Kinect Depth Map Inpainting Using A Multi-Scale Deep Convolutional Neural Network
PROCEEDINGS OF 2018 INTERNATIONAL CONFERENCE ON IMAGE AND GRAPHICS PROCESSING (ICIGP 2018)(2018)
摘要
Consumer level RGB-D camera, such as Kinect, is one of the most important devices to get depth data of 3D vision. However, it is quite difficult to get a high-quality depth map which has the same resolution with its corresponding color image and can be aligned perfectly to it. Most previous methods on depth map inpainting focused on denoising and filling up small holes, but they are ineffective to recover large areas of depth missing. Several reasons will cause the large-area depth missing problem, such as the strong specular reflection, the inconsistency of camera tether between color camera and depth camera. In this paper, we present a novel depth map inpainting method for Kinect with a multi-scale deep Convolutional Neural Network (CNN). This method has three stages: depth map pre-processing, multi-scale network training and image optimization. Our method provides a possibility to make a comprehensive refinement for Kinect depth map, including denoising, filling up small holes and inpainting large areas of depth missing. Besides, our recovered depth map can be aligned perfectly to its corresponding color image. And we also evaluate our method on the SUNCG Dataset and real scenes captured by Kinect 2.0. The experiment results show that our method is more capable of inpainting depth map.
更多查看译文
关键词
CNN, inpainting, depth map
AI 理解论文
溯源树
样例
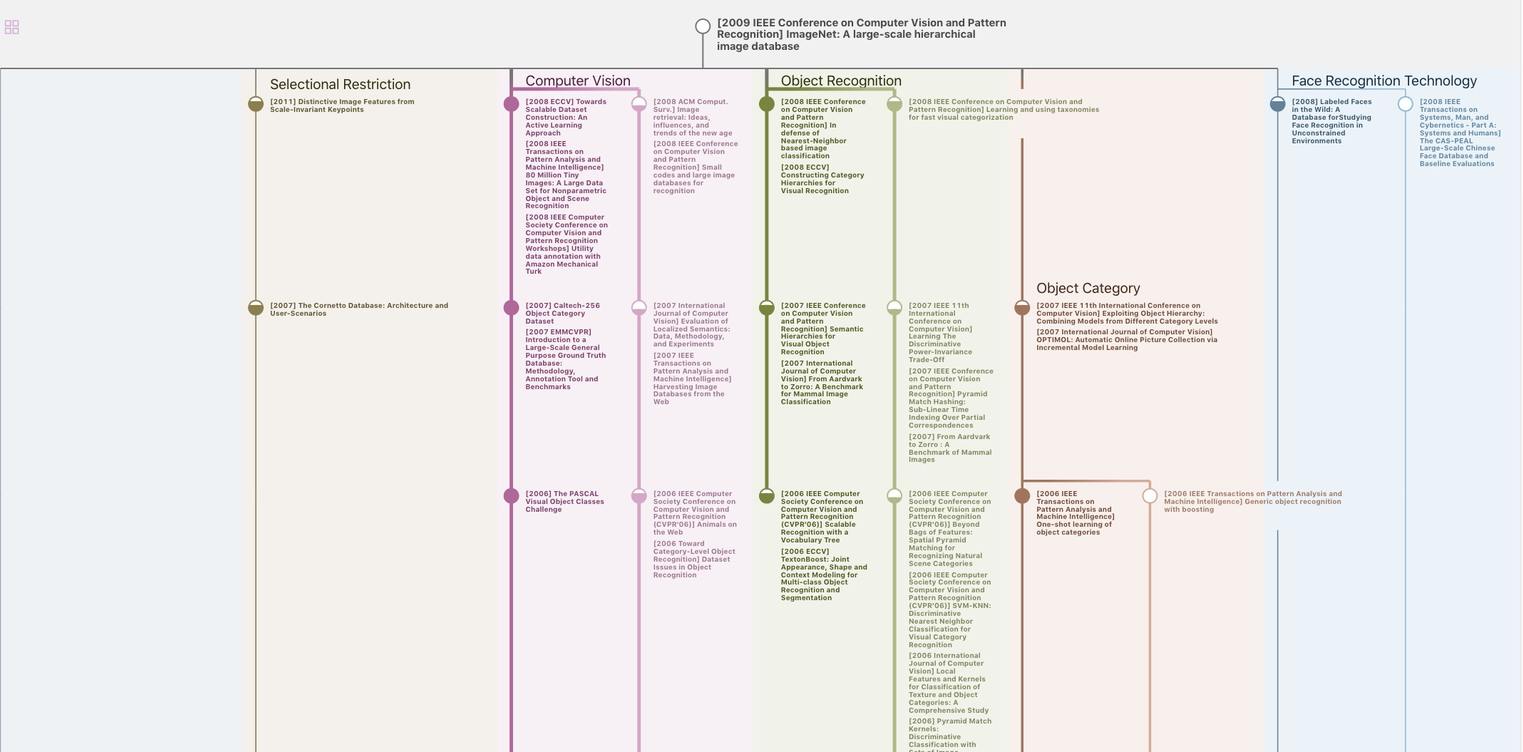
生成溯源树,研究论文发展脉络
Chat Paper
正在生成论文摘要