Genome Functional Annotation using Deep Convolutional Neural Networks
bioRxiv(2019)
摘要
Deep neural network application is today a skyrocketing field in almost all disciplinary domains. In genomics, which deals with DNA sequences, the development of deep neural networks is expected to revolutionize current practice, from fundamental issues such as understanding the evolution of genomes to more specific applications such as the development of personalized medicine. Several approaches have been developed relying on convolution neural networks (CNN) to identify the functional role of sequences such as promoters, enhancers or protein binding sites along genomes. These approaches rely on the generation of sequences batches with known annotations for learning purpose. While they show good performance to predict annotations from a test subset of these batches, they usually work less well when applied genome-wide; i.e. for whole genome annotation. In this paper, we address this issue and propose an optimal strategy to train CNN for this specific application. We use as a case study gene Transcription Start Sites (TSS) and show that a model trained on one organism (e.g. human) can be used to predict TSS in a different species (e.g. mouse).
更多查看译文
AI 理解论文
溯源树
样例
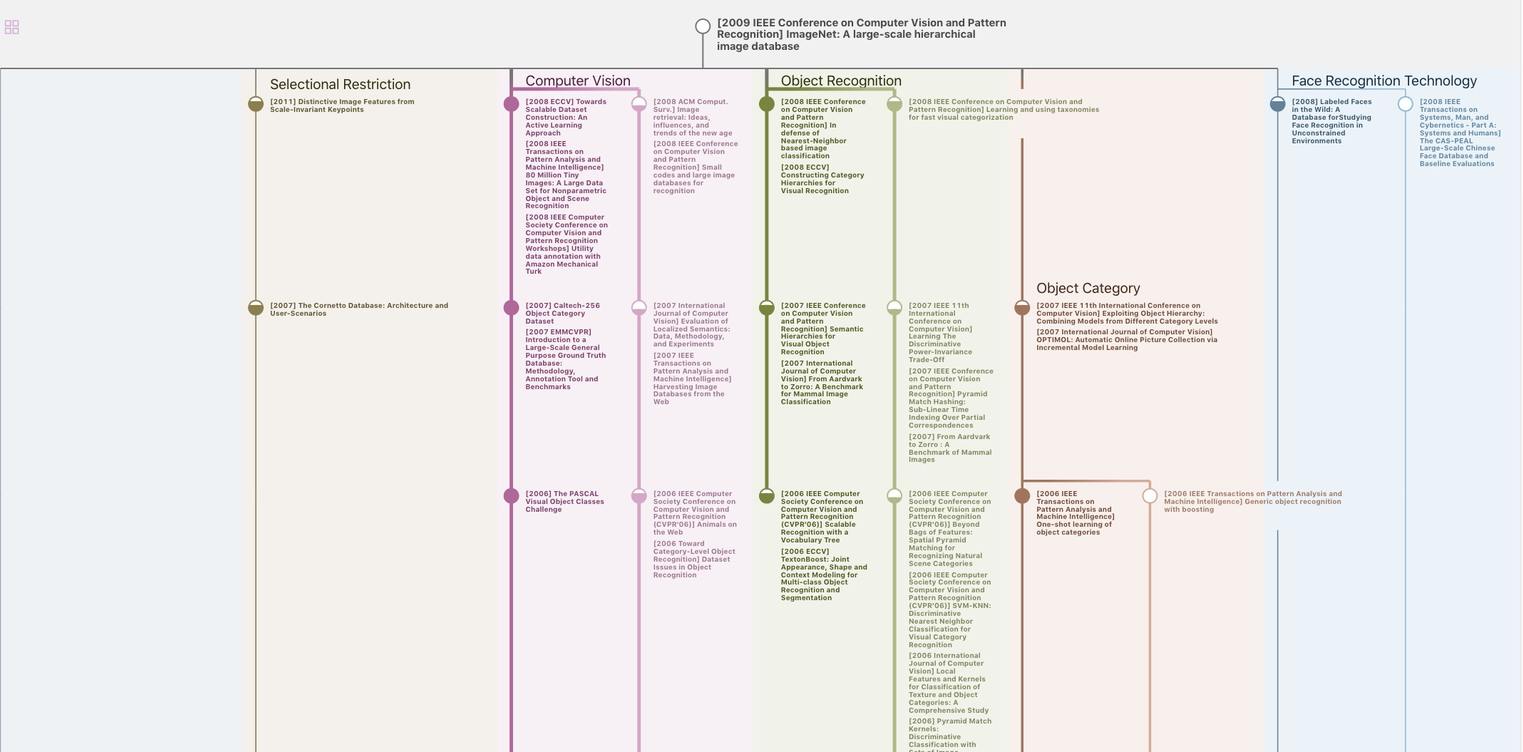
生成溯源树,研究论文发展脉络
Chat Paper
正在生成论文摘要