Exploration of a Graph-based Density-sensitive Metric.
arXiv: Computational Geometry(2018)
摘要
We consider a simple graph-based metric on points in Euclidean space known as the edge-squared metric. This metric is defined by squaring the Euclidean distance between points, and taking the shortest paths on the resulting graph. This metric has been studied before in wireless networks and machine learning, and has the density sensitive property: distances between two points in the same cluster are short, even if their Euclidean distance is long. This property is desirable in machine learning. In this paper, we show that this metric is equal to previously studied geodesic-based metric defined on points in Euclidean space. Previous best work showed that these metrics are $3$-approximations of each other. It was not known or suspected that these metrics were equal. We give fast algorithms to compute sparse spanners of this distance, a problem that can be seen as a generalization of both the Euclidean spanner and the Euclidean MST problem. Spanners of the edge-squared metric are faster to compute and sparser than the best known Euclidean spanners in a variety of settings.
更多查看译文
AI 理解论文
溯源树
样例
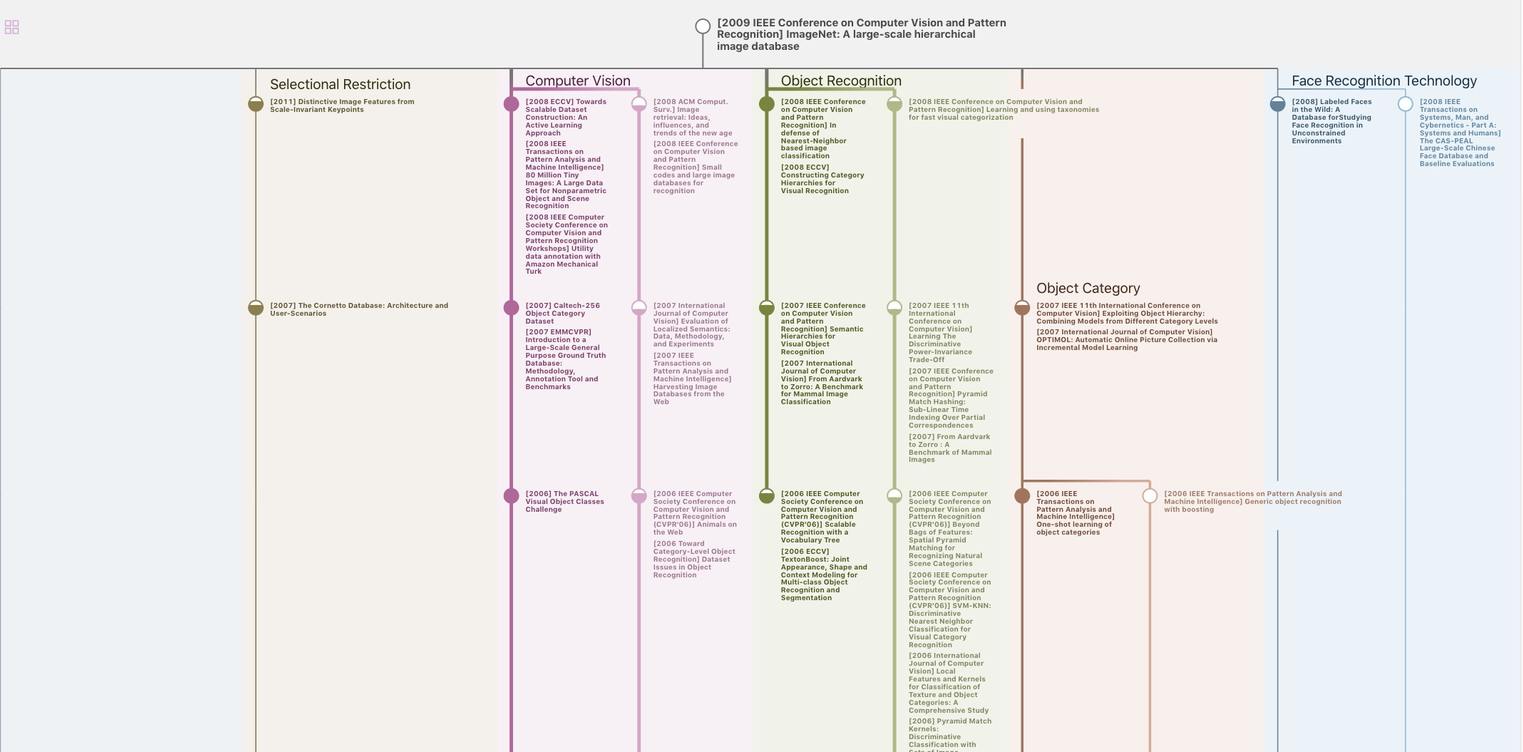
生成溯源树,研究论文发展脉络
Chat Paper
正在生成论文摘要