Kernel partial correlation: a novel approach to capturing conditional independence in graphical models for noisy data
JOURNAL OF APPLIED STATISTICS(2018)
摘要
Graphical models capture the conditional independence structure among random variables via existence of edges among vertices. One way of inferring a graph is to identify zero partial correlation coefficients, which is an effective way of finding conditional independence under a multivariate Gaussian setting. For more general settings, we propose kernel partial correlation which extends partial correlation with a combination of two kernel methods. First, a nonparametric function estimation is employed to remove effects from other variables, and then the dependence between remaining random components is assessed through a nonparametric association measure. The proposed approach is not only flexible but also robust under high levels of noise owing to the robustness of the nonparametric approaches.
更多查看译文
关键词
Conditional independence,graphical model,Hilbert-Schmidt independence criterion,partial correlation coefficient,reproducing kernel Hilbert space,support vector regression
AI 理解论文
溯源树
样例
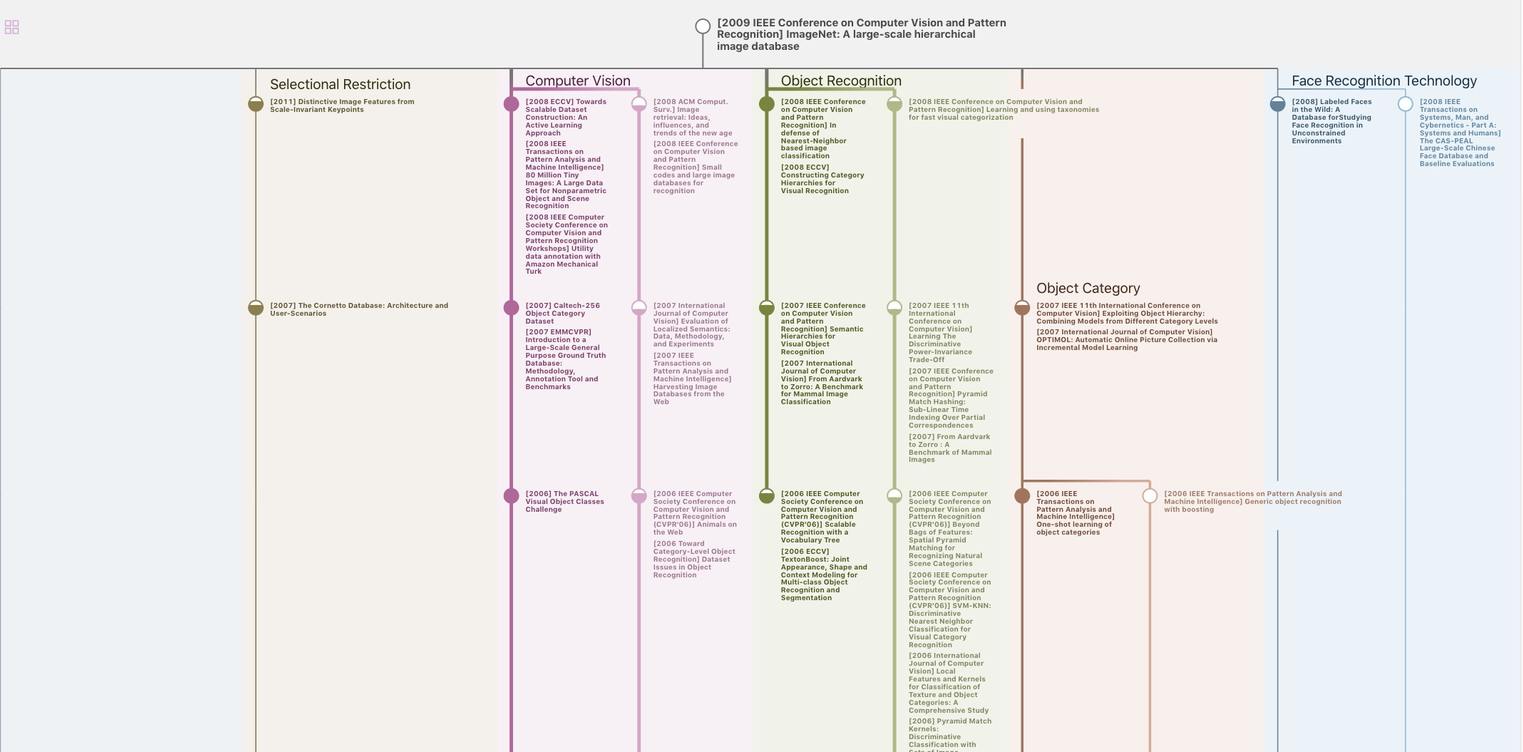
生成溯源树,研究论文发展脉络
Chat Paper
正在生成论文摘要