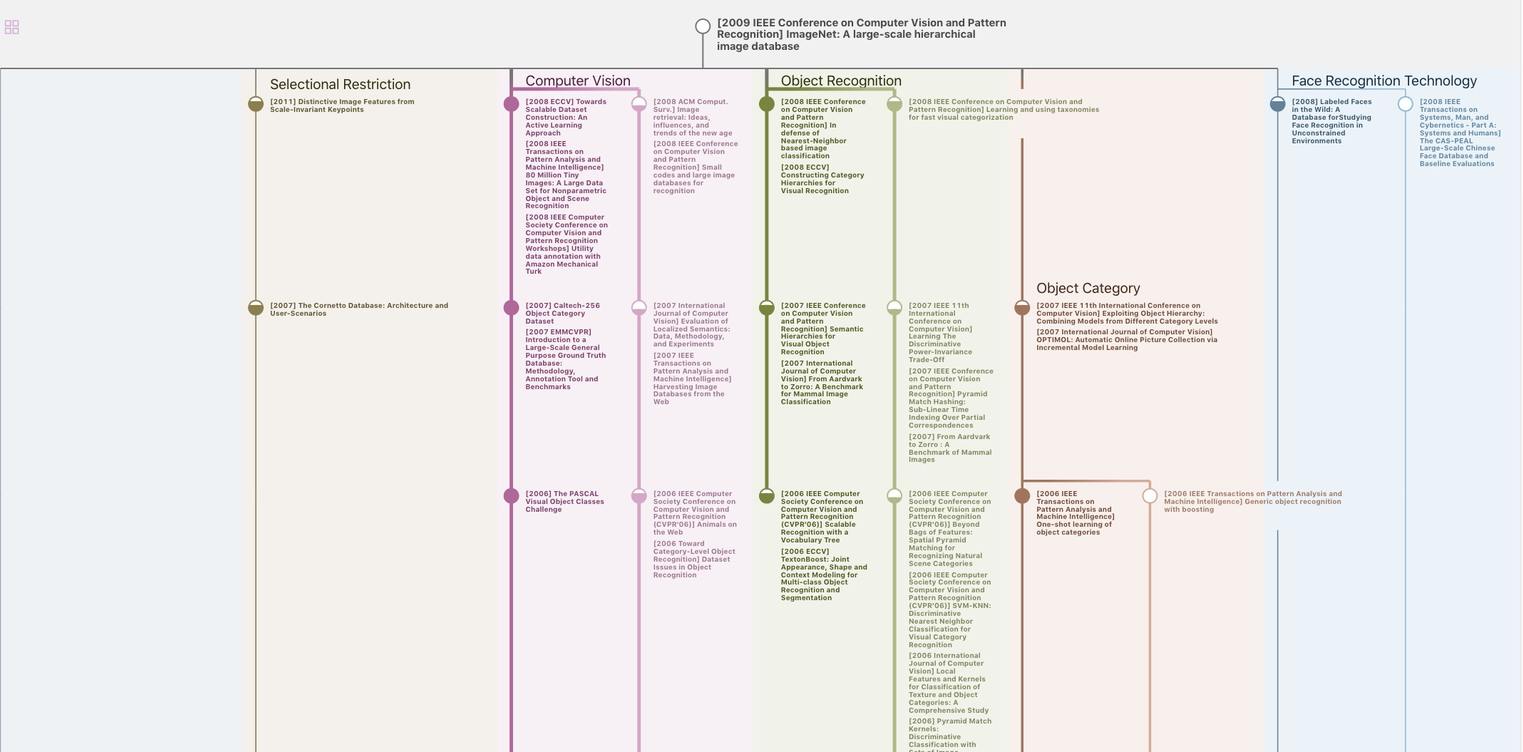
生成溯源树,研究论文发展脉络
Chat Paper
正在生成论文摘要