Certified dimension reduction in nonlinear Bayesian inverse problems.
arXiv: Probability(2018)
摘要
We propose a dimension reduction technique for Bayesian inverse problems with nonlinear forward operators, non-Gaussian priors, and non-Gaussian observation noise. The likelihood function is approximated by a ridge function, i.e., a map which depends non-trivially only on a few linear combinations of the parameters. We build this ridge approximation by minimizing an upper bound on the Kullback--Leibler divergence between the posterior distribution and its approximation. This bound, obtained via logarithmic Sobolev inequalities, allows one to certify the error of the posterior approximation. Computing the bound requires computing the second moment matrix of the gradient of the log-likelihood function. In practice, a sample-based approximation of the upper bound is then required. We provide an analysis that enables control of the posterior approximation error due to this sampling. Numerical and theoretical comparisons with existing methods illustrate the benefits of the proposed methodology.
更多查看译文
关键词
nonlinear bayesian inverse problems,dimension reduction
AI 理解论文
溯源树
样例
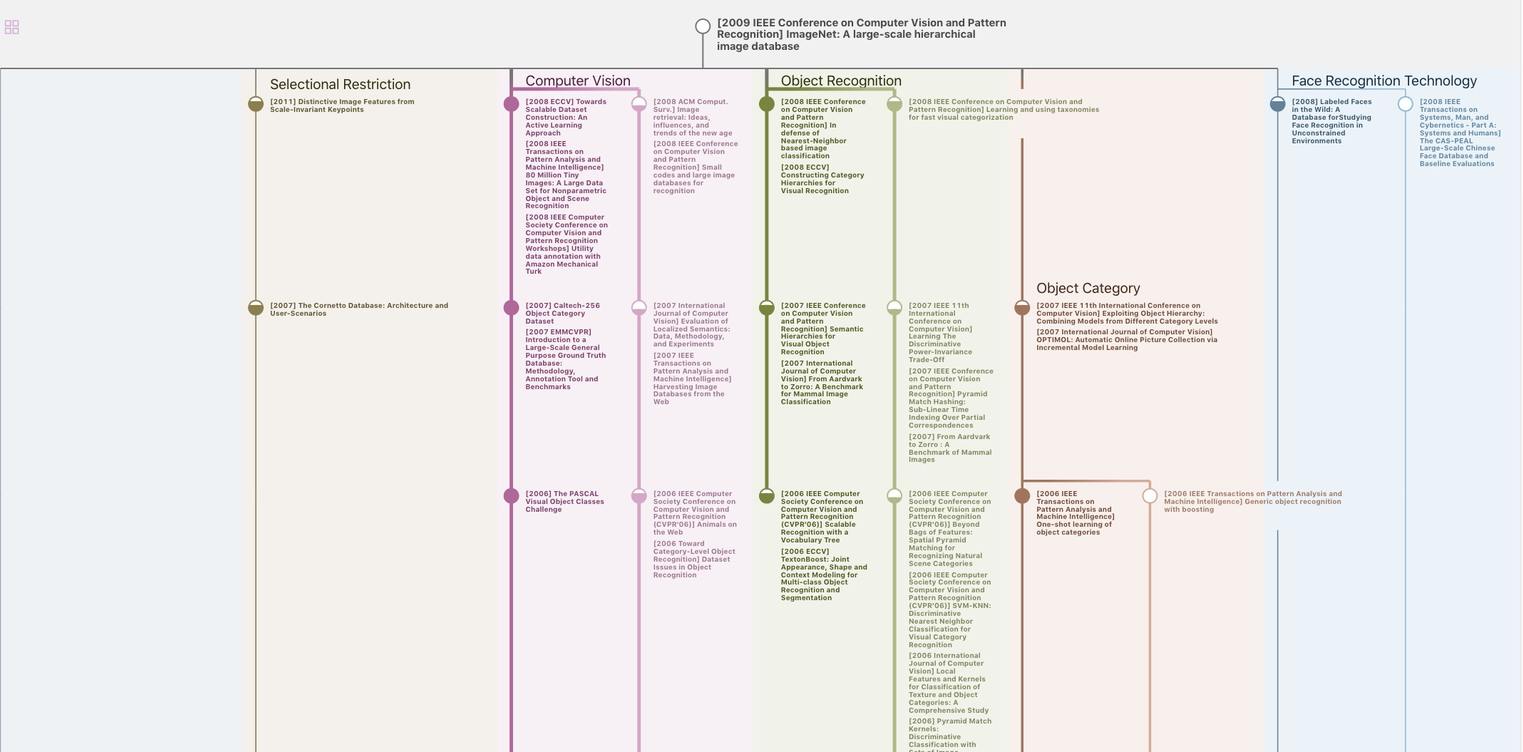
生成溯源树,研究论文发展脉络
Chat Paper
正在生成论文摘要